Visual Knowledge Graph for Human Action Reasoning in Videos
International Multimedia Conference(2022)
摘要
ABSTRACTAction recognition has been traditionally treated as a high-level video classification problem. However, such a manner lacks the detailed and semantic understanding of body movement, which is the critical knowledge to explain and infer complex human actions. To fill this gap, we propose to summarize a novel visual knowledge graph from over 15M detailed human annotations, for describing action as the distinct composition of body parts, part movements and interactive objects in videos. Based on it, we design a generic multi-modal Action Knowledge Understanding (AKU) framework, which can progressively infer human actions from body part movements in the videos, with assistance of visual-driven semantic knowledge mining. Finally, we validate AKU on the recent Kinetics-TPS benchmark, which contains body part parsing annotations for detailed understanding of human action in videos. The results show that, our AKU significantly boosts various video backbones with explainable action knowledge in both supervised and few shot settings, and outperforms the recent knowledge-based action recognition framework, e.g., our AKU achieves 83.9% accuracy on Kinetics-TPS while PaStaNet achieves 63.8% accuracy under the same backbone. The codes and models will be released at https://github.com/mayuelala/AKU.
更多查看译文
AI 理解论文
溯源树
样例
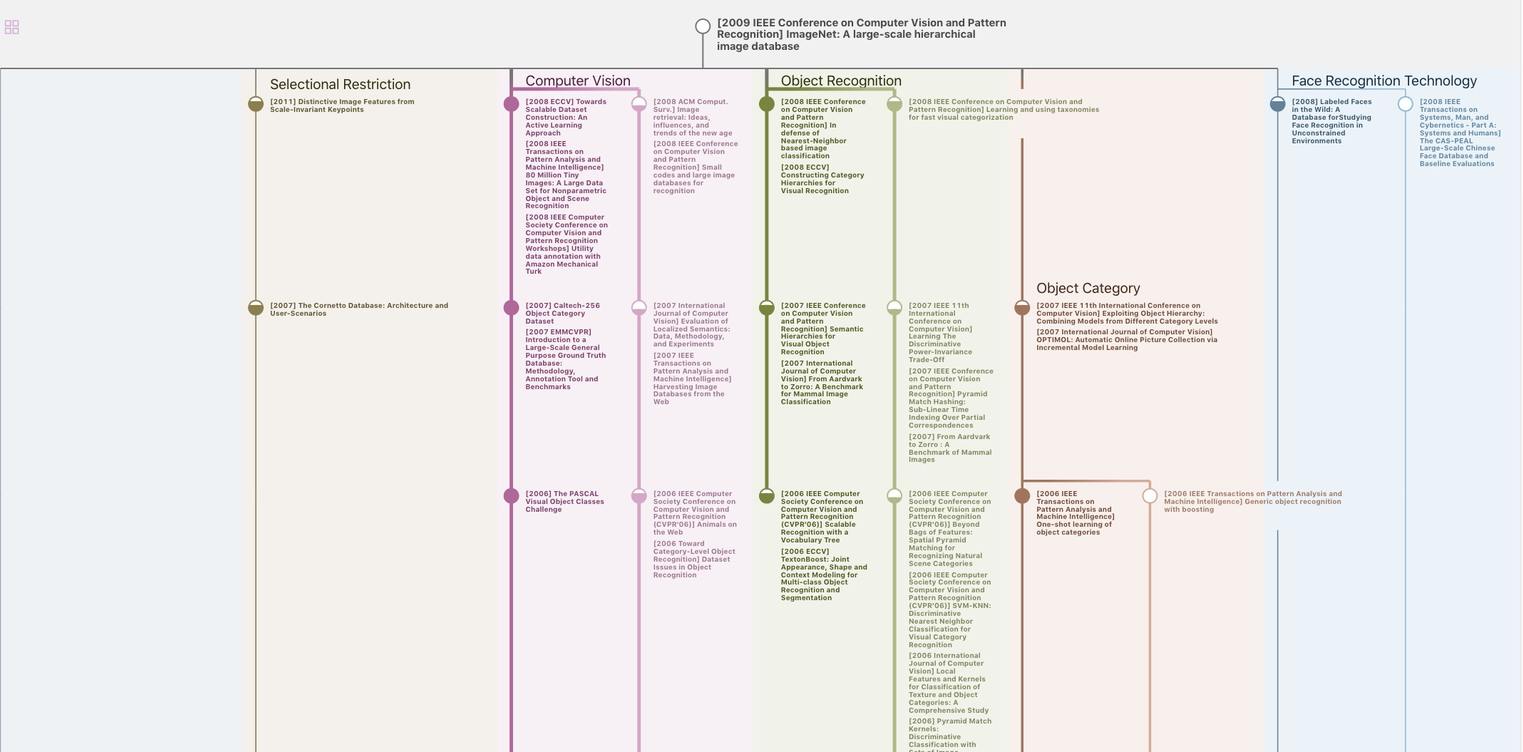
生成溯源树,研究论文发展脉络
Chat Paper
正在生成论文摘要