Controllable Style Transfer via Test-time Training of Implicit Neural Representation
PATTERN RECOGNITION(2024)
摘要
Existing CNN-based style transfer frameworks have suffered from inaccurate control of pixel-wise stylization. As the CNN operation is designed based on kernel-wise operation, such a design unavoidably makes pixels affect each other. To mitigate this problem, we propose a controllable style transfer framework that leverages Implicit Neural Representation to encode each pixel respectively and optimize each style and content pair via test-time training. Unlike previous CNN-based style transfer frameworks, this formulation naturally enables accurate pixel-wise stylization control. In addition, to give explicit controllability on the degree of stylization, we define two vectors that represent the content and style respectively, enabling control by interpolating these vectors. We further demonstrate that, after being test-time trained once, our framework can show a various range of applications by precisely controlling the stylized images pixel-wise and freely adjusting image resolution and degree of stylization without further optimization or training.
更多查看译文
关键词
Style transfer,Implicit neural representation
AI 理解论文
溯源树
样例
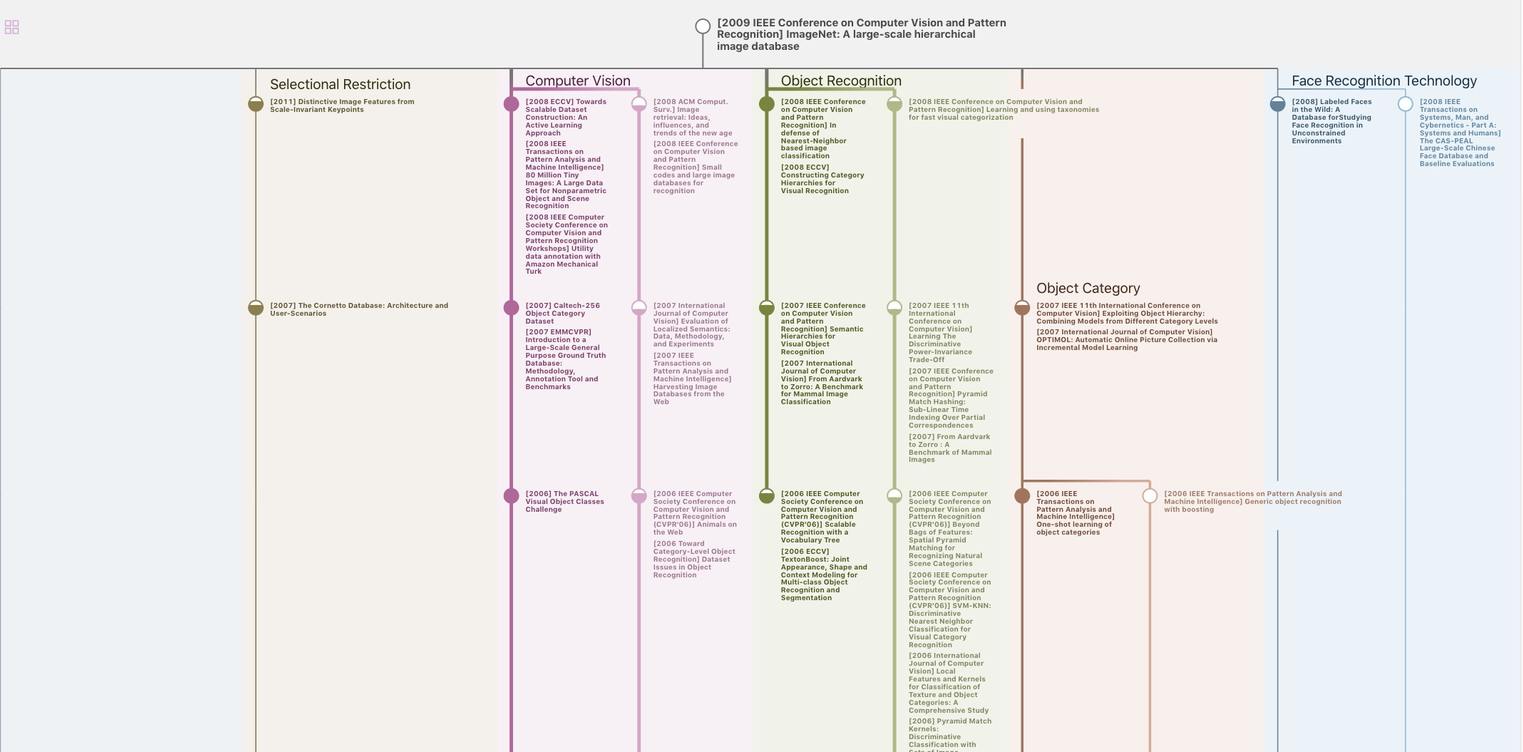
生成溯源树,研究论文发展脉络
Chat Paper
正在生成论文摘要