Enhancing Class Understanding Via Prompt-Tuning For Zero-Shot Text Classification
IEEE International Conference on Acoustics, Speech, and Signal Processing (ICASSP)(2022)
摘要
Zero-shot text classification (ZSTC) poses a big challenge due to the lack of labeled data for unseen classes during training. Most studies focus on transferring knowledge from seen classes to unseen classes, which have achieved good performance in most cases. Whereas, it is difficult to transfer knowledge when the classes have semantic gaps or low similarities. In this paper, we propose a prompt-based method, which enhances semantic understanding for each class and learns the matching between texts and classes for better ZSTC. Specifically, we first generate discriminative words for class description with prompt inserting (PIN). Then, a prompt matching (POM) model is learned to determine whether the text can well match the class description. Experiments on three benchmark datasets show the great advantages of our proposed method. In particular, we achieve the state-of-the-art performance on the unseen classes, while maintaining comparable strength with the existing ZSTC approaches regarding to the seen classes.
更多查看译文
关键词
Zero-shot Text Classification,Prompt Tuning,Semantics Enhancing
AI 理解论文
溯源树
样例
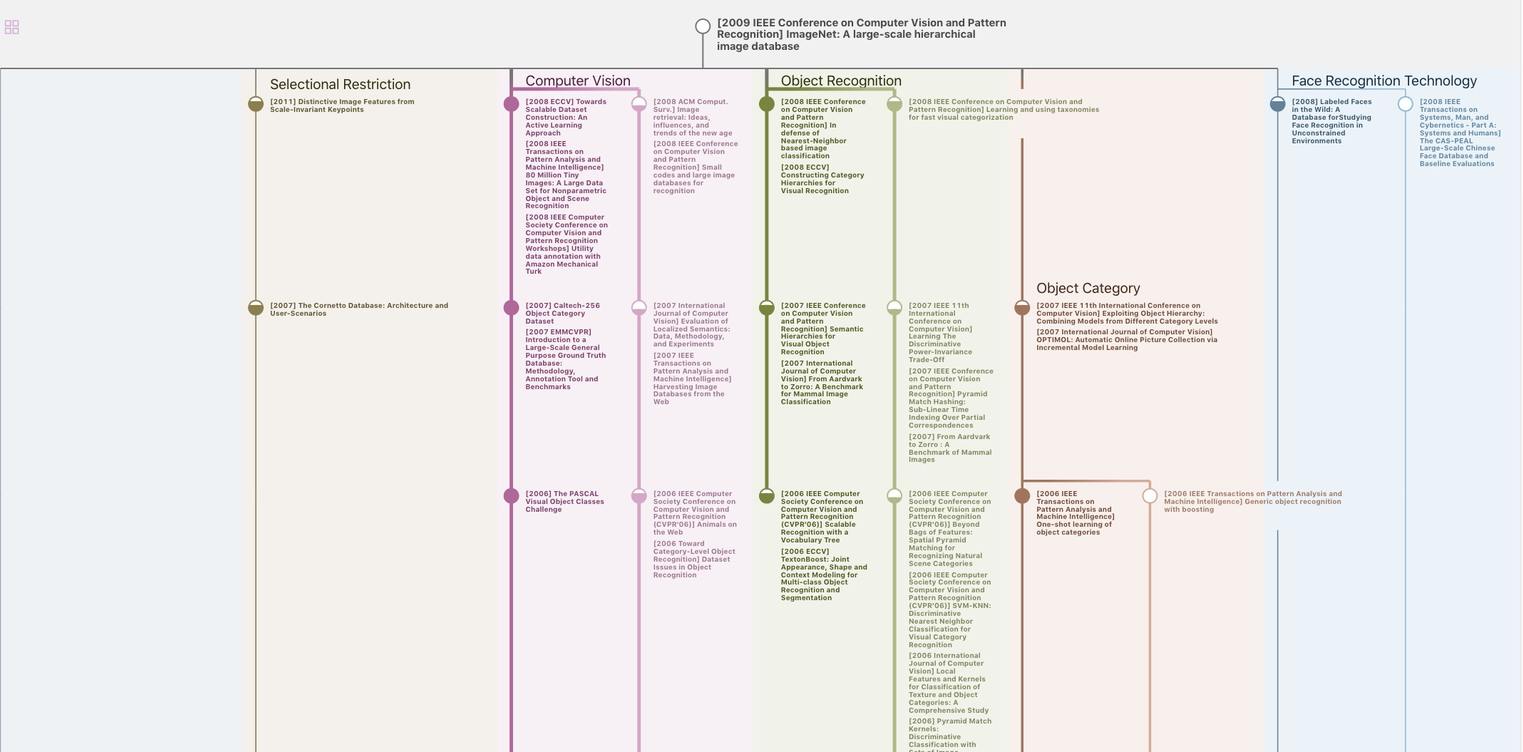
生成溯源树,研究论文发展脉络
Chat Paper
正在生成论文摘要