Balanced Gradient Penalty Improves Deep Long-Tailed Learning
International Multimedia Conference(2022)
摘要
ABSTRACTIn recent years, deep learning has achieved a great success in various image recognition tasks. However, the long-tailed setting over a semantic class plays a leading role in real-world applications. Common methods focus on optimization on balanced distribution or naive models. Few works explore long-tailed learning from a deep learning-based generalization perspective. The loss landscape on long-tailed learning is first investigated in this work. Empirical results show that sharpness-aware optimizers work not well on long-tailed learning. Because they do not take class priors into consideration, and they fail to improve performance of few-shot classes. To better guide the network and explicitly alleviate sharpness without extra computational burden, we develop a universal Balanced Gradient Penalty (BGP) method. Surprisingly, our BGP method does not need the detailed class priors and preserves privacy. Our new algorithm BGP, as a regularization loss, can achieve the state-of-the-art results on various image datasets (i.e., CIFAR-LT, ImageNet-LT and iNaturalist-2018) in the settings of different imbalance ratios.
更多查看译文
关键词
balanced gradient penalty,learning,deep,long-tailed
AI 理解论文
溯源树
样例
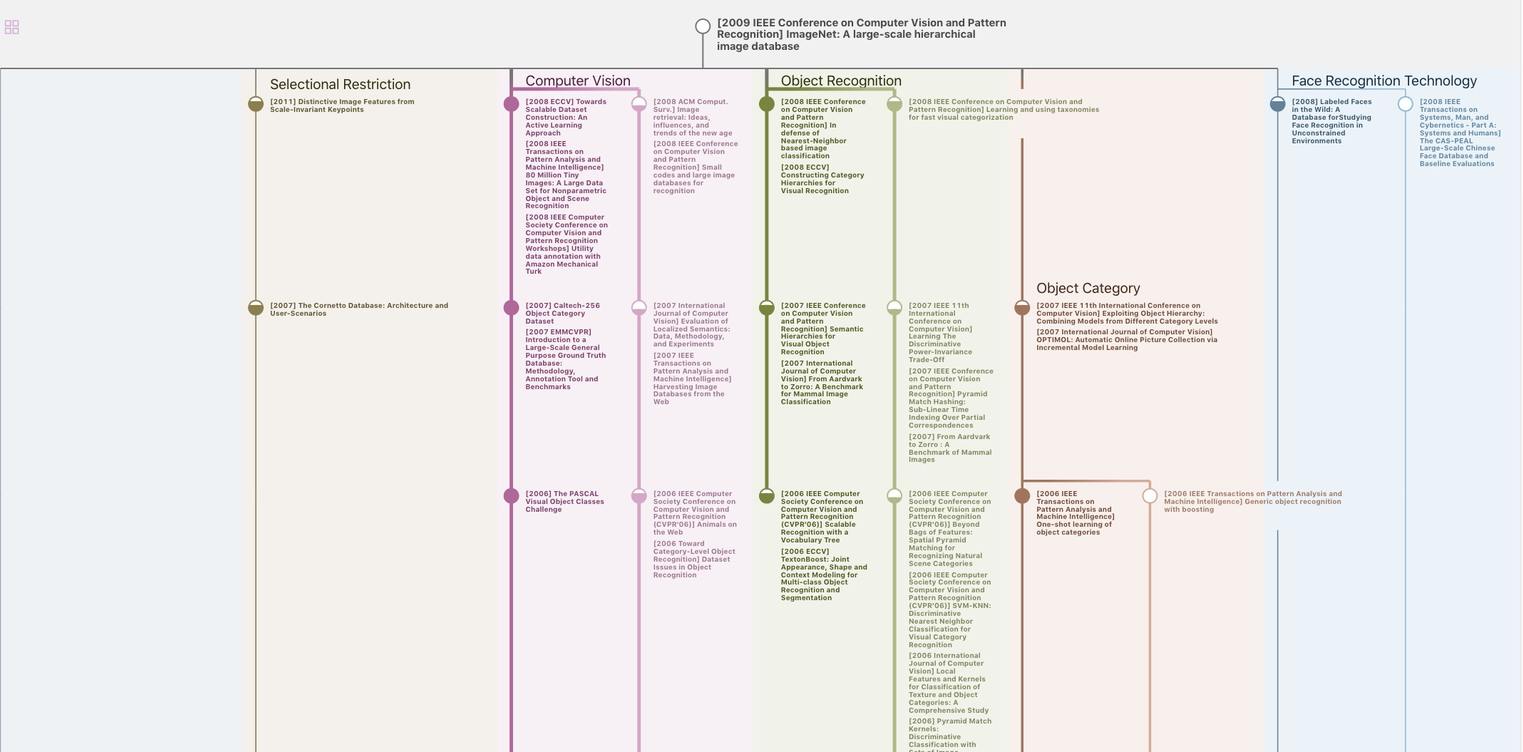
生成溯源树,研究论文发展脉络
Chat Paper
正在生成论文摘要