Prediction of Crack Growth Life at Elevated Temperatures with Neural Network-Based Learning Schemes
Aerospace(2022)
摘要
Applying the machine learning (ML) technique in the modelling of crack growth (CG) behavior is a potential way to improve the efficiency and precision of CG assessment. However, research in this field at elevated temperatures is limited, although a lot of achievements have been obtained in CG assessment at room temperature. Neutral network (NN)-based methods to model the CG at elevated temperatures were therefore investigated in this paper. An “indirect” method (NNK method) assessing the CG by modelling and integrating the crack growth rate (CGR) was established. A “direct” method (ENNIL method) was built by further developing the NN-based increment learning scheme. The NNK method shows high accuracy in CG prediction with relatively short CG life, while the ENNIL method gives perfectly predicted results for cases with relatively long CG life. The combination of these two methods may be an effective way to further improve CG assessment at elevated temperatures.
更多查看译文
关键词
crack growth, neural network, creep-fatigue, multiple increments, machine learning
AI 理解论文
溯源树
样例
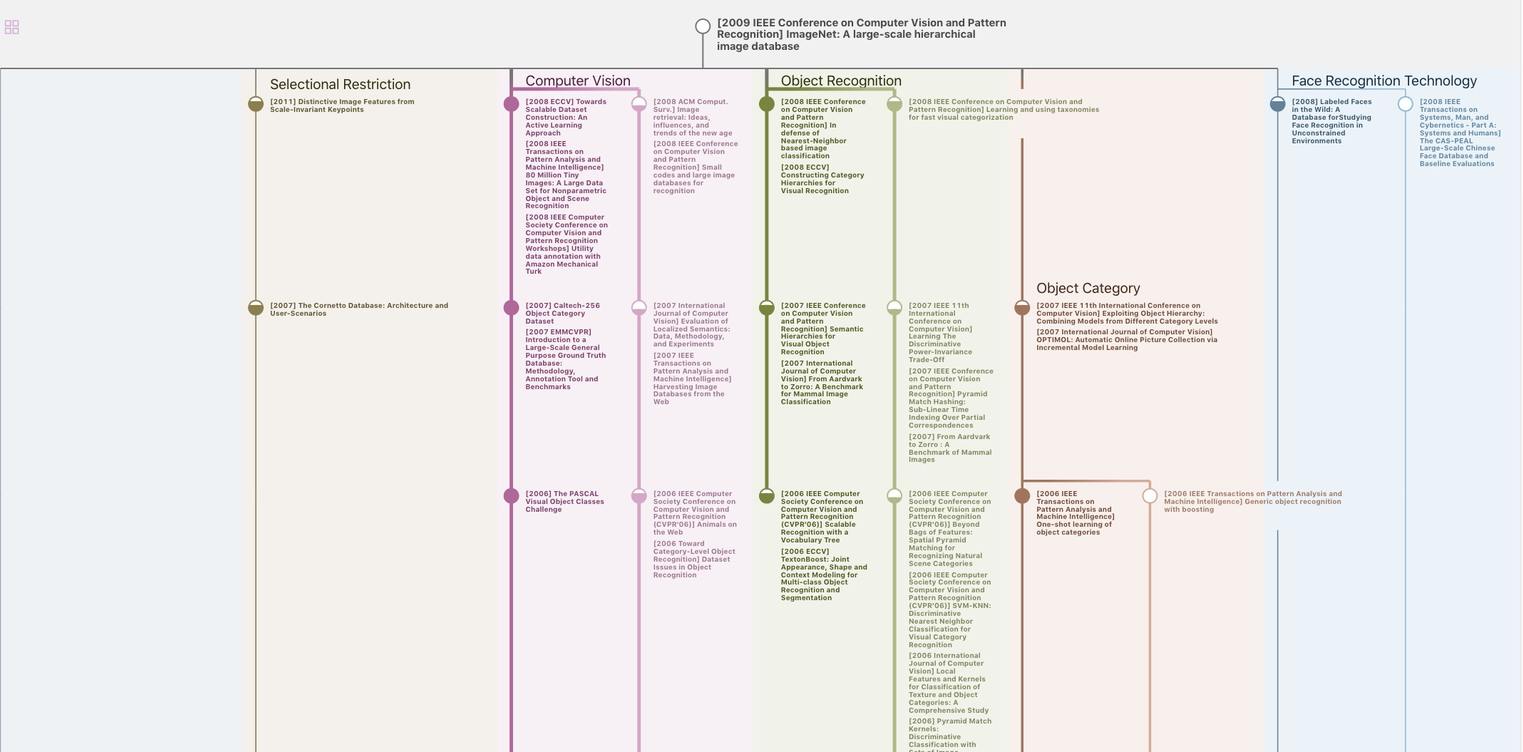
生成溯源树,研究论文发展脉络
Chat Paper
正在生成论文摘要