PSP: Progressive Space Pruning for Efficient Graph Neural Architecture Search
2022 IEEE 38TH INTERNATIONAL CONFERENCE ON DATA ENGINEERING (ICDE 2022)(2022)
摘要
Recently, graph neural network (GNN) has achieved great success in many graph learning tasks such as node classification and graph classification. However, there is no single GNN architecture that can fit different graph datasets. Designing an effective GNN for a specific graph dataset requires considerable expert experience and huge computational costs. Inspired by the success of neural architecture search (NAS), searching the GNN architectures automatically has attracted more and more attention. Motivated by the fact that the search space plays a critical role in the NAS, we propose a novel and effective graph neural architecture search method called PSP from the perspective of search space design in this paper. We first propose an expressive search space composed of multiple cells. Instead of searching the entire architecture, we focus on searching the architecture of the cell. Then, we propose a progressive space pruning-based algorithm to search the architectures efficiently. Moreover, the data-specific search spaces and architectures obtained by PSP can be transferred to new graph datasets based on meta-learning. Extensive experimental results on different types of graph datasets reveal that PSP outperforms the state-of-theart handcrafted architectures and the existing NAS methods in terms of effectiveness and efficiency.
更多查看译文
关键词
graph neural network, neural architecture search, search space pruning, transfer learning
AI 理解论文
溯源树
样例
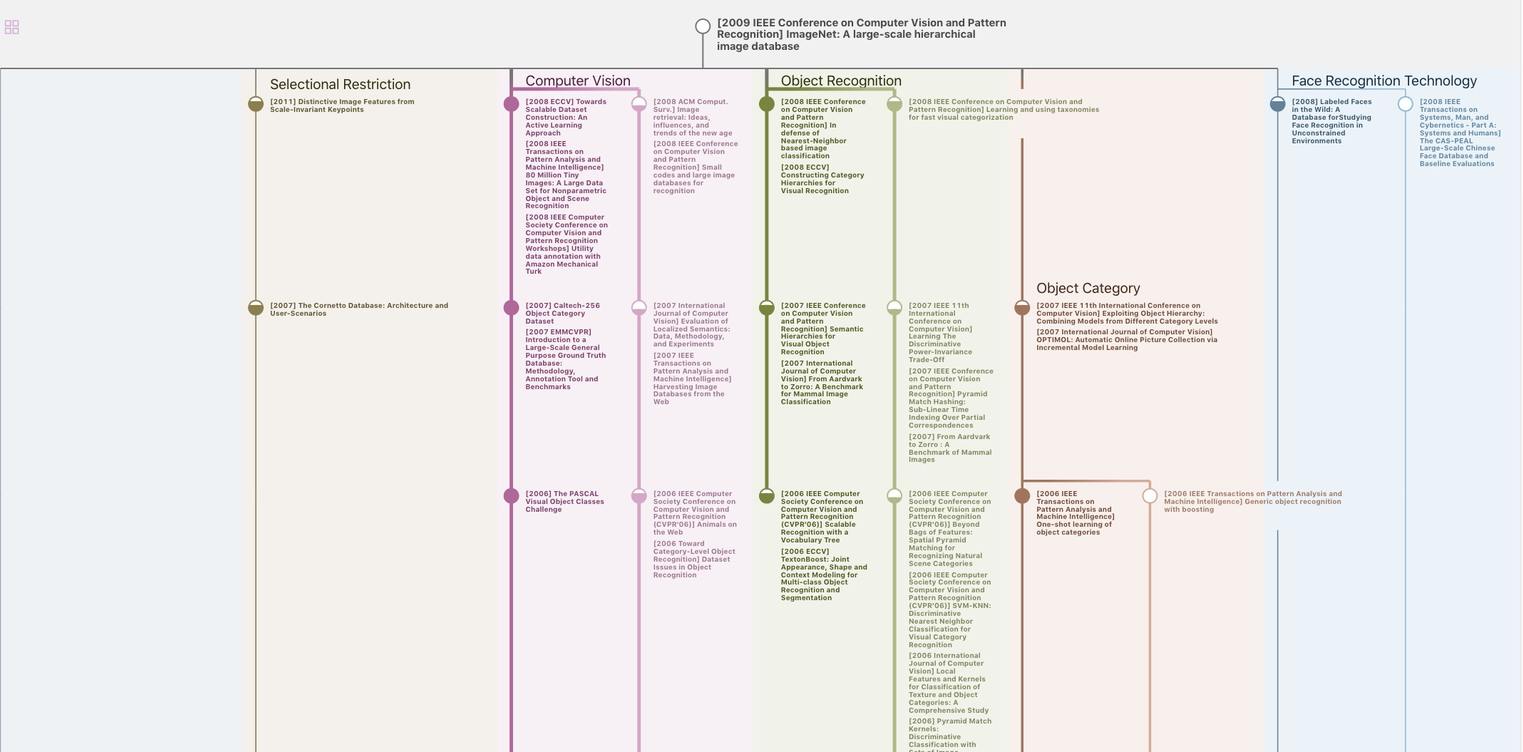
生成溯源树,研究论文发展脉络
Chat Paper
正在生成论文摘要