Point Cloud Completion via Multi-Scale Edge Convolution and Attention
PROCEEDINGS OF THE 30TH ACM INTERNATIONAL CONFERENCE ON MULTIMEDIA, MM 2022(2022)
摘要
Point cloud completion aims to recover a complete shape of a 3D object from its partial observation. Existing methods usually predict complete shapes from global representations, consequently, local geometric details may be ignored. Furthermore, they tend to overlook relations among different local regions, which are valuable during shape inference. To solve these problems, we propose a novel point cloud completion network based on multi-scale edge convolution and attention mechanism, named MEAPCN. We represent a point cloud as a set of embedded points, each of which contains geometric information of local patches around it. Firstly, we devise an encoder to extract multi-scale local features of the input point cloud and produce partial embedded points. Then, we generate coarse complete embedded points to represent the overall shape. In order to enrich features of complete embedded points, attention mechanism is utilized to selectively aggregate local informative features of partial ones. Lastly, we recover a fine-grained point cloud with highly detailed geometries using folding-based strategy. To better reflect real-world occlusion scenarios, we contribute a more challenging dataset, which consists of view-occluded partial point clouds. Experimental results on various benchmarks demonstrate that our method achieves a superior completion performance with much smaller model size and much lower computation cost.
更多查看译文
关键词
Shape completion,Edge convolution,Attention mechanism,Autoencoder
AI 理解论文
溯源树
样例
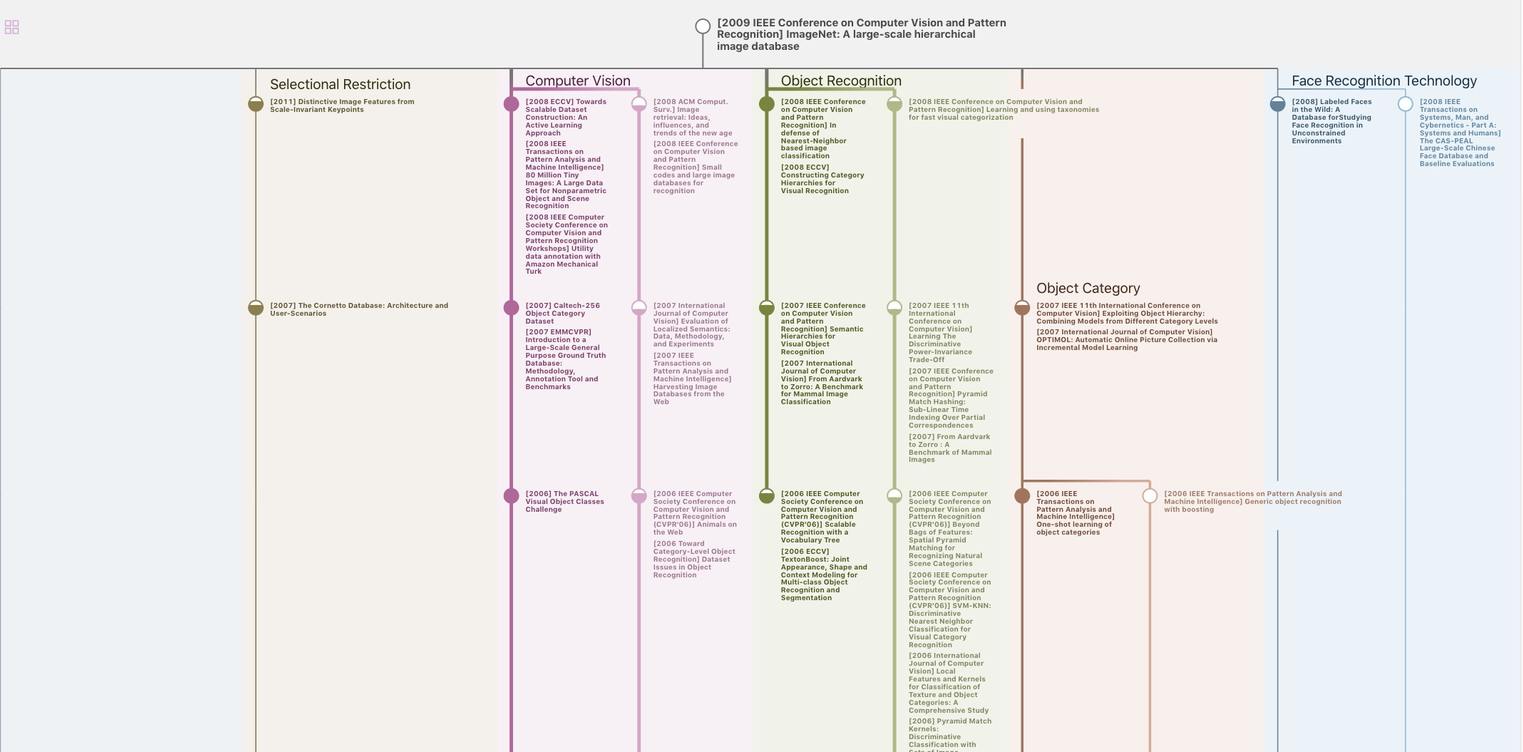
生成溯源树,研究论文发展脉络
Chat Paper
正在生成论文摘要