Autoencoder for Network Anomaly Detection
2022 IEEE International Symposium on Measurements & Networking (M&N)(2022)
摘要
In modern network and telecommunication systems, hundreds of thousands of nodes are interconnected by telecommunication links to exchange information between nodes. The complexity of the system and the stringent requirements on service level agreement makes it necessary to monitor network performance intelligently and enable preventative measures to ensure network performance. Anomaly detection - the task of identifying events that deviate from the normal behavior - continues to be an important task. However, techniques traditionally employed by industry on real-world data - DBSCAN and MAD - have severe limitations, such as the need to manually tune and calibrate the algorithms frequently and limited capacity to capture past history in the model. Lately, there has been much progression in applying machine learning techniques, specifically autoencoders to the problem of AD. However, thus far, few of these techniques have been tested for use in scenarios involving multivariate time-series data that would be faced by telecommunication companies. We propose a novel auto encoder based deep learning framework called ERICA including a new pipeline to address these shortcomings. Our approach has been demonstrated to achieve better performance (an increase in F-score by over 10%) and significantly enhance the scalability.
更多查看译文
关键词
anomaly detection,machine learning,deep learning
AI 理解论文
溯源树
样例
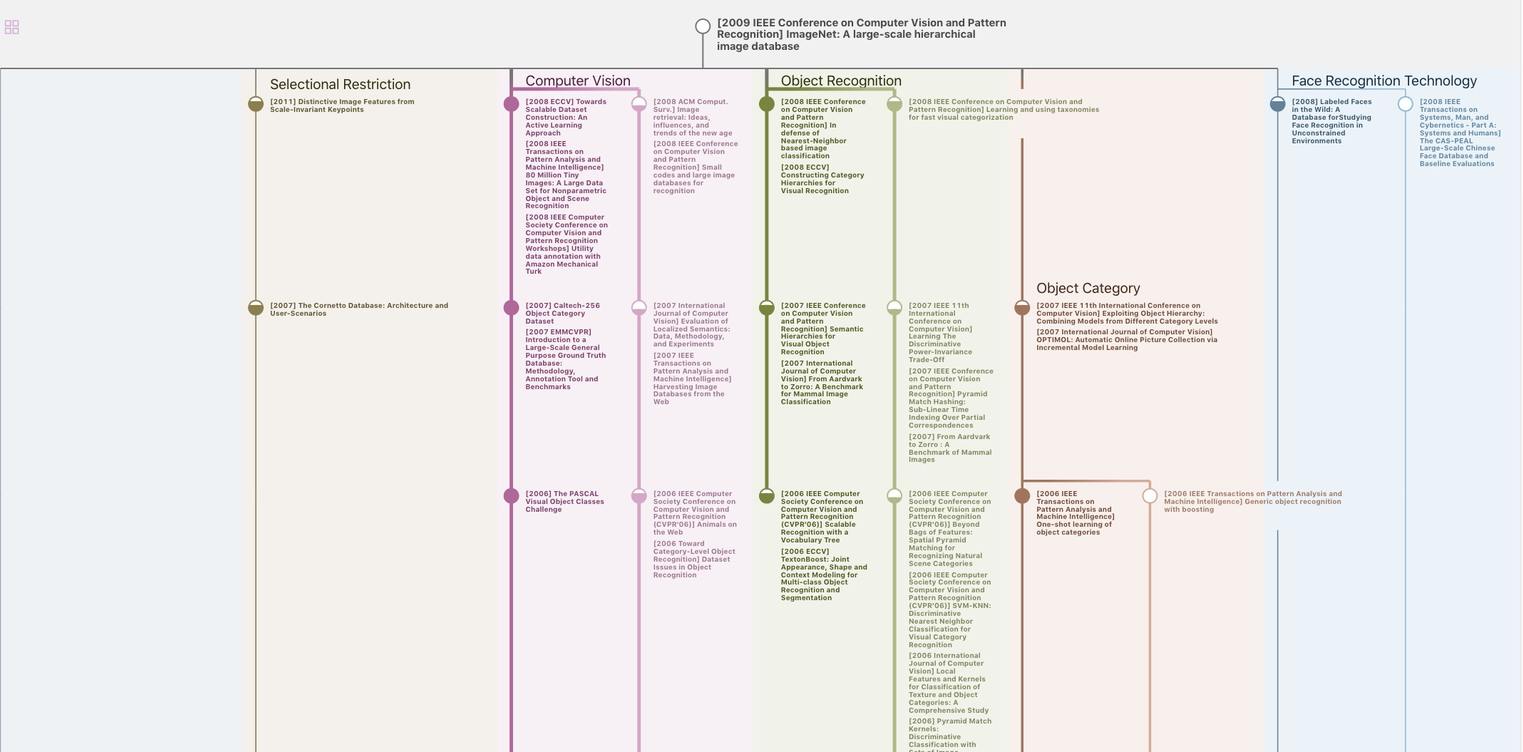
生成溯源树,研究论文发展脉络
Chat Paper
正在生成论文摘要