PDAS: Probability-Driven Adaptive Streaming for Short Video
International Multimedia Conference(2022)
摘要
ABSTRACTTo improve Quality of Experience (QoE) for short video applications, most commercial companies adopt preloading and adaptive streaming technologies concurrently. Though preloading can reduce rebuffering, it may greatly waste bandwidth if the downloaded video chunks are not played. Also, each short video's downloading competes against others, which makes the existing adaptive streaming technologies fail to optimize the QoE for all videos. In this paper, we propose PDAS, a Probability-Driven Adaptive Streaming framework, to minimize the bandwidth waste while guaranteeing QoE simultaneously. We formulate PDAS into an optimization problem, where a probabilistic model is designed to describe the swiping events. Then, the maximum preload size is controlled by the proposed probability-driven max-buffer model, which reduces the bandwidth waste by proactively sleeping. At last, the optimization problem is solved by jointly deciding the preload order and preload bitrate. Extensive experimental results demonstrate that PDAS achieves almost 22.34% gains on QoE and 22.80% reductions on bandwidth usage against the existing methods. As for online evaluation, PDAS ranks first in the ACM MM 2022 Grand Challenge: Short Video Streaming.
更多查看译文
AI 理解论文
溯源树
样例
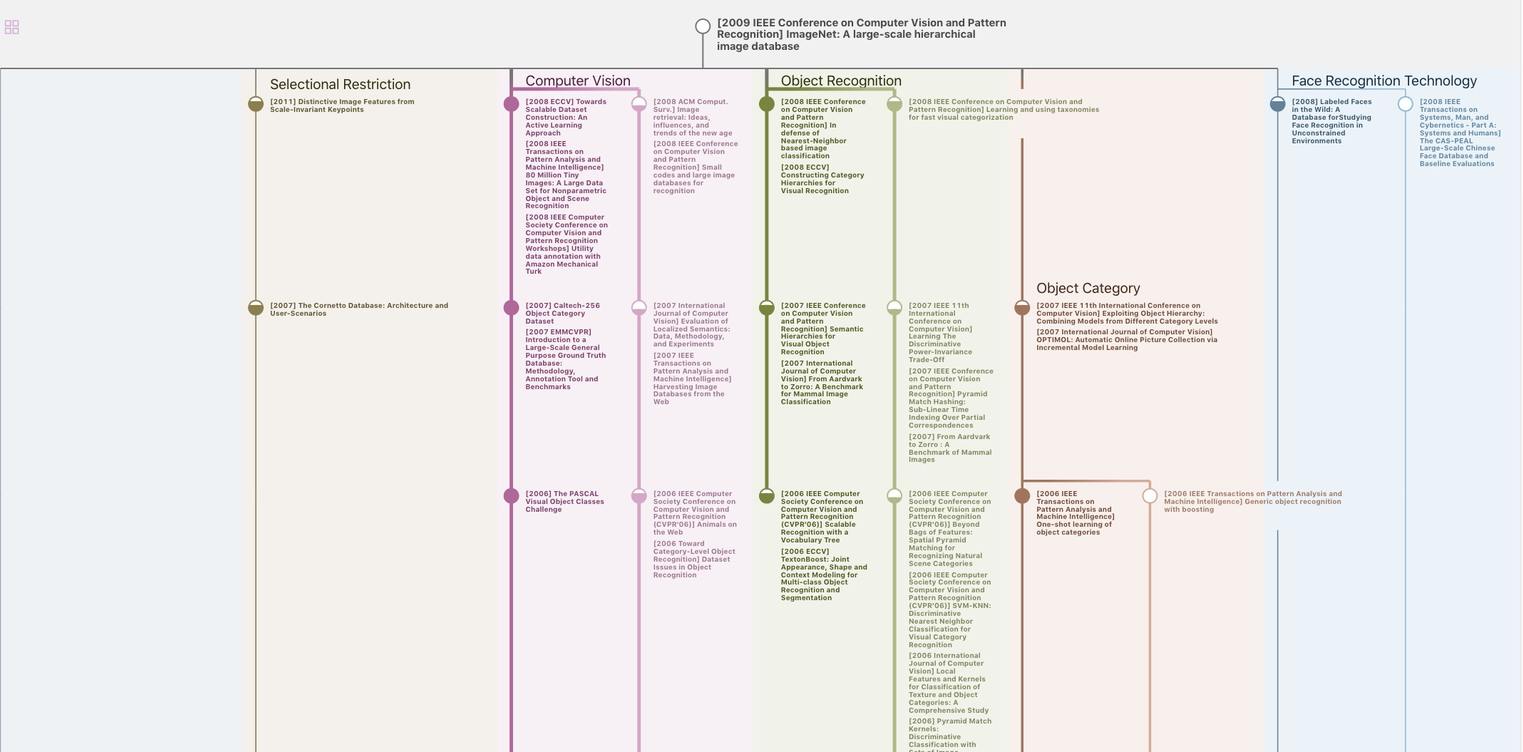
生成溯源树,研究论文发展脉络
Chat Paper
正在生成论文摘要