Graph-Structured Sparse Regularization Via Convex Optimization
IEEE International Conference on Acoustics, Speech, and Signal Processing (ICASSP)(2022)
摘要
In this paper, we present a novel convex method for the graph-structured sparse recovery. While various structured sparsities can be represented as the graph-structured sparsity, graph-structured sparse recovery remains to be a challenging nonconvex problem. To solve this difficulty, we propose a convex penalty function which automatically identifies the relevant subgraph of an underlying graph. We design a graph-structured recovery model using the proposed penalty, and develop its first-order iterative solver which consists only of simple operations such as closed-form proximity operators and difference operator on the graph. Numerical experiments show that the proposed method has better estimation accuracy than the existing convex regularizations using fixed structures.
更多查看译文
关键词
Graph-structured sparsity,convex regularization,proximal splitting method,first-order algorithm
AI 理解论文
溯源树
样例
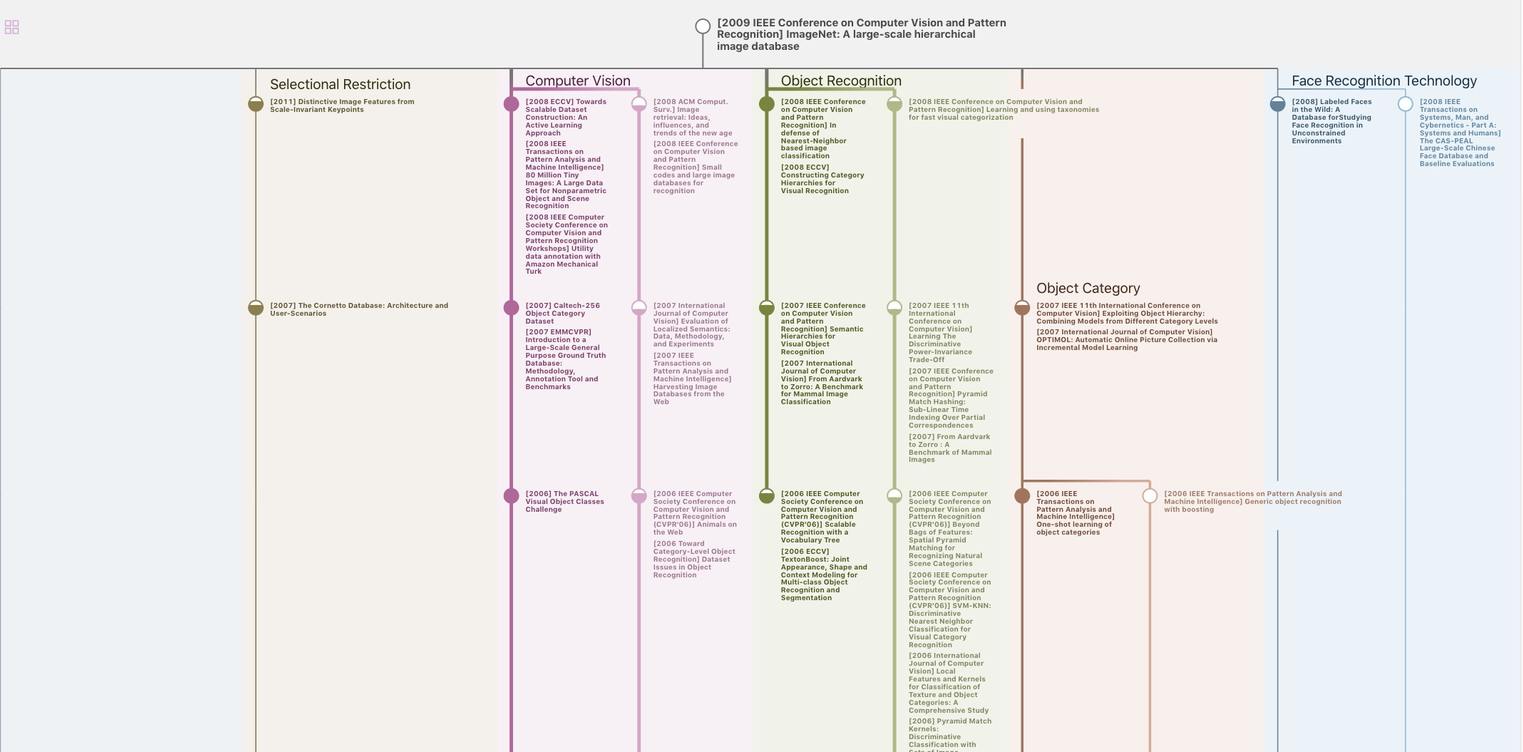
生成溯源树,研究论文发展脉络
Chat Paper
正在生成论文摘要