Combating False Sense of Security: Breaking the Defense of Adversarial Training Via Non-Gradient Adversarial Attack
IEEE International Conference on Acoustics, Speech, and Signal Processing (ICASSP)(2022)
摘要
Adversarial training is believed to be the most robust and effective defense method against adversarial attacks. Gradientbased adversarial attack methods are generally adopted to evaluate the effectiveness of adversarial training. However, in this paper, by diving into the existing adversarial attack literature, we find that adversarial examples generated by these attack methods tend to be less imperceptible, which may lead to an inaccurate estimation for the effectiveness of the adversarial training. The existing adversarial attacks mostly adopt gradient-based optimization methods and such optimization methods have difficulties in searching the most effective adversarial examples (i.e., the global extreme points). On the contrast, in this work, we propose a novel Non-Gradient Attack (NGA) to overcome the above-mentioned problem. Extensive experiments show that NGA significantly outperforms the state-of-the-art adversarial attacks on Attack Success Rate (ASR) by 2% similar to 7%.
更多查看译文
关键词
adversarial attack,adversarial training,non-gradient attack
AI 理解论文
溯源树
样例
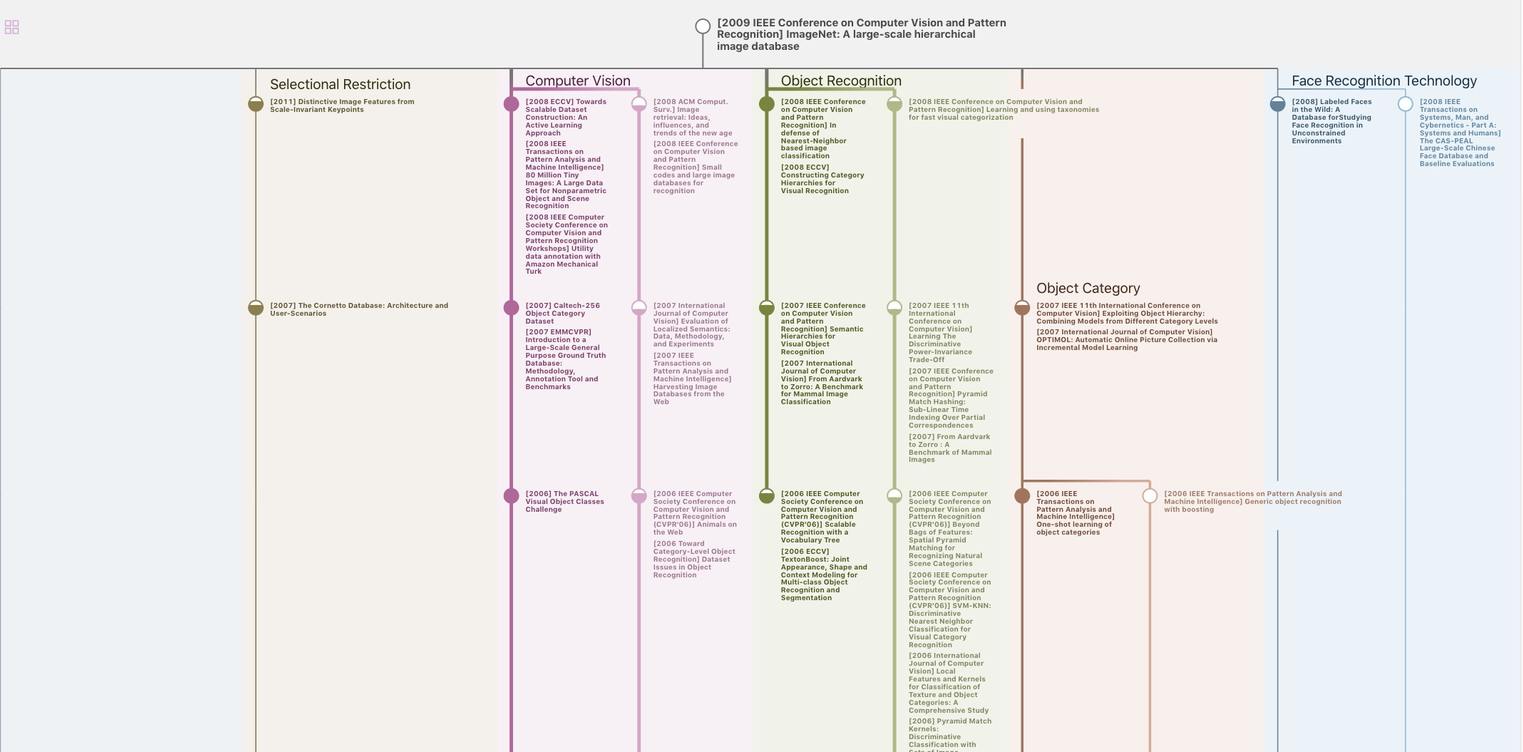
生成溯源树,研究论文发展脉络
Chat Paper
正在生成论文摘要