Towards Causality Inference for Very Important Person Localization
International Multimedia Conference(2022)
Abstract
ABSTRACTVery Important Person Localization (VIPLoc) aims at detecting certain individuals in a given image, who are more attractive than others in the image. Existing uncontrolled VIPLoc benchmark assumes that the image has one single VIP, which is not suitable for actual application scenarios when multiple VIPs or no VIPs appear in the image. In this paper, we re-built a complex uncontrolled conditions (CUC) dataset to make the VIPLoc closer to the actual situation, containing no, single, and multiple VIPs. Existing methods use the hand-designed and deep learning strategies to extract the features of persons and analyze the differences between VIPs and other persons from the perspective of statistics. They are not explainable as to why the VIP located this output for that input. Thus, there exist the severe performance degradation when we use these models in real-world VIPLoc. Specifically, we establish a causal inference framework that unpacks the causes of previous methods and derives a new principled solution for VIPLoc. It treats the scene as confounding factor, allowing the ever-elusive confounding effects to be eliminated and the essential determinants to be uncovered. Through extensive experiments, our method outperforms the state-of-the-art methods on public VIPLoc datasets and the re-built CUC dataset.
MoreTranslated text
Key words
important person localization,causality inference
AI Read Science
Must-Reading Tree
Example
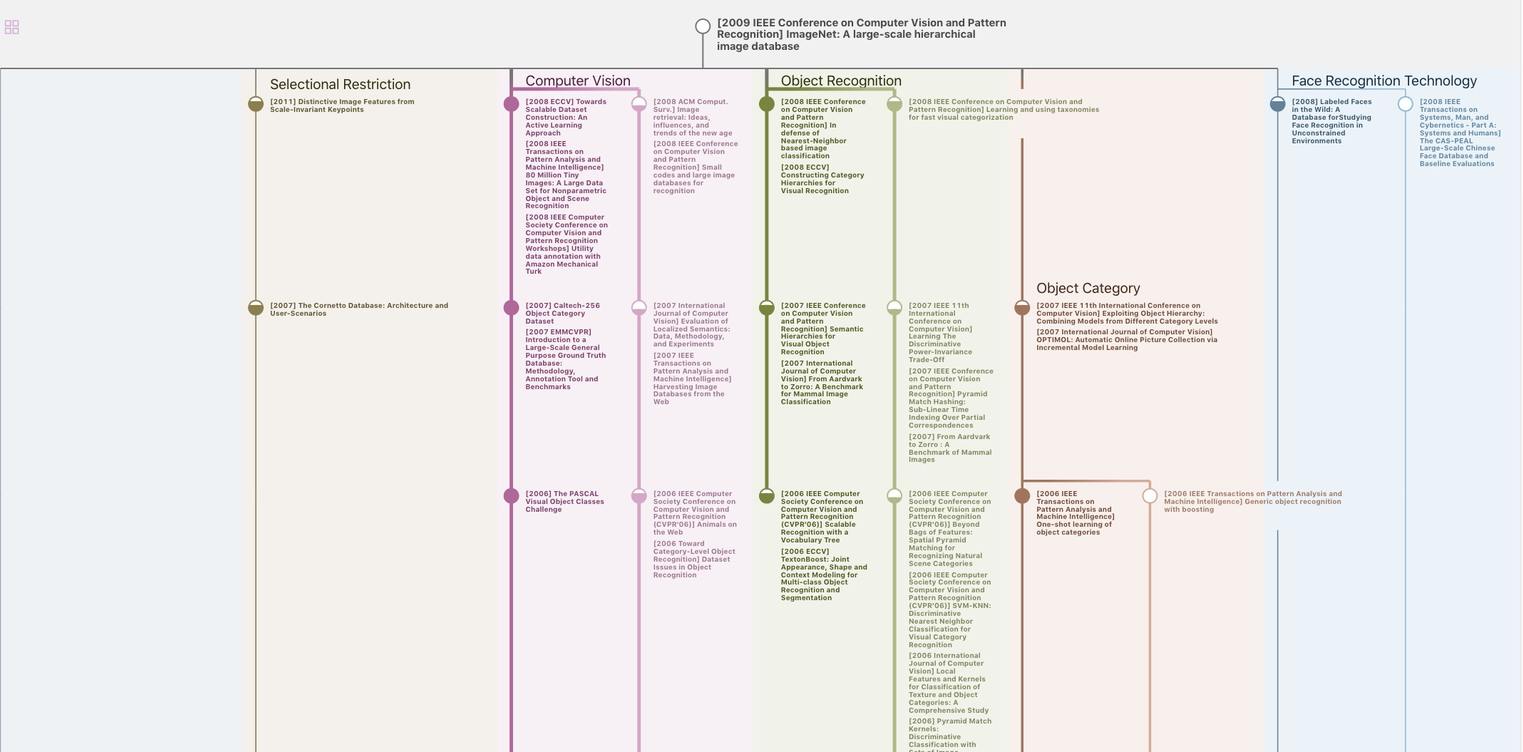
Generate MRT to find the research sequence of this paper
Chat Paper
Summary is being generated by the instructions you defined