Jmpnet: Joint Motion Prediction for Learning-Based Video Compression
IEEE International Conference on Acoustics, Speech, and Signal Processing (ICASSP)(2022)
摘要
In recent years, more attention is attracted by learning-based approaches in the field of video compression. Recent methods of this kind normally consist of three major components: intra-frame network, motion prediction network, and residual network, among which the motion prediction part is particularly critical for video compression. Benefiting from the optical flow which enables dense motion prediction, recent methods have shown competitive performance compared with traditional codecs. However, problems such as tail shadow and background distortion in the predicted frame remain unsolved. To tackle these problems, JMPNet is introduced in this paper to provide more accurate motion information by using both optical flow and dynamic local filter as well as an attention map to further fuse these motion information in a smarter way. Experimental results show that the proposed method surpasses state-of-the-art (SOTA) rate-distortion (RD) performance in the most data-sets.
更多查看译文
关键词
deep learning,video compression,joint motion prediction,optical flow,dynamic local filter
AI 理解论文
溯源树
样例
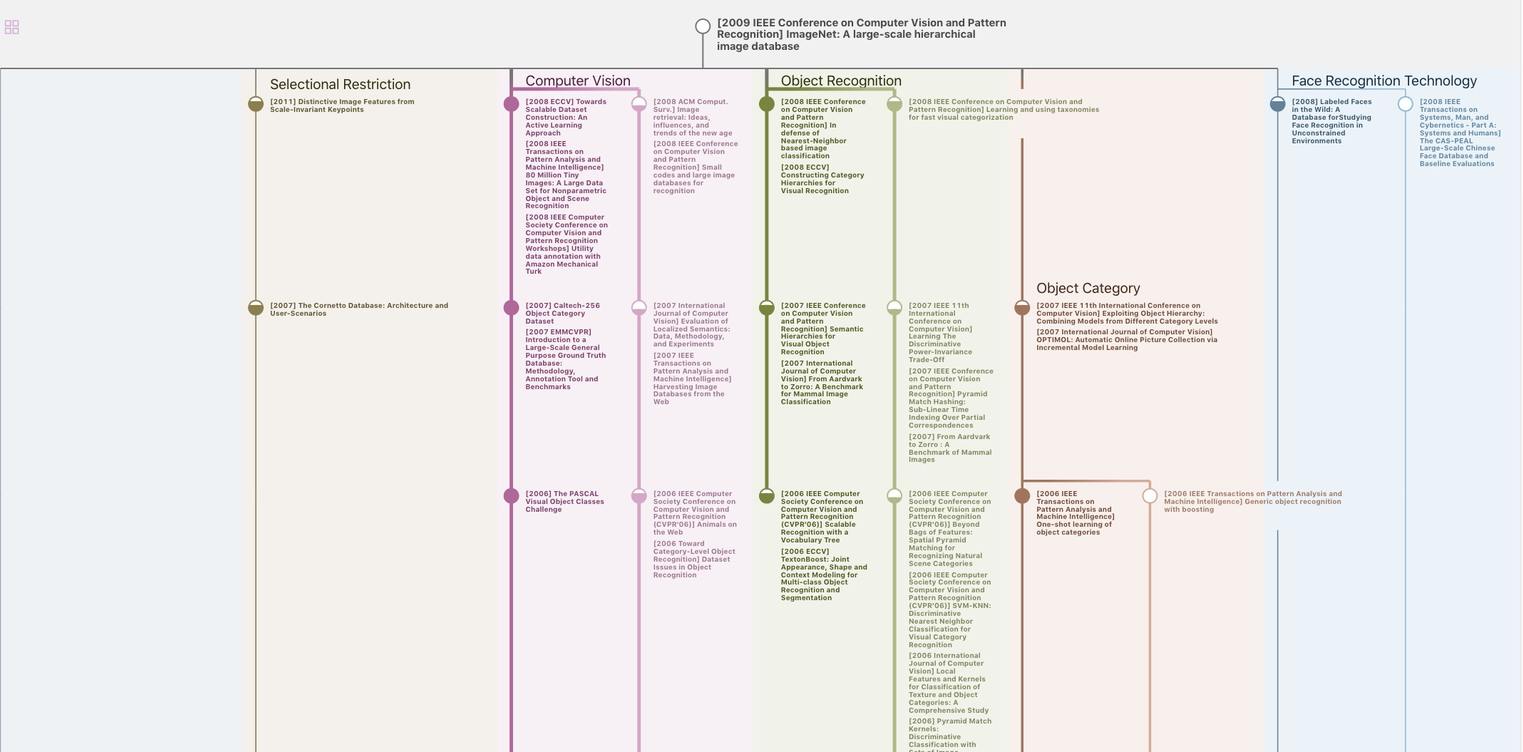
生成溯源树,研究论文发展脉络
Chat Paper
正在生成论文摘要