Sentiment-aware Classifier for Out-of-Context Caption Detection
International Multimedia Conference(2022)
摘要
ABSTRACTIn this work we propose additions to the COSMOS and COSMOS on Steroids pipelines for the detection of Cheapfakes for Task 1 of the ACM Grand Challenge for Detecting Cheapfakes. We compute sentiment features, namely polarity and subjectivity, using the news image captions. Multiple logistic regression results show that these sentiment features are significant in prediction of the outcome. We then combine the sentiment features with the four image-text features obtained in the aforementioned previous works to train an MLP. This classifies sets of inputs into being out-of-context (OOC) or not-out-of-context (NOOC). On a test set of 400 samples, the MLP with all features achieved a score of 87.25%, and that with only the image-text features a score of 88%. In addition to the challenge requirements, we also propose a separate pipeline to automatically construct caption pairs and annotations using the images and captions provided in the large, un-annotated training dataset. We hope that this endeavor will open the door for improvements, since hand-annotating cheapfake labels is time-consuming. To evaluate the performance on the test set, the Docker image with the models is available at: https://hub.docker.com/repository/docker/malkaddour/mmsys22cheapfakes. The open-source code for the project is accessible at: https://github.com/malkaddour/ACMM-22-Cheapfake-Detection-Sentiment-aware-Classifier-for-Out-of-Context-Caption-Detection.
更多查看译文
关键词
sentiment-aware,out-of-context
AI 理解论文
溯源树
样例
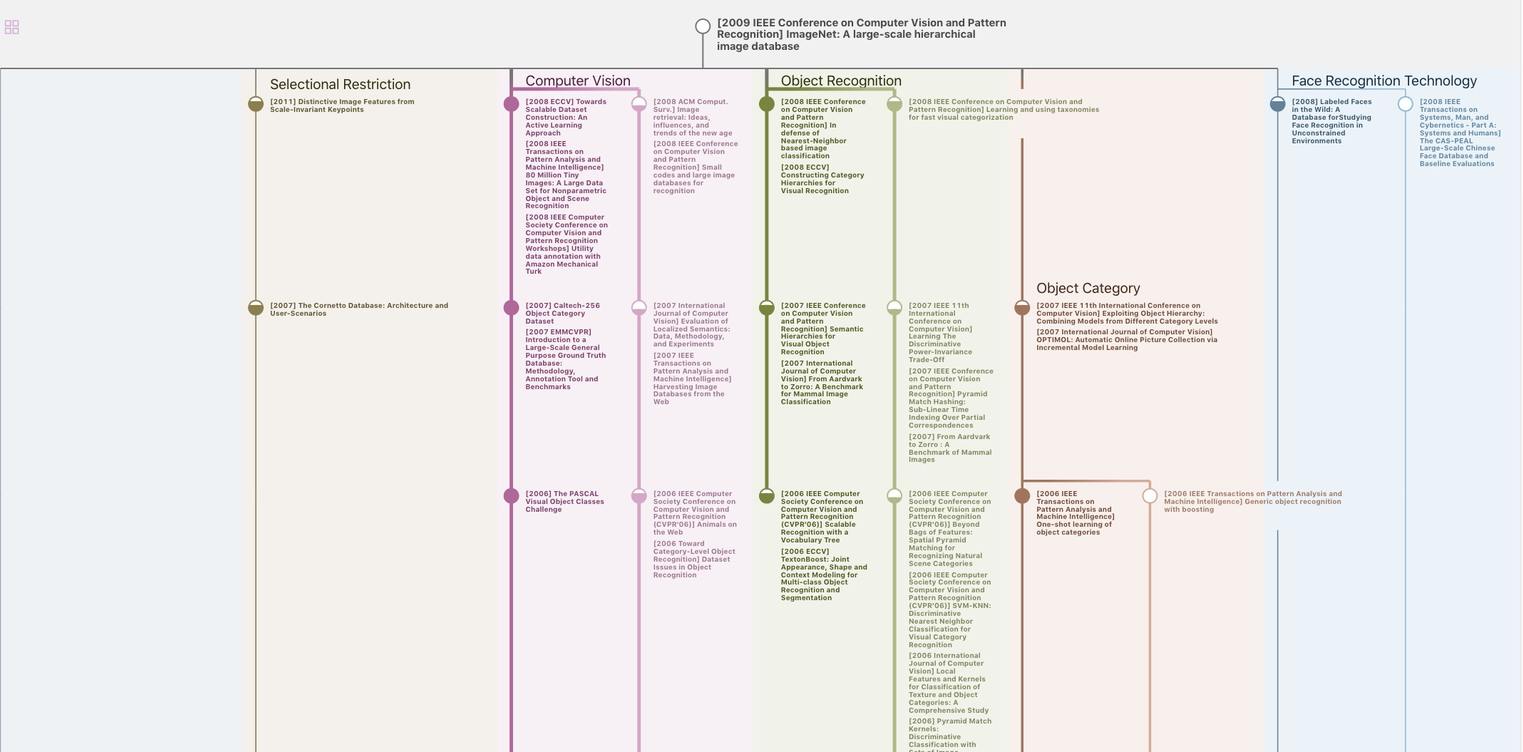
生成溯源树,研究论文发展脉络
Chat Paper
正在生成论文摘要