Distributed Influence Maximization for Large-Scale Online Social Networks
2022 IEEE 38th International Conference on Data Engineering (ICDE)(2022)
摘要
Thanks to billions of users in online social networks (OSNs), viral marketing becomes one of the most effective promotion channels for various new products or campaigns. Influence maximization is a classic problem in viral marketing, which has been extensively studied in the past two decades. Existing algorithms for influence maximization, however, mostly focus on single machine processing. To address the influence maximization problem on a massive scale, we design distributed algorithms via a cluster of machines, which can effectively speed up the computation while maintaining the state-of-the-art (1 -1/e-c)-approximation guarantee. Our distributed algorithms consist of two building blocks: (i) distributed reverse influence sampling, and (ii) element-distributed maximum coverage. We carry out extensive experiments on real datasets with millions of nodes and billions of edges to demonstrate the scalability of our distributed algorithms for both influence maximization and maximum coverage. In particular, our distributed algorithms accelerate the state-of-the-art IMM algorithm by 31x-56x times using a machine with 64 cores.
更多查看译文
关键词
Influence maximization,Distributed algorithms,Online social networks
AI 理解论文
溯源树
样例
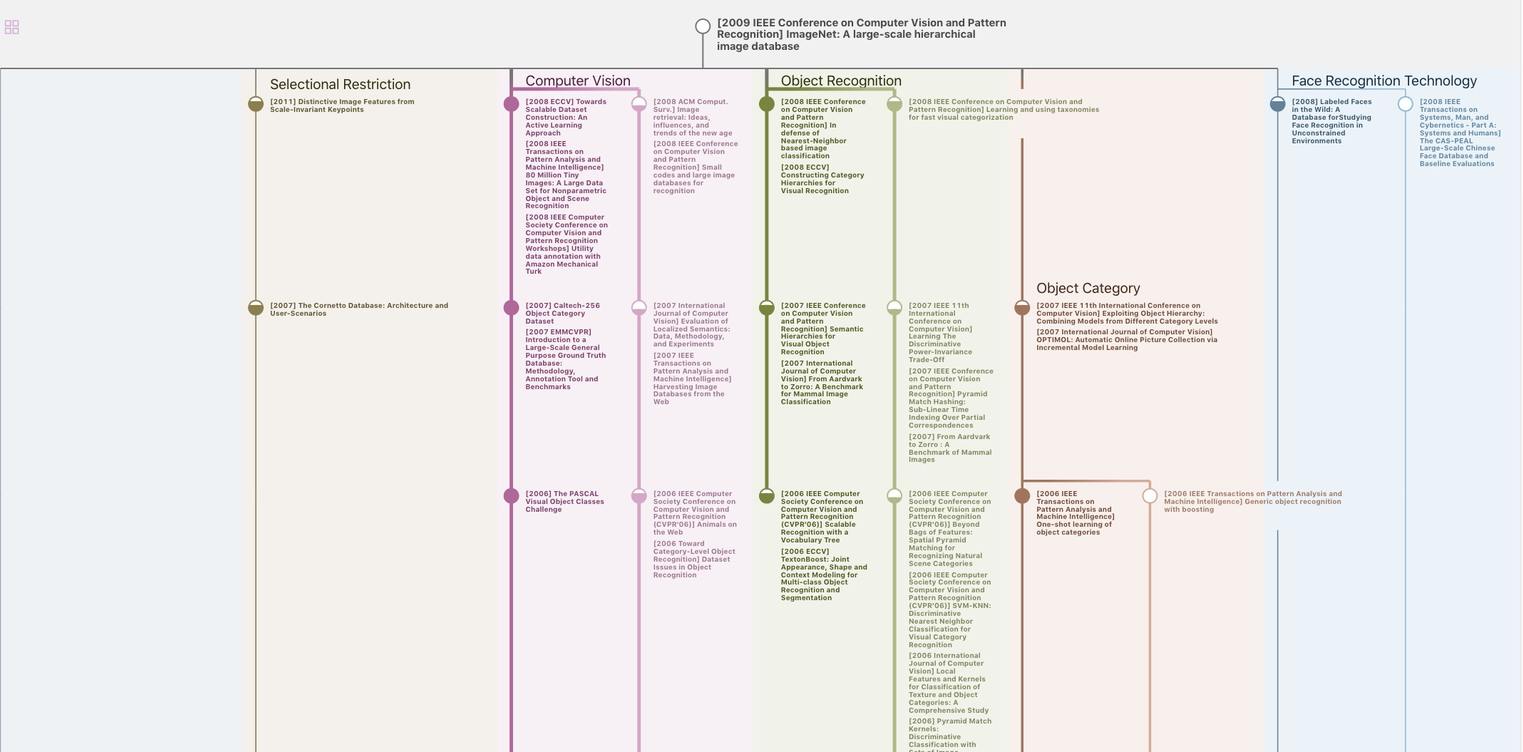
生成溯源树,研究论文发展脉络
Chat Paper
正在生成论文摘要