LossLeaP: Learning to Predict for Intent-Based Networking
IEEE INFOCOM 2022 - IEEE Conference on Computer Communications(2022)
摘要
Intent-Based Networking mandates that high-level human-understandable intents are automatically interpreted and implemented by network management entities. As a key part in this process, it is required that network orchestrators activate the correct automated decision model to meet the intent objective. In anticipatory networking tasks, this requirement maps to identifying and deploying a tailored prediction model that can produce a forecast aligned with the specific –and typically complex– network management goal expressed by the original intent. Current forecasting models for network demands or network management optimize generic, non-flexible, and manually designed objectives, hence do not fulfil the needs of anticipatory Intent-Based Networking. To close this gap, we propose LossLeaP, a novel forecasting model that can autonomously learn the relationship between the prediction and the target management objective, steering the former to minimize the latter. To this end, LossLeaP adopts an original deep learning architecture that advances current efforts in automated machine learning, towards a spontaneous design of loss functions for regression tasks. Extensive experiments in controlled environments and in practical application case studies prove that LossLeaP outperforms a wide range of benchmarks, including state-of-the-art solutions for network capacity forecasting.
更多查看译文
关键词
LossLeaP,high-level human-understandable intents,network management entities,network orchestrators,intent objective,anticipatory networking tasks,tailored prediction model,forecasting models,network demands,target management objective,automated machine learning,network capacity forecasting,intent-based networking mandates,automated decision model,deep learning architecture,spontaneous design,regression tasks
AI 理解论文
溯源树
样例
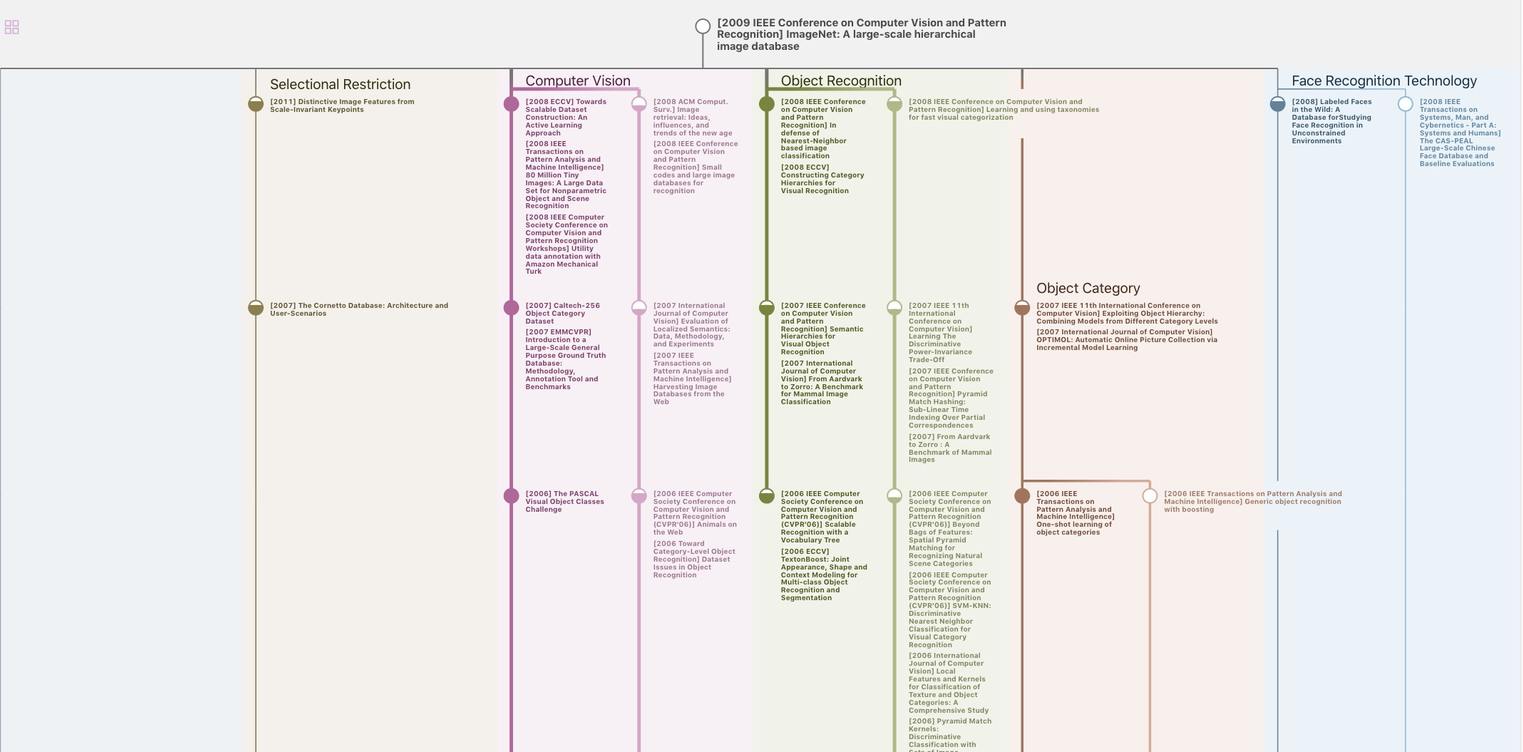
生成溯源树,研究论文发展脉络
Chat Paper
正在生成论文摘要