Asymmetric Student-Teacher Networks for Industrial Anomaly Detection
WACV(2023)
Abstract
Industrial defect detection is commonly addressed with anomaly detection (AD) methods where no or only incomplete data of potentially occurring defects is available. This work discovers previously unknown problems of studentteacher approaches for AD and proposes a solution, where two neural networks are trained to produce the same output for the defect-free training examples. The core assumption of student-teacher networks is that the distance between the outputs of both networks is larger for anomalies since they are absent in training. However, previous methods suffer from the similarity of student and teacher architecture, such that the distance is undesirably small for anomalies. For this reason, we propose asymmetric student-teacher networks (AST). We train a normalizing flow for density estimation as a teacher and a conventional feed-forward network as a student to trigger large distances for anomalies: The bijectivity of the normalizing flow enforces a divergence of teacher outputs for anomalies compared to normal data. Outside the training distribution the student cannot imitate this divergence due to its fundamentally different architecture. Our AST network compensates for wrongly estimated likelihoods by a normalizing flow, which was alternatively used for anomaly detection in previous work. We show that our method produces state-of-the-art results on the two currently most relevant defect detection datasets MVTec AD and MVTec 3D-AD regarding image-level anomaly detection on RGB and 3D data.
MoreTranslated text
Key words
industrial anomaly detection,anomaly detection,networks,student-teacher
AI Read Science
Must-Reading Tree
Example
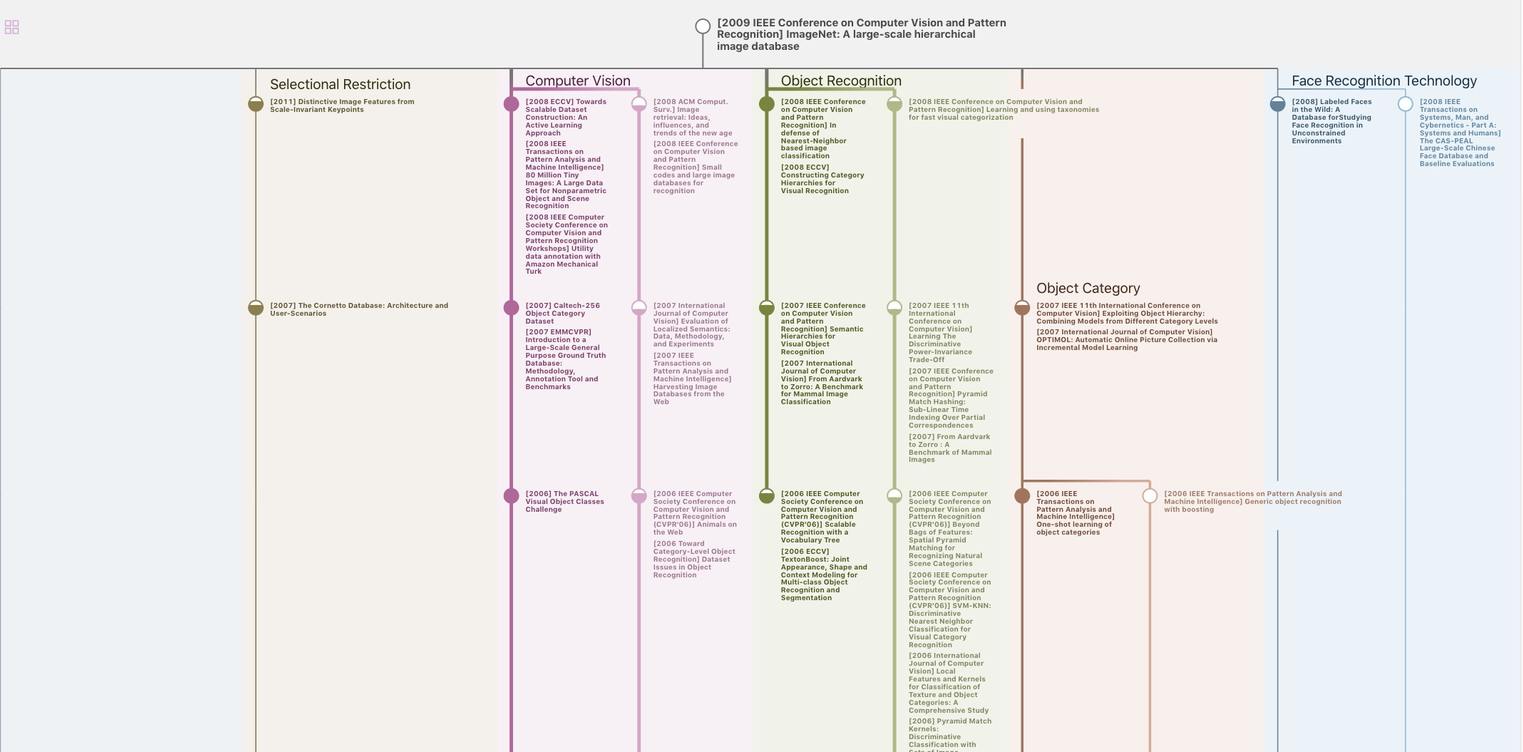
Generate MRT to find the research sequence of this paper
Chat Paper
Summary is being generated by the instructions you defined