Cooperative Route Planning Framework for Multiple Distributed Assets in Maritime Applications
PROCEEDINGS OF THE 2022 INTERNATIONAL CONFERENCE ON MANAGEMENT OF DATA (SIGMOD '22)(2022)
摘要
This work formalizes the Route Planning Problem (RPP), wherein a set of distributed assets (e.g., ships, submarines, unmanned systems) simultaneously plan routes to optimize a team goal (e.g., find the location of an unknown threat or object in minimum time and/or fuel consumption) while ensuring that the planned routes satisfy certain constraints (e.g., avoiding collisions and obstacles). This problem becomes overwhelmingly complex for multiple distributed assets as the search space grows exponentially to design such plans. The RPP is formalized as a Team Discrete Markov Decision Process (TDMDP) and we propose a Multi-agent Multi-objective Reinforcement Learning (MaMoRL) framework for solving it. We investigate challenges in deploying the solution in real-world settings and study approximation opportunities. We experimentally demonstrate MaMoRL's effectiveness on multiple real-world and synthetic grids, as well as for transfer learning. MaMoRL is deployed for use by the Naval Research Laboratory - Marine Meteorology Division (NRL-MMD), Monterey, CA.
更多查看译文
关键词
Route planning, Multi-Agent Reinforcement Learning, Function Approximation, Scalable Solution Design, Data Management for AI
AI 理解论文
溯源树
样例
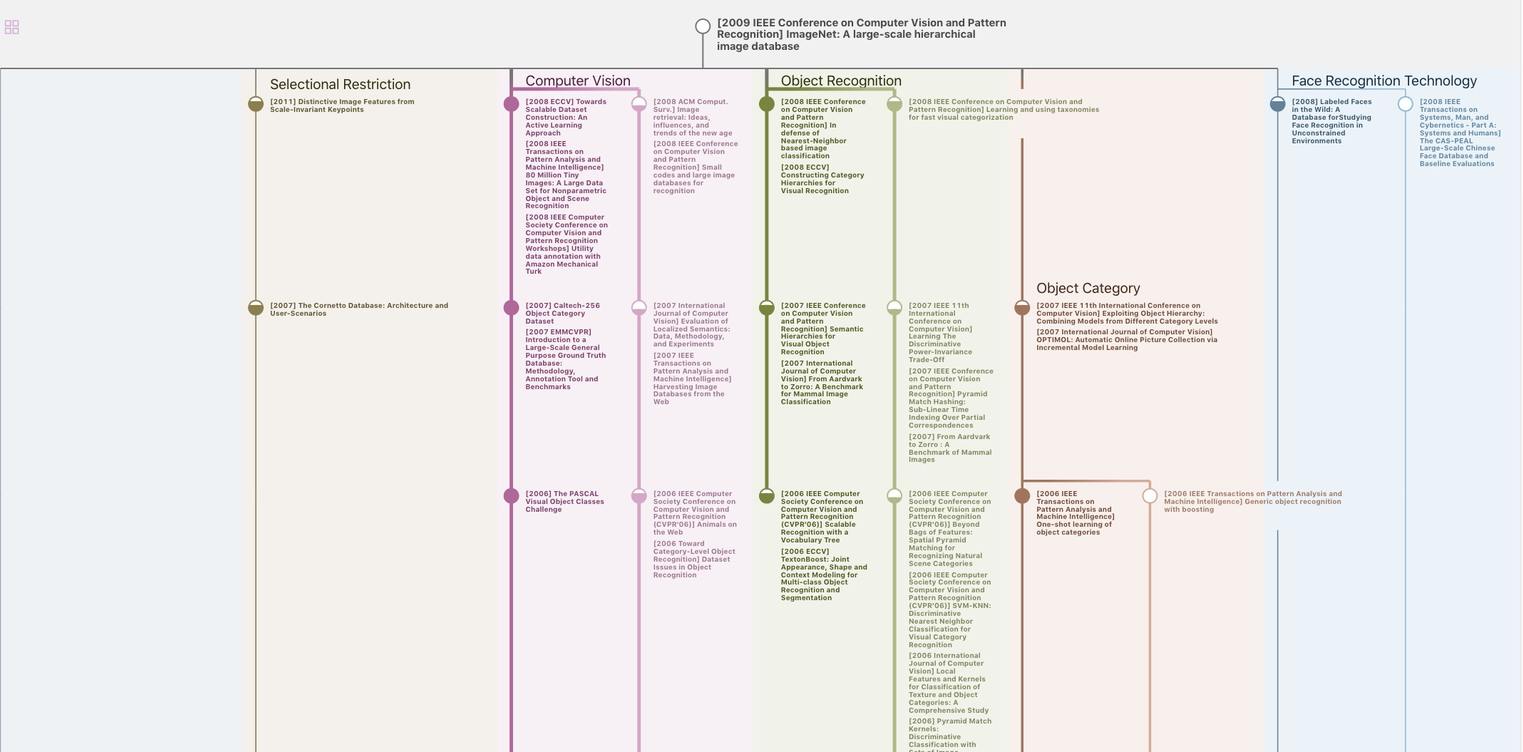
生成溯源树,研究论文发展脉络
Chat Paper
正在生成论文摘要