Guess-It-Generator: Generating in a Lewis Signaling Framework through Logical Reasoning
International Multimedia Conference(2022)
摘要
ABSTRACTHuman minds spontaneously integrate two inherited cognitive capabilities: perception and reasoning to accomplish cognitive tasks such as problem solving, imagination, and causation. It is observed in the primate brains that perception offers the assistance required for problem comprehension, whilst the reasoning elucidates upon the facts recovered during perception in order to make a decision. The field of artificial intelligence (AI) thus considers perception and reasoning as two complementary areas that are realized by machine learning and logic programming, respectively. In this work, we propose a generative model using a collaborative guessing game of the kind first introduced by David Lewis in his famous work called the Lewis signaling game that is synonymous with the "20 Questions'' game. Our proposed model, Guess-It-Generator (GIG) is a collaborative framework that engages two recurrent neural networks in a guessing game. GIG unifies perception and reasoning with a view to generating labeled images by capturing, (X, y), the underlying density of a data distribution, i.e. (X, y) - p(X, y). An encoder attends to a region of the input image and encodes that onto a latent variable that acts as a perception signal to a decoder. In contrast, the decoder leverages on the perception signals to guess the image and verifies the guess by reasoning with logical facts derived from the domain knowledge. Our experiments and comprehensive studies on seven datasets: PCAM, Chest-Xray-14, FIRE, HAM10000 from the medical domain, and CIFAR 10, LSUN, ImageNet, among standard benchmark datasets, show significant promise for the proposed method.
更多查看译文
AI 理解论文
溯源树
样例
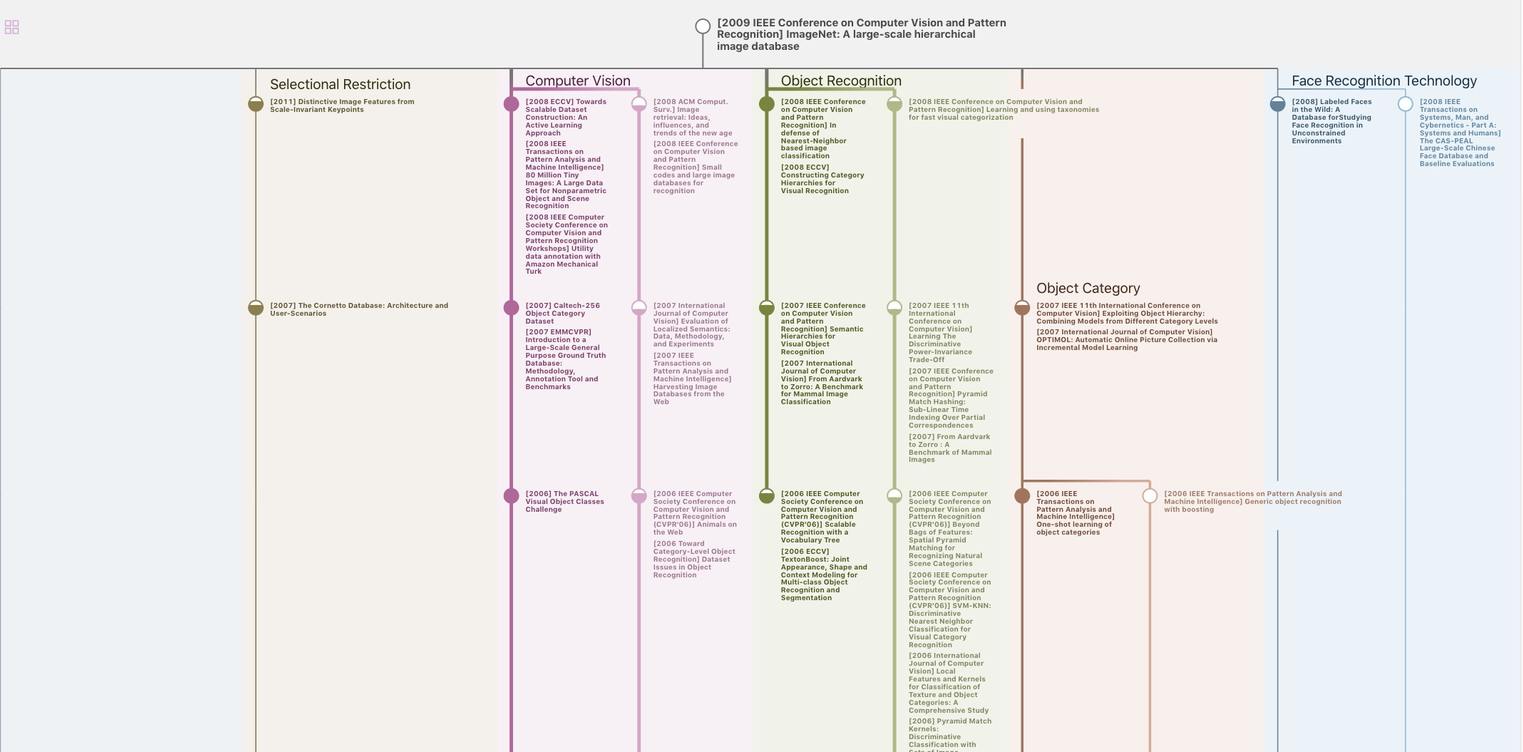
生成溯源树,研究论文发展脉络
Chat Paper
正在生成论文摘要