Enhancing Contrastive Learning with Temporal Cognizance for Audio-Visual Representation Generation
IEEE International Conference on Acoustics, Speech, and Signal Processing (ICASSP)(2022)
摘要
Audio-visual data allows us to leverage different modalities for downstream tasks. The idea being individual streams can complement each other in the given task, thereby resulting in a model with improved performance. In this work, we present our experimental results on action recognition and video summarization tasks. The proposed modeling approach builds upon the recent advances in contrastive loss based audio-visual representation learning. Temporally cognizant audio-visual discrimination is achieved in a Transformer model by learning with a masked feature reconstruction loss over a fixed time window in addition to learning via contrastive loss. Overall, our results indicate that the addition of temporal information significantly improved the performance of the contrastive loss based framework. We achieve an action classification accuracy of 66.2% versus the next best baseline at 64.7% on the HMDB dataset. For video summarization, we attain an F1 score of 43.5 verses 42.2 on the SumMe dataset.
更多查看译文
关键词
representation learning,action recognition,video summarization,contrastive loss,transformers
AI 理解论文
溯源树
样例
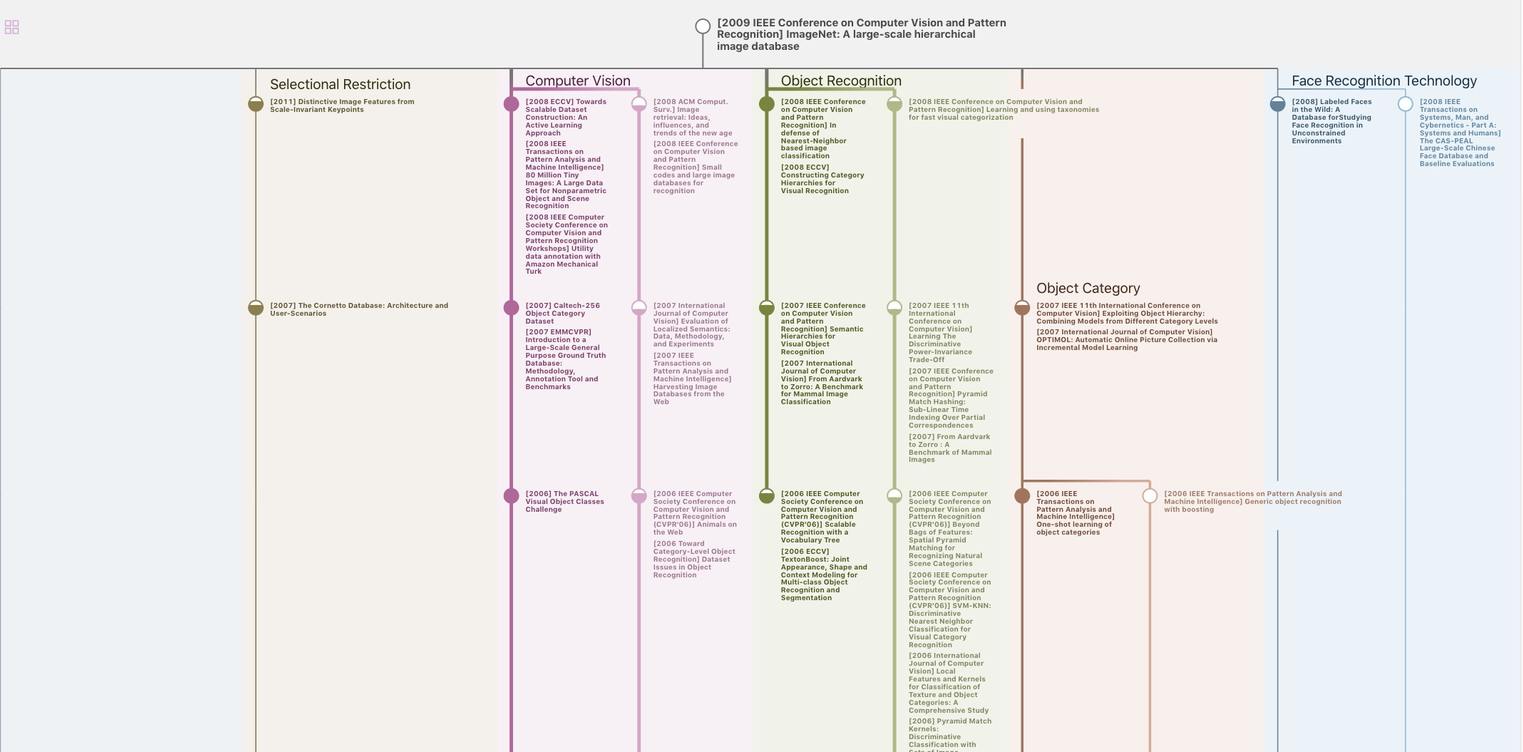
生成溯源树,研究论文发展脉络
Chat Paper
正在生成论文摘要