Unsupervised Hierarchical Translation-Based Model for Multi-Modal Medical Image Registration
IEEE International Conference on Acoustics, Speech, and Signal Processing (ICASSP)(2022)
Abstract
Deformable registration of multi-modal medical images is a challenging task in medical image processing due to the differences in both appearance and structure. We propose an unsupervised hierarchical translation-based model to perform a coarse to fine registration of multi-modal medical images. The proposed model consists of three parts: a coarse registration network, a modal translation network and a fine registration network. First, the coarse registration network learns to obtain the coarse deformation field, which is applied as structure-preserving information to generate a translated image by the modal translation network. Then, the translated image as enhancing information combined with the original images are used to derive a fine deformation field in the fine registration network. Furthermore, the final deformation field is composed from the coarse and the fine deformation fields. In this way, the proposed model can learn high accurate deformation field to implement multi-modal medical image registration. Experiments on two multi-modal brain image datasets demonstrate the effectiveness of this model.
MoreTranslated text
AI Read Science
Must-Reading Tree
Example
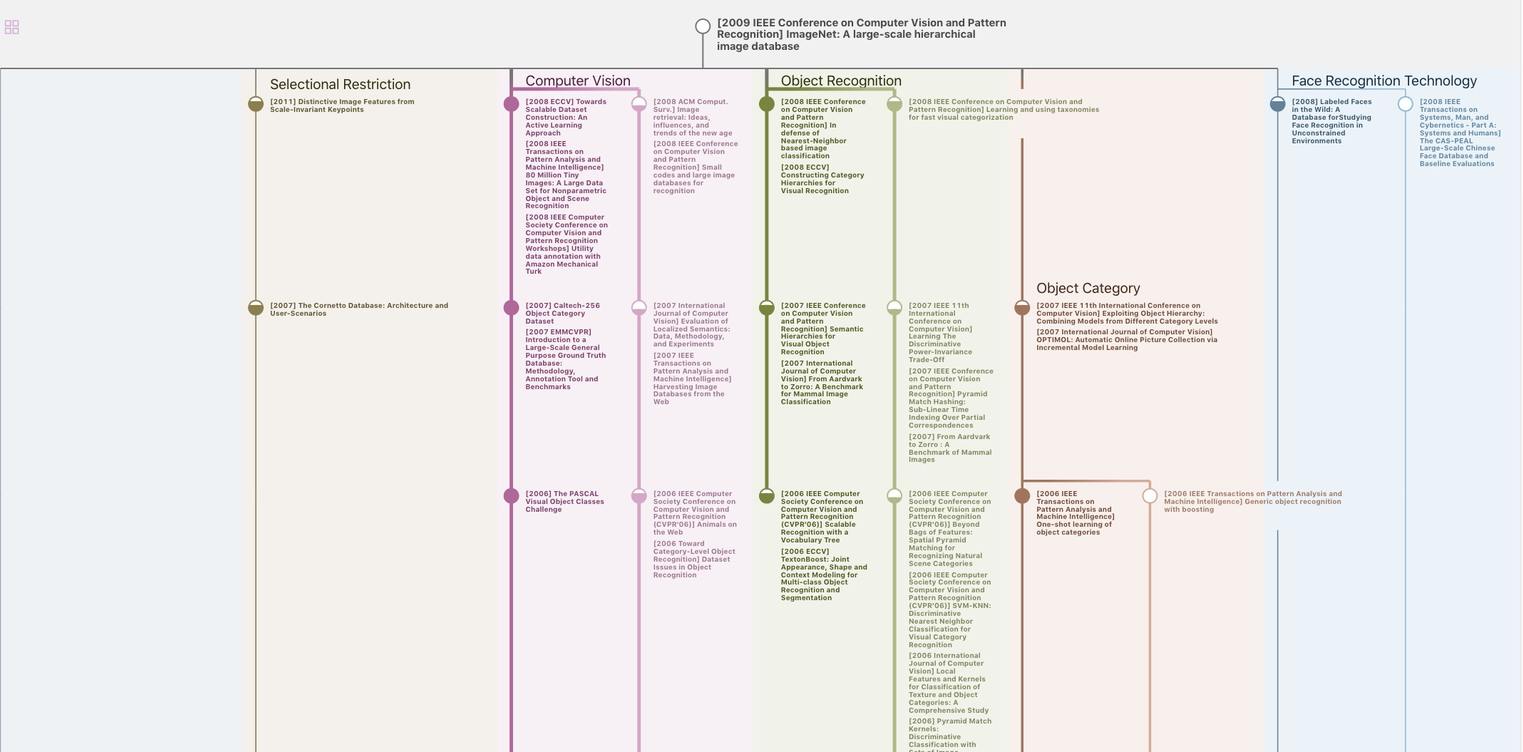
Generate MRT to find the research sequence of this paper
Chat Paper
Summary is being generated by the instructions you defined