Non-Autoregressive Cross-Modal Coherence Modelling
International Multimedia Conference(2022)
摘要
ABSTRACTModelling the coherence of information is important for human to perceive and prehend the physical world. Existing works on coherence modelling mainly focus on single modality, which overlook the effect of information integration and semantic consistency across modalities. To fill the research gap, this paper targets at the cross-modal coherence modelling, specifically, the cross-modal ordering task. The task requires to not only explore the coherence information in single modality, but also leverage cross-modal information to model the semantic consistency between modalities. To this end, we propose a Non-Autoregressive Cross-modal Ordering Net (NACON) adopting a basic encoder-decoder architecture. Specifically, NACON is equipped with an order-invariant context encoder to model the unordered input set and a non-autoregressive decoder to generate ordered sequences in parallel. We devise a cross-modal positional attention module in NACON to take advantage of the cross-modal order guidance. To alleviate the repetition problem of non-autoregressive models, we introduce an elegant exclusive loss to constrain the ordering exclusiveness between positions and elements. We conduct extensive experiments on two assembled datasets to support our task, SIND and TACoS-Ordering. Experimental results show that the proposed NACON can effectively leverage cross-modal guidance and recover the correct order of the elements.The code is available at https://github.com/YiBin-CHN/CMCM.
更多查看译文
关键词
modelling,non-autoregressive,cross-modal
AI 理解论文
溯源树
样例
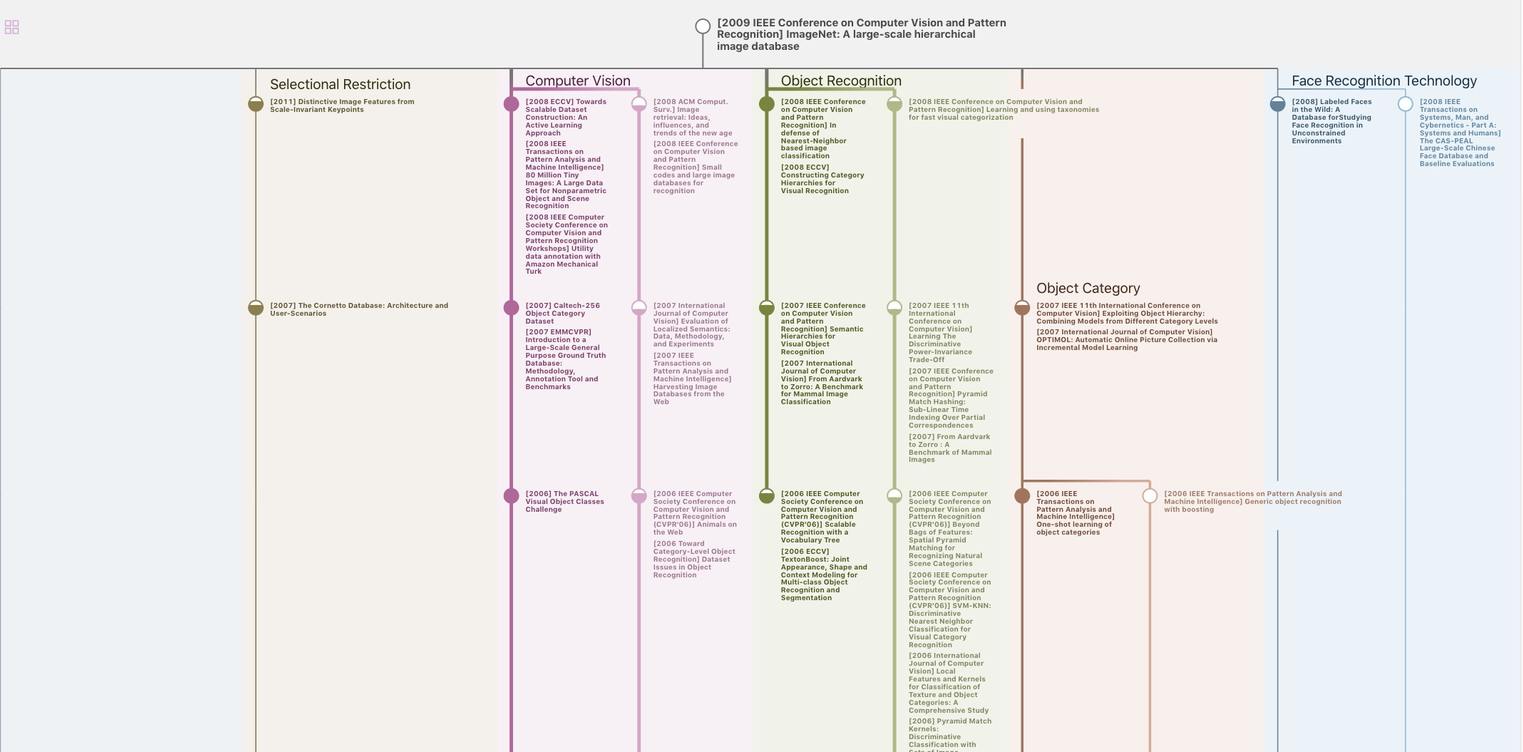
生成溯源树,研究论文发展脉络
Chat Paper
正在生成论文摘要