Continuous and Unified Person Re-Identification
IEEE SIGNAL PROCESSING LETTERS(2022)
摘要
Person re-identification (ReID) aims to match pedestrian images across disjoint cameras. Mainstream Re-ID tasks focus on training ReID models once using all the data, which become limited in some real-world scenarios where training data tends to arrive in stages. To match scenarios where training data is incrementally available, some works began to explore ReID task that can make efficient use of piecemeal new data. However, due to the limitations of the training and testing setups, these efforts are still preliminary explorations. In this letter, we explore a novel yet harder Continuous and Unified ReID (CUReID), which not only enables to continuously learn discrimination knowledge from data streams with style differences, but also to be uniformly evaluated discriminatory capability on all the data (seen and unseen). Furthermore, we propose a novel Generalized Feature Decoupled Learning (GFDL) framework for CUReID, which characterizes by introducing alternate training with extra images to solve the problem of optimization divergence between regularisation (learning new knowledge) and generalization (anti-forgetting old knowledge) tasks. In our newly proposed benchmark setup, GFDL achieves the state-of-the-art performance.
更多查看译文
关键词
Task analysis, Training, Data models, Predictive models, Optimization, Training data, Feature extraction, Person re-identification, continuous learning, unified processing, alternate learning
AI 理解论文
溯源树
样例
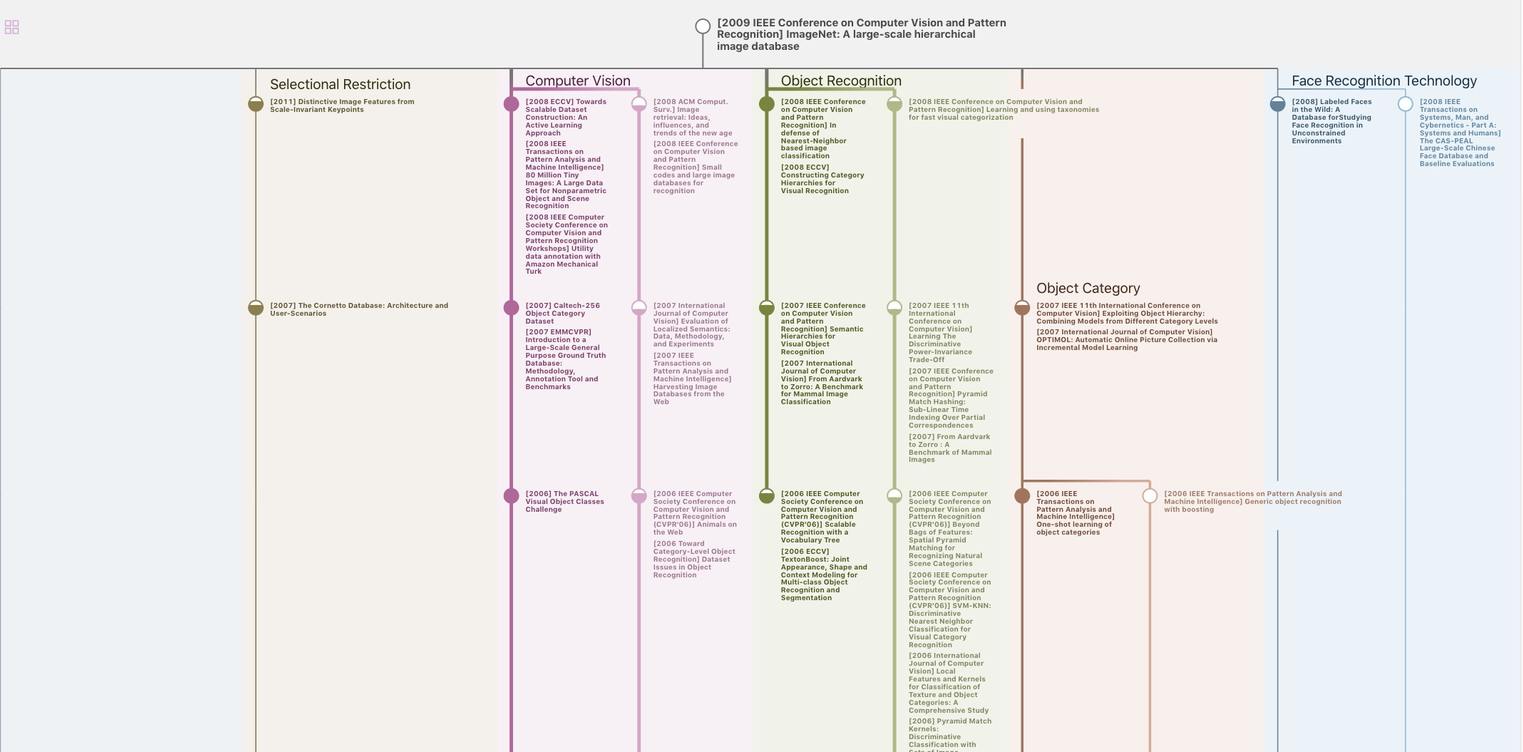
生成溯源树,研究论文发展脉络
Chat Paper
正在生成论文摘要