Combined Machine Learning Techniques For Characteristics Classification and Threshold Voltage Extraction of Transistors
2022 IEEE 34th International Conference on Microelectronic Test Structures (ICMTS)(2022)
摘要
We present an autonomous 2-step Machine Learning based approach for characteristics classification and key parameter extraction for transistors. The first step is a multi-model ensemble, composed of Machine Learning, more particularly a Convolutional Neural Network (CNN) approach to enable fast classification of transistor characteristics. The second step is another CNN model to extract the threshold voltage parameters that enable us to measure the performance of ultra-scaled MOSFETs. Our CNN-based classifier has demonstrated accuracy above 90% with an execution time significantly faster than that of the current human expert-based methods while our V
th
extractor has demonstrated less than 8mV error rate in the filtered dataset that comes from the classifier. The proposed techniques which do not incorporate hard-coded domain knowledge, and are also tested with input data coming from 16nm-node FinFET technology and evaluated by a consensus of experts to prove the universality of the models using the same parameters.
更多查看译文
关键词
Semiconductors,Transistor defect,Machine Learning,Convolutional Neural Network,Threshold Voltage Extraction
AI 理解论文
溯源树
样例
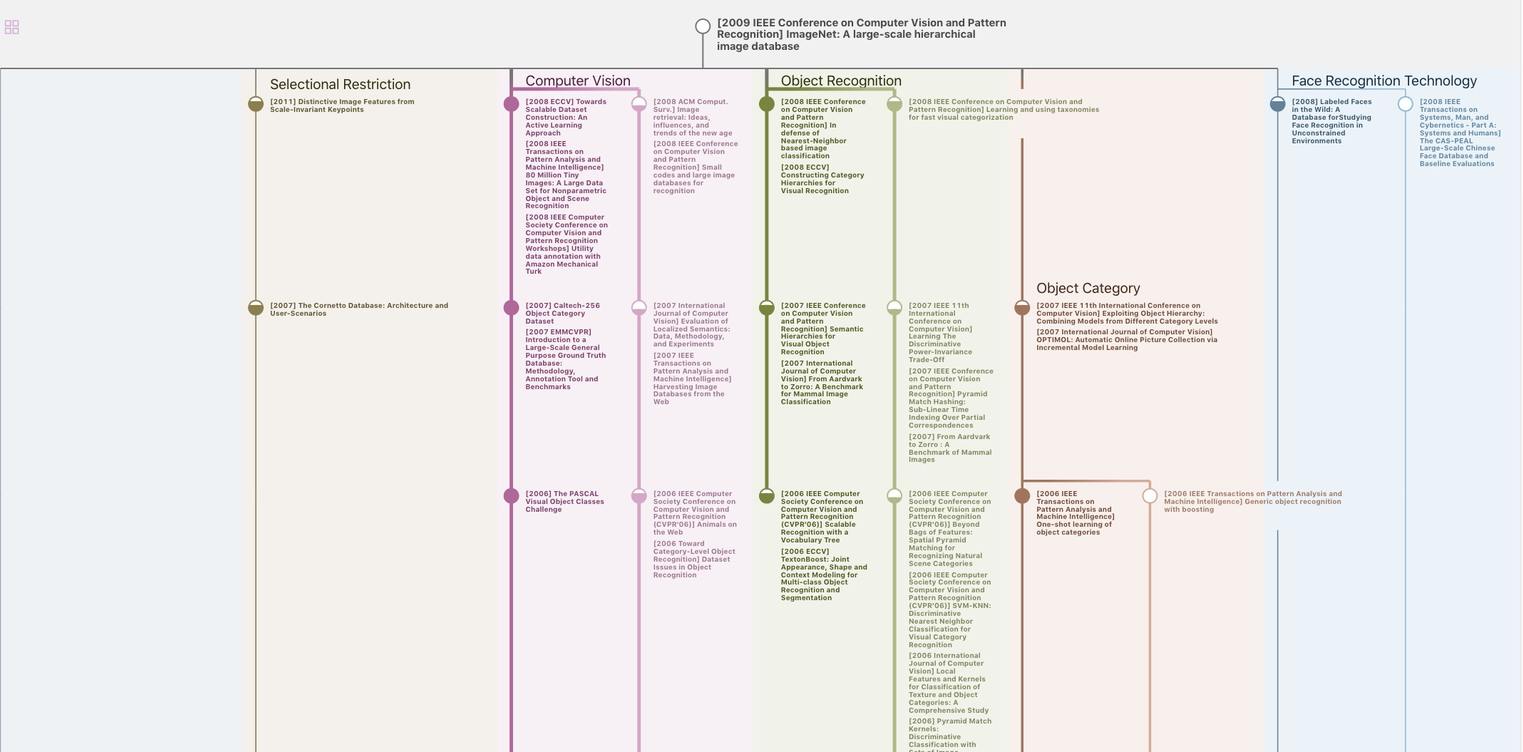
生成溯源树,研究论文发展脉络
Chat Paper
正在生成论文摘要