Multiple Temporal Context Embedding Networks for Unsupervised time Series Anomaly Detection
IEEE International Conference on Acoustics, Speech, and Signal Processing (ICASSP)(2022)
Abstract
Unsupervised anomaly detection for time series signals is challenging, due to the imbalanced distribution of data and the lack of ground-truth labels. Current methods on this topic are mainly based on deep neural networks, which are optimized by heuristic constraints or empirical priors. However, various patterns of anomalous data, especially those lasting for varying periods, are hard to be captured by plain networks. To tackle this problem, we propose a multiple temporal context embedding method. The core of our method is to construct a unified representation of the multiple temporal contexts of data, which is achieved by learning a set of base features to reconstruct the hidden features within existing anomaly detection networks. The proposed method can be implemented as a convenient plug-in module, and be combined with various network architectures, such as autoencoders and graph neural networks. Extensive experiments on multiple datasets demonstrate that the proposed method can boost the performance of baseline networks significantly.
MoreTranslated text
Key words
Anomaly Detection,Unsupervised Learning,Temporal Context,Time Series Signal Processing
AI Read Science
Must-Reading Tree
Example
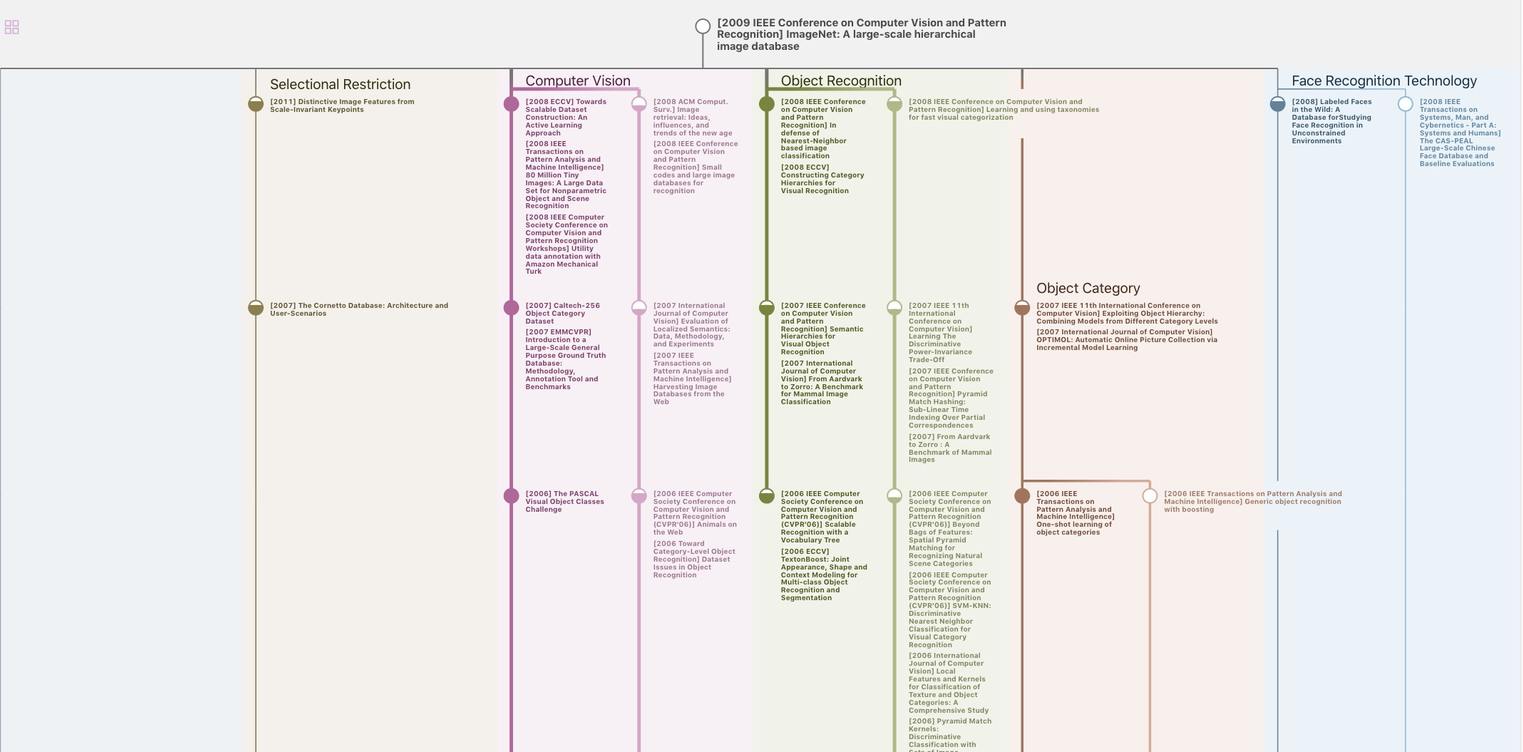
Generate MRT to find the research sequence of this paper
Chat Paper
Summary is being generated by the instructions you defined