Learning from Different text-image Pairs: A Relation-enhanced Graph Convolutional Network for Multimodal NER
International Multimedia Conference(2022)
摘要
ABSTRACTMultimodal Named Entity Recognition (MNER) aims to locate and classify named entities mentioned in a (text, image) pair. However, dominant work independently models the internal matching relations in a pair of image and text, ignoring the external matching relations between different (text, image) pairs inside the dataset, though such relations are crucial for alleviating image noise in MNER task. In this paper, we primarily explore two kinds of external matching relations between different (text, image) pairs, i.e., inter-modal relations and intra-modal relations. On the basis, we propose a Relation-enhanced Graph Convolutional Network (R-GCN) for the MNER task. Specifically, we first construct an inter-modal relation graph and an intra-modal relation graph to gather the image information most relevant to the current text and image from the dataset, respectively. And then, multimodal interaction and fusion are leveraged to predict the NER label sequences. Extensive experimental results show that our model consistently outperforms state-of-the-art works on two public datasets. Our code and datasets are available at https://github.com/1429904852/R-GCN.
更多查看译文
关键词
graph convolutional network,learning,text-image,relation-enhanced
AI 理解论文
溯源树
样例
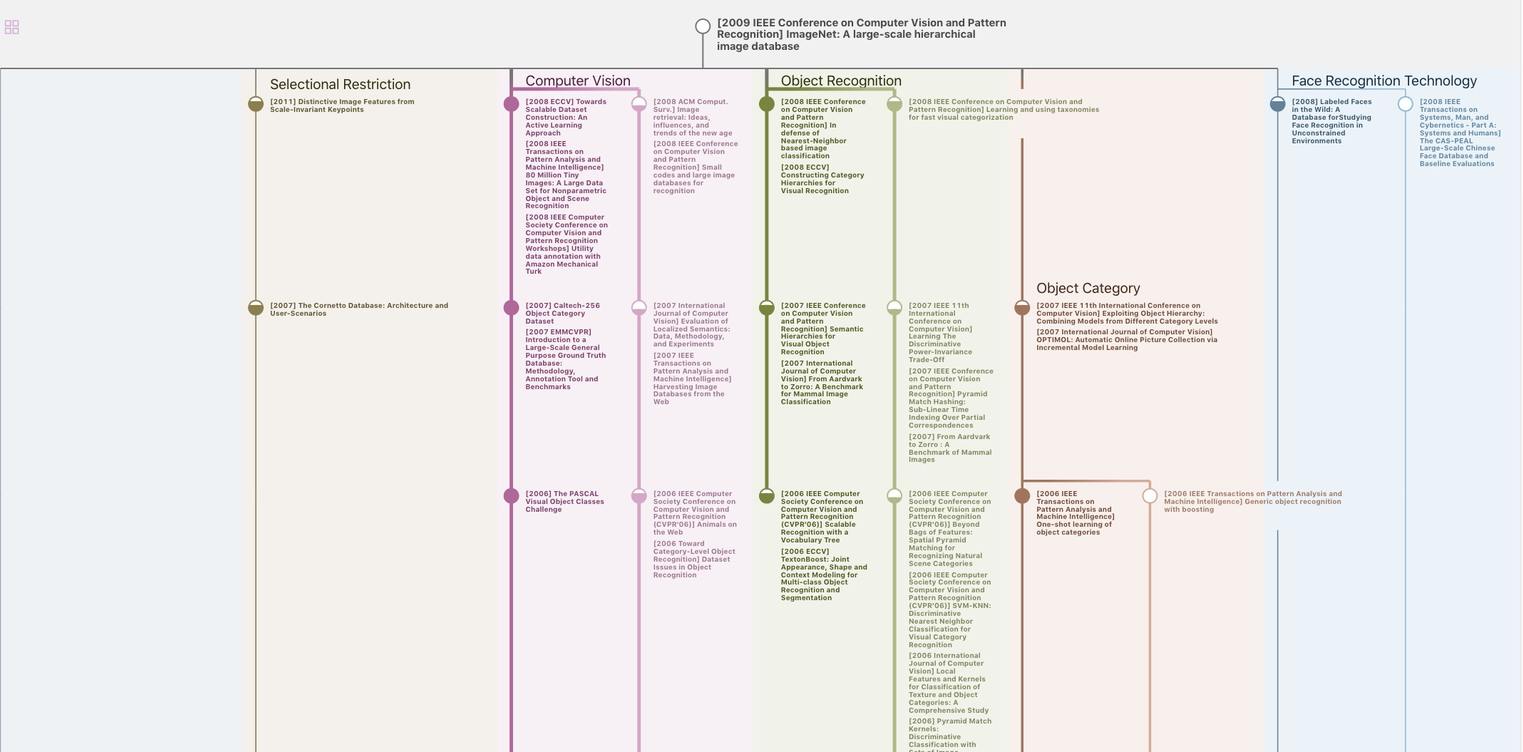
生成溯源树,研究论文发展脉络
Chat Paper
正在生成论文摘要