MEGCF: Multimodal Entity Graph Collaborative Filtering for Personalized Recommendation
ACM Transactions on Information Systems(2023)
摘要
In most E-commerce platforms, whether the displayed items trigger the user's interest largely depends on their most eye-catching multimodal content. Consequently, increasing efforts focus on modeling multimodal user preference, and the pressing paradigm is to incorporate complete multimodal deep features of the items into the recommendation module. However, the existing studies ignore the mismatch problem between multimodal feature extraction (MFE) and user interest modeling (UIM). That is, MFE and UIM have different emphases. Specifically, MFE is migrated from and adapted to upstream tasks such as image classification. In addition, it is mainly a content-oriented and non-personalized process, while UIM, with its greater focus on understanding user interaction, is essentially a user-oriented and personalized process. Therefore, the direct incorporation of MFE into UIM for purely user-oriented tasks, tends to introduce a large number of preference-independent multimodal noise and contaminate the embedding representations in UIM. This paper aims at solving the mismatch problem between MFE and UIM, so as to generate high-quality embedding representations and better model multimodal user preferences. Towards this end, we develop a novel model, multimodal entity graph collaborative filtering, short for MEGCF. The UIM of the proposed model captures the semantic correlation between interactions and the features obtained from MFE, thus making a better match between MFE and UIM. More precisely, semantic-rich entities are first extracted from the multimodal data, since they are more relevant to user preferences than other multimodal information. These entities are then integrated into the user-item interaction graph. Afterwards, a symmetric linear Graph Convolution Network (GCN) module is constructed to perform message propagation over the graph, in order to capture both high-order semantic correlation and collaborative filtering signals. Finally, the sentiment information from the review data are used to fine-grainedly weight neighbor aggregation in the GCN, as it reflects the overall quality of the items, and therefore it is an important modality information related to user preferences. Extensive experiments demonstrate the effectiveness and rationality of MEGCF.(1)
更多查看译文
关键词
Collaborative filtering,semantic correlation,multimodal user preference,multimodal semantic entity,collaborative signal,graph convolutional network,sentiment analysis
AI 理解论文
溯源树
样例
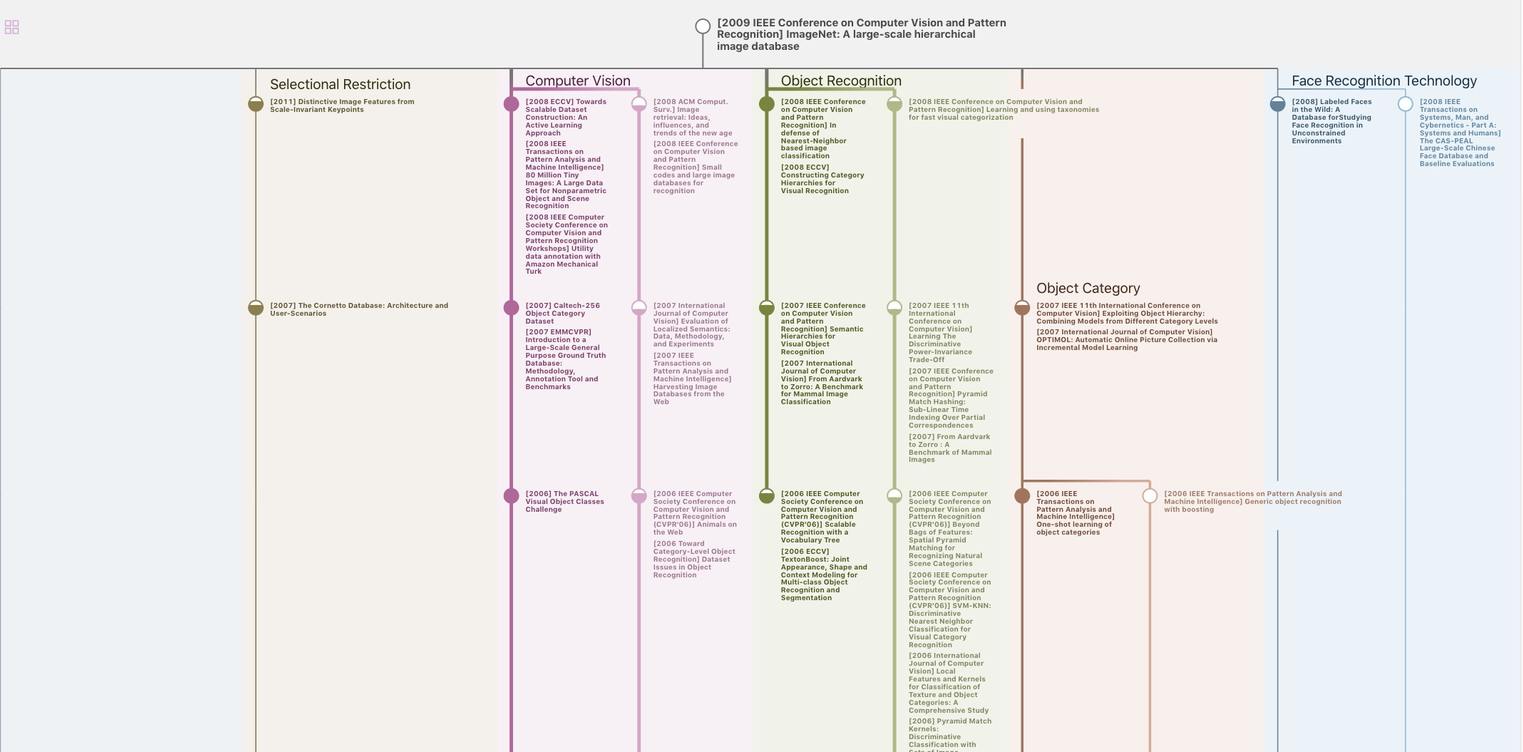
生成溯源树,研究论文发展脉络
Chat Paper
正在生成论文摘要