Building a High-performance Fine-grained Deduplication Framework for Backup Storage with High Deduplication Ratio
USENIX Annual Technical Conference (USENIX ATC)(2022)
摘要
Fine-grained deduplication, which first removes identical chunks and then eliminates redundancies between similar but non-identical chunks (i.e., delta compression), could exploit workloads' compressibility to achieve a very high deduplication ratio but suffers from poor backup/restore performance. This makes it not as popular as chunk-level deduplication thus far. This is because allowing workloads to share more references among similar chunks further reduces spatial/temporal locality, causes more I/O overhead, and leads to worse backup/restore performance. In this paper, we address issues for different forms of poor locality with several techniques, and propose MeGA, which achieves backup and restore speed close to chunk-level deduplication while preserving fine-grained deduplication's significant deduplication ratio advantage. Specifically, MeGA applies (1) a backup-workflow-oriented delta selector to address poor locality when reading base chunks, and (2) a delta-friendly data layout and "Always-Forward-Reference" traversing in the restore workflow to deal with the poor spatial/temporal locality of deduplicated data. Evaluations on four datasets show that MeGA achieves a better performance than other fine-grained deduplication approaches. In particular, compared with the traditional greedy approach, MeGA achieves a 4.47-34.45x higher backup performance and a 30-105x higher restore performance while maintaining a very high deduplication ratio.
更多查看译文
AI 理解论文
溯源树
样例
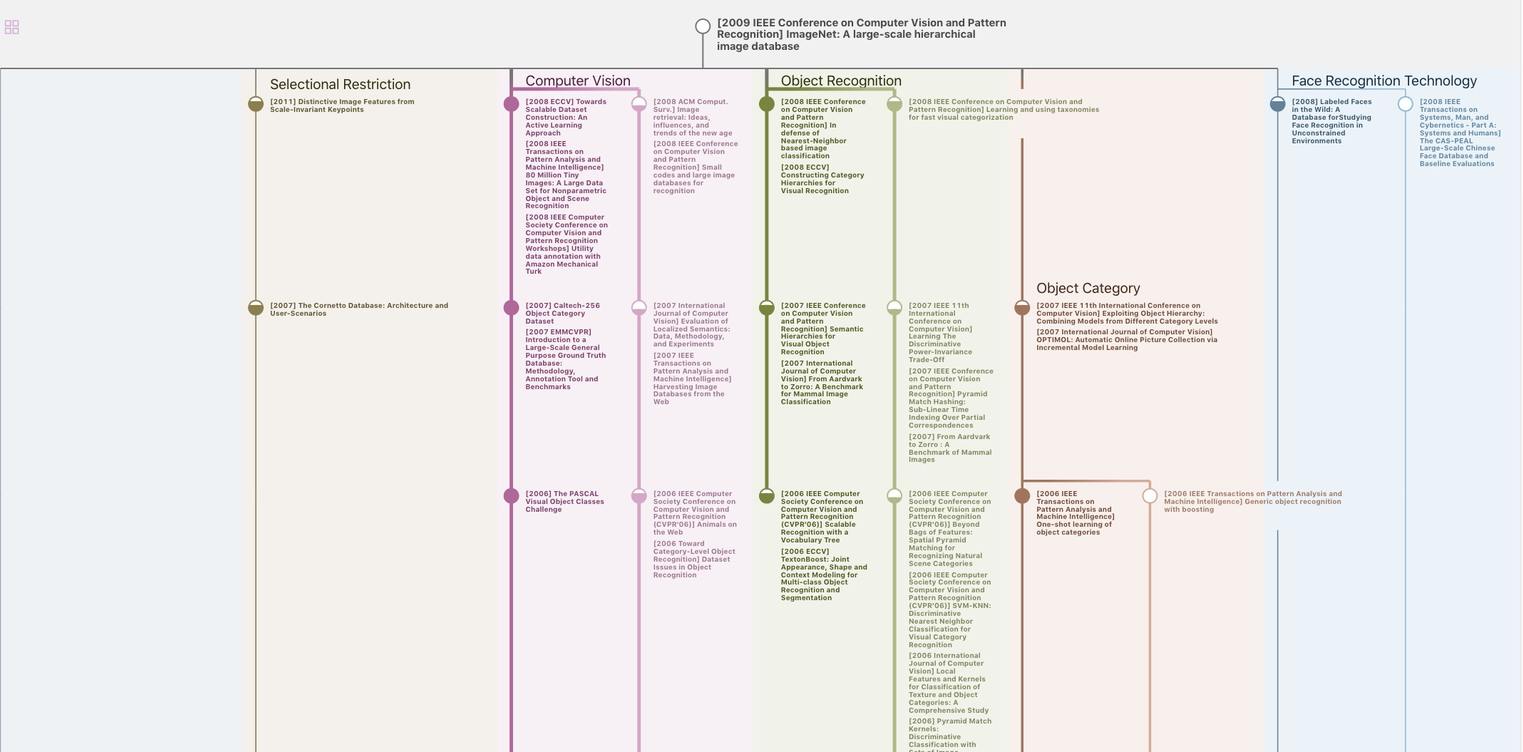
生成溯源树,研究论文发展脉络
Chat Paper
正在生成论文摘要