Melon: breaking the memory wall for resource-efficient on-device machine learning
Mobile Systems, Applications, and Services(2022)
摘要
BSTRACTOn-device learning is a promising technique for emerging privacy-preserving machine learning paradigms. However, through quantitative experiments, we find that commodity mobile devices cannot well support state-of-the-art DNN training with a large enough batch size, due to the limited local memory capacity. To fill the gap, we propose Melon, a memory-friendly on-device learning framework that enables the training tasks with large batch size beyond the physical memory capacity. Melon judiciously retrofits existing memory saving techniques to fit into resource-constrained mobile devices, i.e., recomputation and micro-batch. Melon further incorporates novel techniques to deal with the high memory fragmentation and memory adaptation. We implement and evaluate Melon with various typical DNN models on commodity mobile devices. The results show that Melon can achieve up to 4.33× larger batch size under the same memory budget. Given the same batch size, Melon achieves 1.89× on average (up to 4.01×) higher training throughput, and saves up to 49.43% energy compared to competitive alternatives. Furthermore, Melon reduces 78.59% computation on average in terms of memory budget adaptation.
更多查看译文
AI 理解论文
溯源树
样例
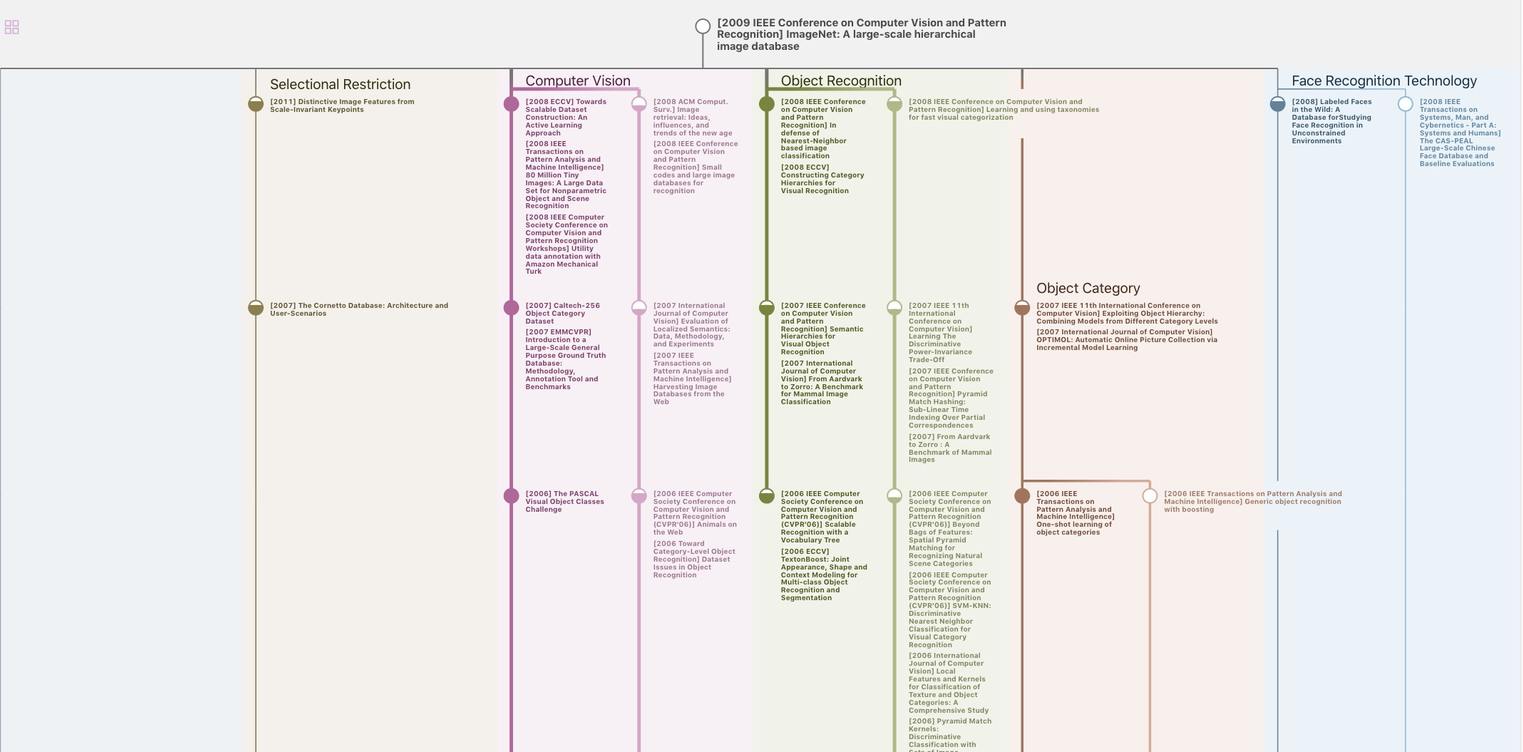
生成溯源树,研究论文发展脉络
Chat Paper
正在生成论文摘要