FiGO: Fine-Grained Query Optimization in Video Analytics
PROCEEDINGS OF THE 2022 INTERNATIONAL CONFERENCE ON MANAGEMENT OF DATA (SIGMOD '22)(2022)
摘要
Video database management systems (VDBMSs) enable automated analysis of videos at scale using computationally-intensive deep learning models. To reduce the computational overhead of these models, researchers have proposed two techniques: (1) leveraging a specialized, lightweight model to filter out irrelevant frames or to directly answer the query, and (2) using a cascade of models of increasing complexity to answer the query. For both techniques, the query optimizer generates a coarse-grained query plan for the entire video. These techniques suffer from four limitations: (1) lower query accuracy over hard-to-detect predicates, (2) lower filtering efficacy with frequently-occurring objects, (3) lower accuracy due to non-trivial model cascade configuration, and (4) missed optimization opportunities due to coarse-grained planning for the entire video. In this paper, we present FiGO to tackle these limitations. The design of FiGO is centered around three techniques. First, it uses an ensemble of models to support a range of throughput-accuracy tradeoffs. Second, it adopts a fine-grained approach to query optimization. It processes different chunks of the video using different models in the given ensemble to meet the user's accuracy requirement. Lastly, it uses a lightweight technique to prune the model ensemble to lower the query optimization time. We empirically show that these techniques enable FiGO to outperform the state-of-the-art systems for processing queries over videos by 3.3x on average across four video datasets.
更多查看译文
关键词
Video Analytics
AI 理解论文
溯源树
样例
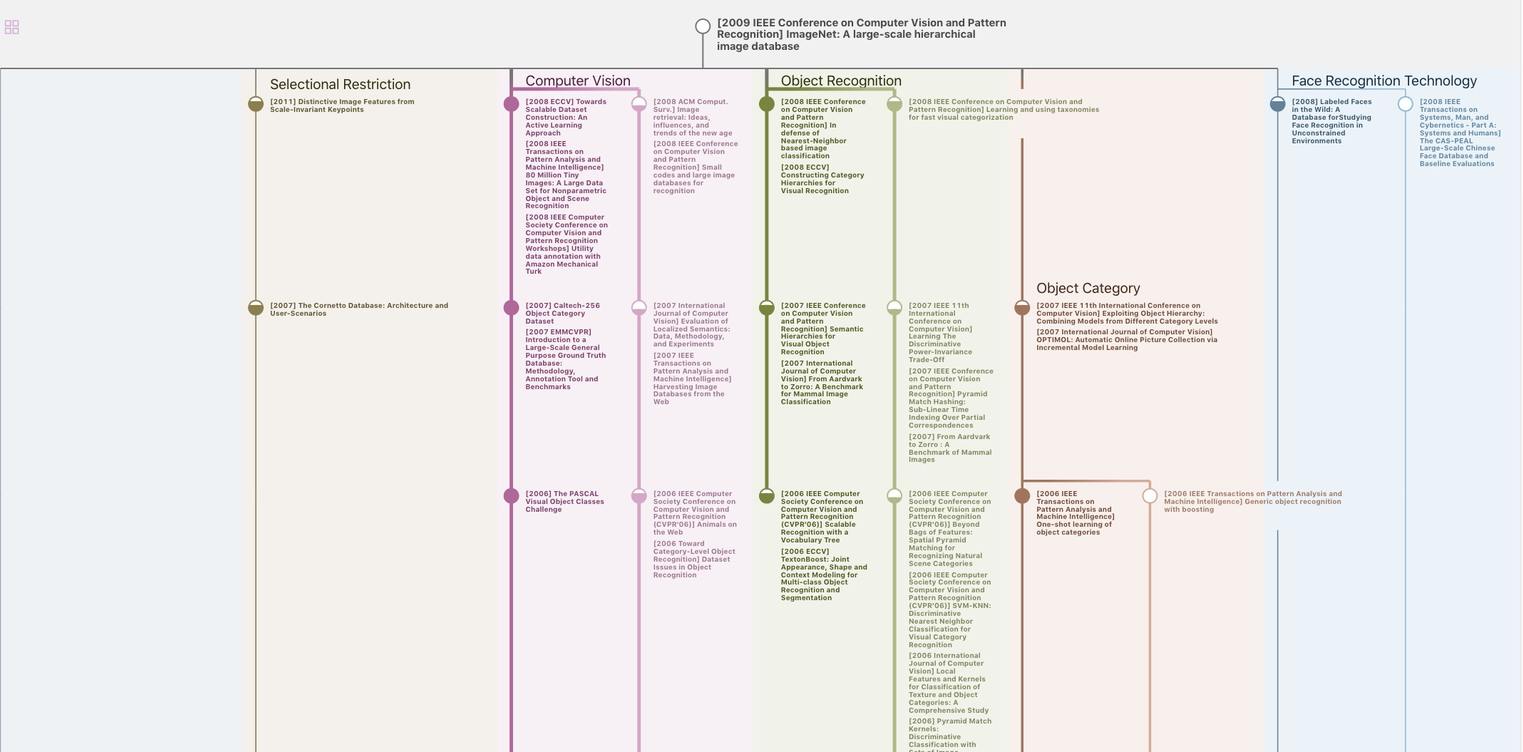
生成溯源树,研究论文发展脉络
Chat Paper
正在生成论文摘要