LESSL: Can LEGO sampling and collaborative optimization contribute to self-supervised learning?
Information Sciences(2022)
摘要
Self-supervised visual representation learning (SSL) aims to extract the most distinctive features from unlabeled datasets to overcome challenges of labor-intensive and time-consuming data annotation. Recent advances in this area are dominated by contrastive learning-based methods with various sampling strategies and optimization objectives. Unfortunately, these methods are limited by larger batch sizes and longer training epochs, resulting in non-negligible computational resource consumption and memory footprint. Unlike prior sampling manners and inspired by LEGO bricks, we design a comprehensive interleave sampling module to fully exploit unlabeled datasets, which seamlessly integrates the advantages of semantic and spatial relations complementarity from the unlabeled image via optimizing two objectives. Specifically, the unlabeled images are sampled in a dense and interleaved manner, which breaks down barriers of under-utilization of datasets existing in state-of-the-art methods. Meanwhile, the redefined collaborative optimization term is designed to alleviate feature-specificity and implicitly explore sample relationships during training, which effectively improves the performance of downstream tasks on various datasets. Besides, we focus on high-level semantic features and utilize the spatial structure relationships provided by the unlabeled datasets to ensure the learned features with low-level texture characteristics. Extensive experiments on four types of datasets demonstrate that our method performs favorably against the state-of-the-art SSL methods.
更多查看译文
关键词
Self-supervised learning,LEGO sampling,Contrastive learning,Collaborative optimization
AI 理解论文
溯源树
样例
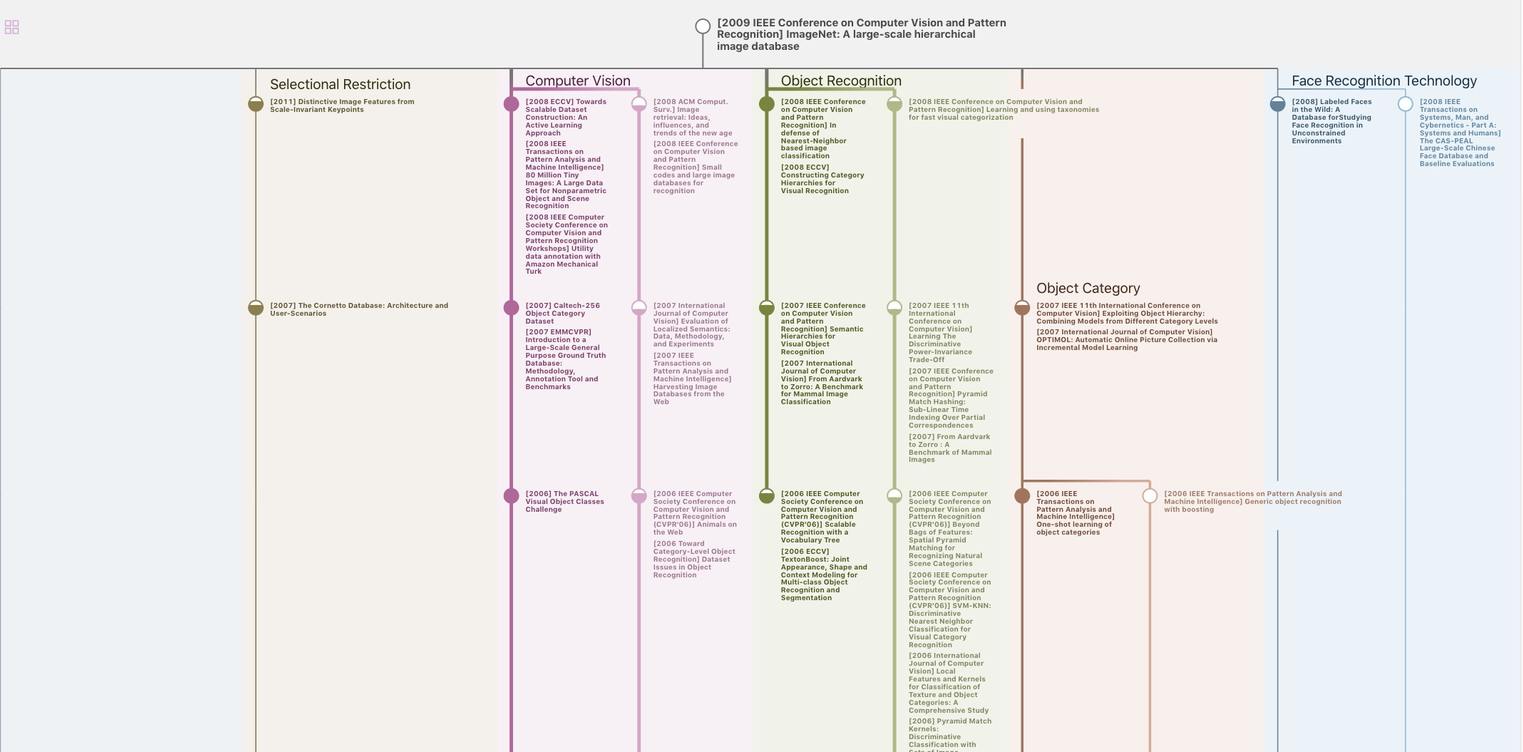
生成溯源树,研究论文发展脉络
Chat Paper
正在生成论文摘要