Duration Modeling of Neural TTS for Automatic Dubbing
IEEE International Conference on Acoustics, Speech, and Signal Processing (ICASSP)(2022)
Abstract
Automatic dubbing (AD) addresses the problem of translating speech in a video with speech in another language while preserving the viewer experience. A most important requirement of AD is isochrony, i.e. dubbed speech has to closely match the timing of speech and pauses of the original audio. In our automatic dubbing system, isochrony is modeled by controlling the verbosity of machine translation; inserting pauses in the translations, a.k.a. prosodic alignment; and controlling the duration of text-to-speech (TTS) utterances. The latter two steps heavily rely on speech duration information, either to predict or control TTS duration. So far, duration prediction was based on a proxy method while duration control on linear warping of the TTS speech spectrogram. In this study, we propose novel duration models for neural TTS that can be leveraged both to predict and control TTS duration. Experimental results show that compared to previous work, the new models improve or match the performance of prosodic alignment and significantly enhance neural TTS speech quality for both slow and fast speaking rates.
MoreTranslated text
Key words
speech translation,text-to-speech,automatic dubbing,duration modelling
AI Read Science
Must-Reading Tree
Example
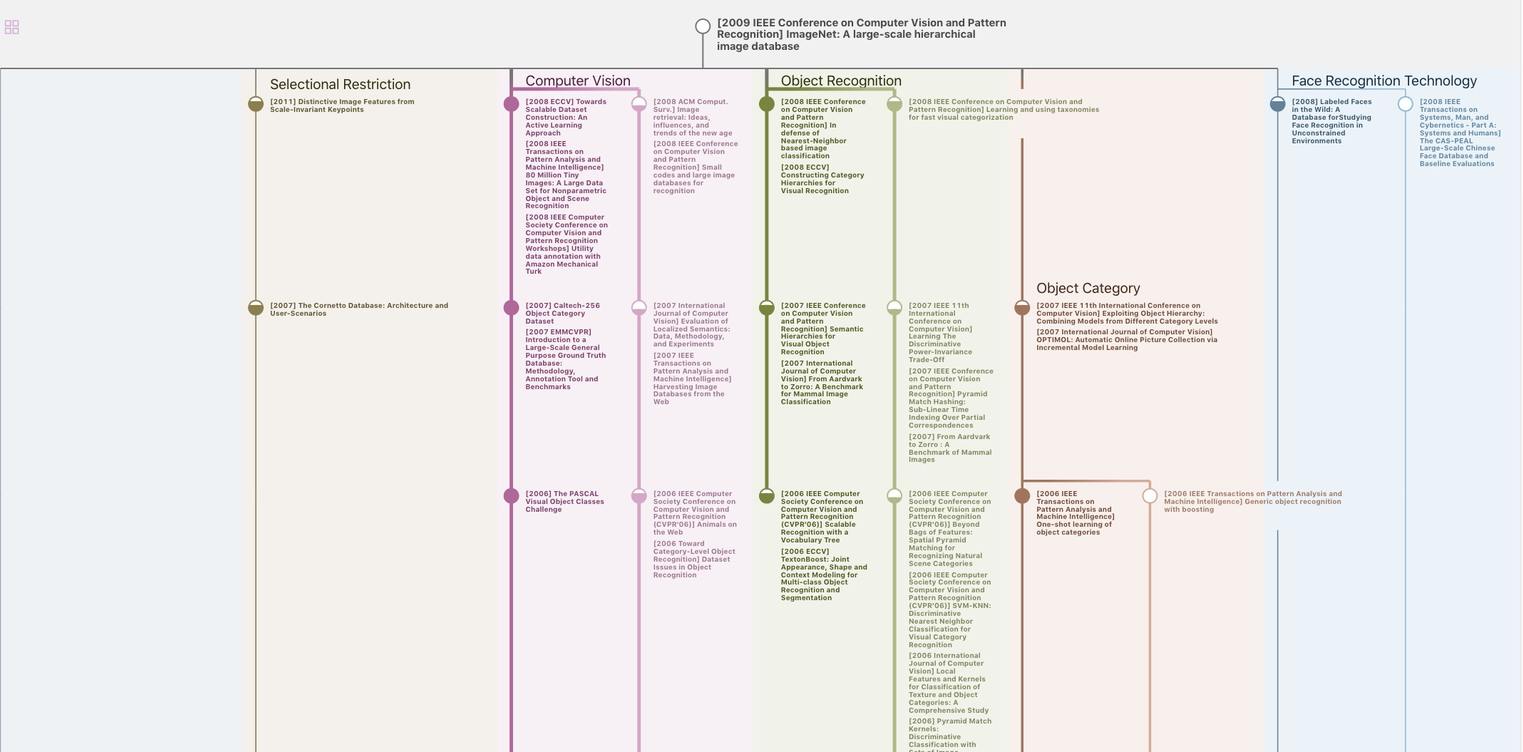
Generate MRT to find the research sequence of this paper
Chat Paper
Summary is being generated by the instructions you defined