Evaluation of Probability Distribution Distance Metrics in Traffic Flow Outlier Detection
2022 23rd IEEE International Conference on Mobile Data Management (MDM)(2022)
摘要
Recent approaches have proven the effectiveness of local outlier factor-based outlier detection when applied over traffic flow probability distributions. However, these approaches used distance metrics based on the Bhattacharyya coefficient when calculating probability distribution similarity. Consequently, the limited expressiveness of the Bhattacharyya coefficient restricted the accuracy of the methods. The crucial deficiency of the Bhattacharyya distance metric is its inability to compare distributions with non-overlapping sample spaces over the domain of natural numbers. Traffic flow intensity varies greatly, which results in numerous non-overlapping sample spaces, rendering metrics based on the Bhattacharyya coefficient inappropriate. In this work, we address this issue by exploring alternative distance metrics and showing their applicability in a massive real-life traffic flow data set from 26 vital intersections in The Hague. The results on these data collected from 272 sensors for more than two years show various advantages of the Earth Mover's distance both in effectiveness and efficiency.
更多查看译文
关键词
Traffic Flow,Probability Distribution Distance Metric,Outlier Detection,Earth Mover's Distance,Kullback-Leibler divergence,Hellinger Distance,Bhattacharyya Distance
AI 理解论文
溯源树
样例
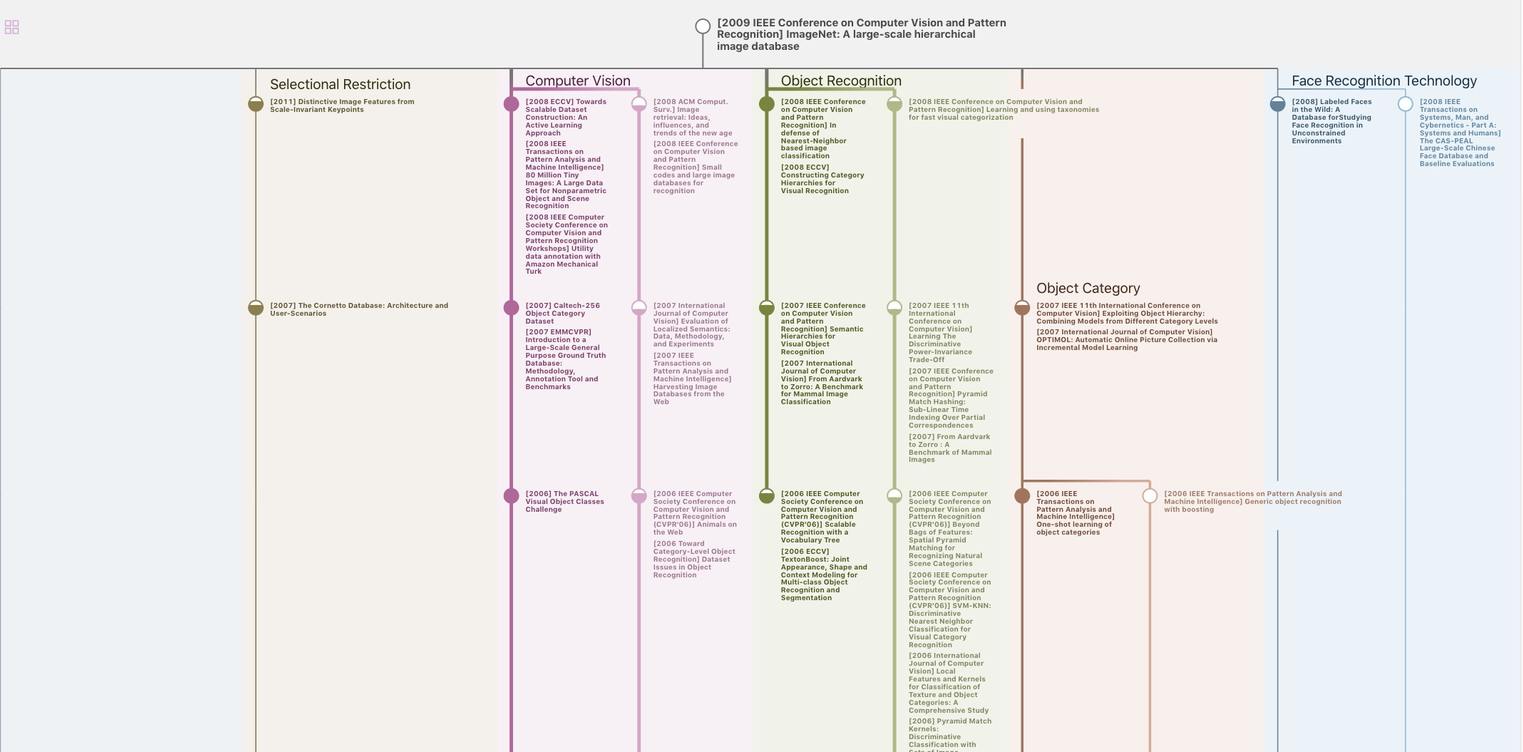
生成溯源树,研究论文发展脉络
Chat Paper
正在生成论文摘要