Accurate Instance Segmentation Via Collaborative Learning
IEEE International Conference on Acoustics, Speech, and Signal Processing (ICASSP)(2022)
摘要
We propose an instance segmentation model, named CoMask, that effectively alleviates the scale variation issue and addresses the precise localization. Specifically, we develop a multi-scale feature extraction module (MSFEM) to exploit multi-scale spatial cues. Besides, the channel attention mechanism is also adopted to further enhance the discriminating ability. Equipped with MSFEMs, multiscale and multi-level features can be extracted to better characterize objects of various sizes and provide affluent high-level semantic information. For precise localization, we propose a collaborative learning framework to compute coarse masks and regresses position-sensitive dense offsets. The foreground confidence of each position is then assigned as the weight of the corresponding bounding box to calculate a weighted average. Thus, we can mitigate interference of background regions. After obtaining the final regressed bounding boxes, finer foreground masks can be calculated. We conduct experiments on MS COCO dataset. Experimental results validate that CoMask is competitive compared with state-of-the-art models.
更多查看译文
关键词
Collaborative learning,deep learning,instance segmentation,multi-scale feature extraction
AI 理解论文
溯源树
样例
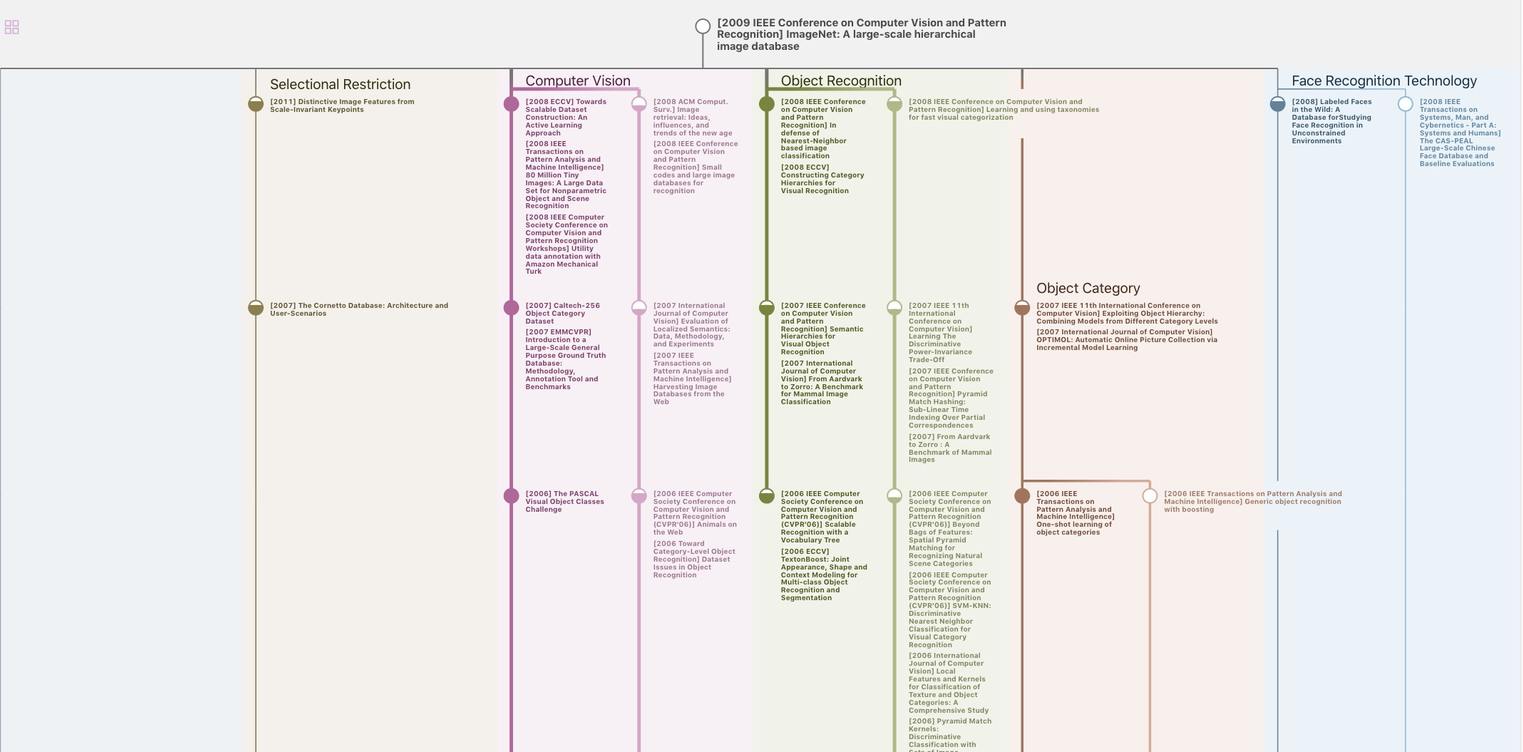
生成溯源树,研究论文发展脉络
Chat Paper
正在生成论文摘要