Relation-enhanced Negative Sampling for Multimodal Knowledge Graph Completion
International Multimedia Conference(2022)
摘要
ABSTRACTKnowledge Graph Completion (KGC), aiming to infer the missing part of Knowledge Graphs (KGs), has long been treated as a crucial task to support downstream applications of KGs, especially for the multimodal KGs (MKGs) which suffer the incomplete relations due to the insufficient accumulation of multimodal corpus. Though a few research attentions have been paid to the completion task of MKGs, there is still a lack of specially designed negative sampling strategies tailored to MKGs. Meanwhile, though effective negative sampling strategies have been widely regarded as a crucial solution for KGC to alleviate the vanishing gradient problem, we realize that, there is a unique challenge for negative sampling in MKGs about how to model the effect of KG relations during learning the complementary semantics among multiple modalities as an extra context. In this case, traditional negative sampling techniques which only consider the structural knowledge may fail to deal with the multimodal KGC task. To that end, in this paper, we propose a MultiModal Relation-enhanced Negative Sampling (MMRNS) framework for multimodal KGC task. Especially, we design a novel knowledge-guided cross-modal attention (KCA) mechanism, which provides bi-directional attention for visual & textual features via integrating relation embedding. Then, an effective contrastive semantic sampler is devised after consolidating the KCA mechanism with contrastive learning. In this way, a more similar representation of semantic features between positive samples, as well as a more diverse representation between negative samples under different relations could be learned. Afterwards, a masked gumbel-softmax optimization mechanism is utilized for solving the non-differentiability of sampling process, which provides effective parameter optimization compared with traditional sample strategies. Extensive experiments on three multimodal KGs demonstrate that our MMRNS framework could significantly outperform the state-of-the-art baseline methods, which validates the effectiveness of relation guides in multimodal KGC task.
更多查看译文
关键词
negative sampling,knowledge,relation-enhanced
AI 理解论文
溯源树
样例
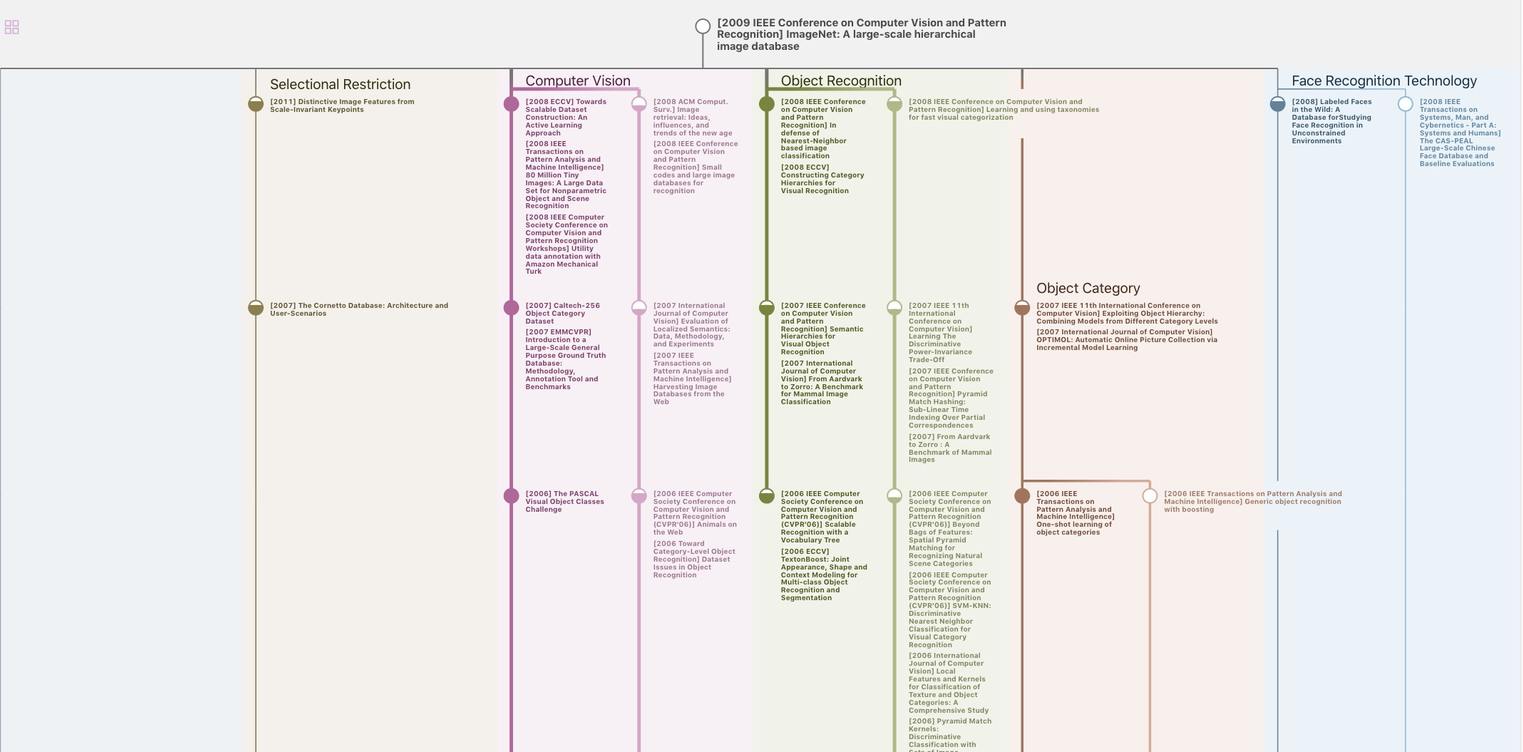
生成溯源树,研究论文发展脉络
Chat Paper
正在生成论文摘要