Coronary artery stenosis and vulnerable plaque quantification on CCTA by deep learning methods
European Heart Journal(2022)
摘要
Abstract Introduction Coronary computed tomography angiography (CCTA) has emerged as a reliable non-invasive modality to assess coronary artery stenosis (CAS) severity and vulnerable plaque (VP). However, comprehensive CCTA assessment, especially VP, is time-consuming and dependent on reader expertise, limiting CCTA's true potential. Purpose In this study, we aim to develop and validate a deep learning (DL) based system capable of evaluating CAS severity and characterising VP on CCTA. Methods A DL system was trained to assess CAS severity on 3909 expert annotated vessels. A subset of 824 vessels was used to train the model to assess for the presence of VP. The model was based on a 2D U-Net and 3D convolutional neural network architecture. The system automatically performed vessel tracking and segmentation to quantify stenosis severity and characterise the presence of VP. CAS severity was categorised as 0%, 1–49% and ≥50%. VP was defined as: low attenuation plaque (LAP; ≤30 Hounsfield units), positive remodelling (PR; ≥10% diameter) and spotty calcification (SC; <3mm). The model was then tested on 1435 vessels for CAS (mean calcium score 197±502) and a subset of 365 vessels for VP (mean calcium score 419±551), and its diagnostic performance compared with expert readers. Results The CAS testing data had a prevalence of 75% (1080/1435), 18% (257/1435) and 6.8% (98/1435) for 0%, 1–49% and ≥50% stenosis, respectively. VP was present in 20% (72/365) in the respective dataset with 44% (32/72) LAP, 19% (14/72) PR and 36% (26/72) SC. Average analysis time for CAS severity and VP was 3.7±2.0s and 3.5±1.8s, respectively. Diagnostic performance of our system is summarised in Tables 1 (CAS severity) and 2 (VP characteristics). Conclusions We developed an DL based system capable of rapidly evaluating CAS severity and characterising VP on CCTA. Our system demonstrated high specificity and accuracy for both CAS severity and VP quantification when compared with expert readers. Funding Acknowledgement Type of funding sources: None.
更多查看译文
关键词
vulnerable plaque quantification,coronary artery stenosis,coronary artery,deep learning methods,ccta
AI 理解论文
溯源树
样例
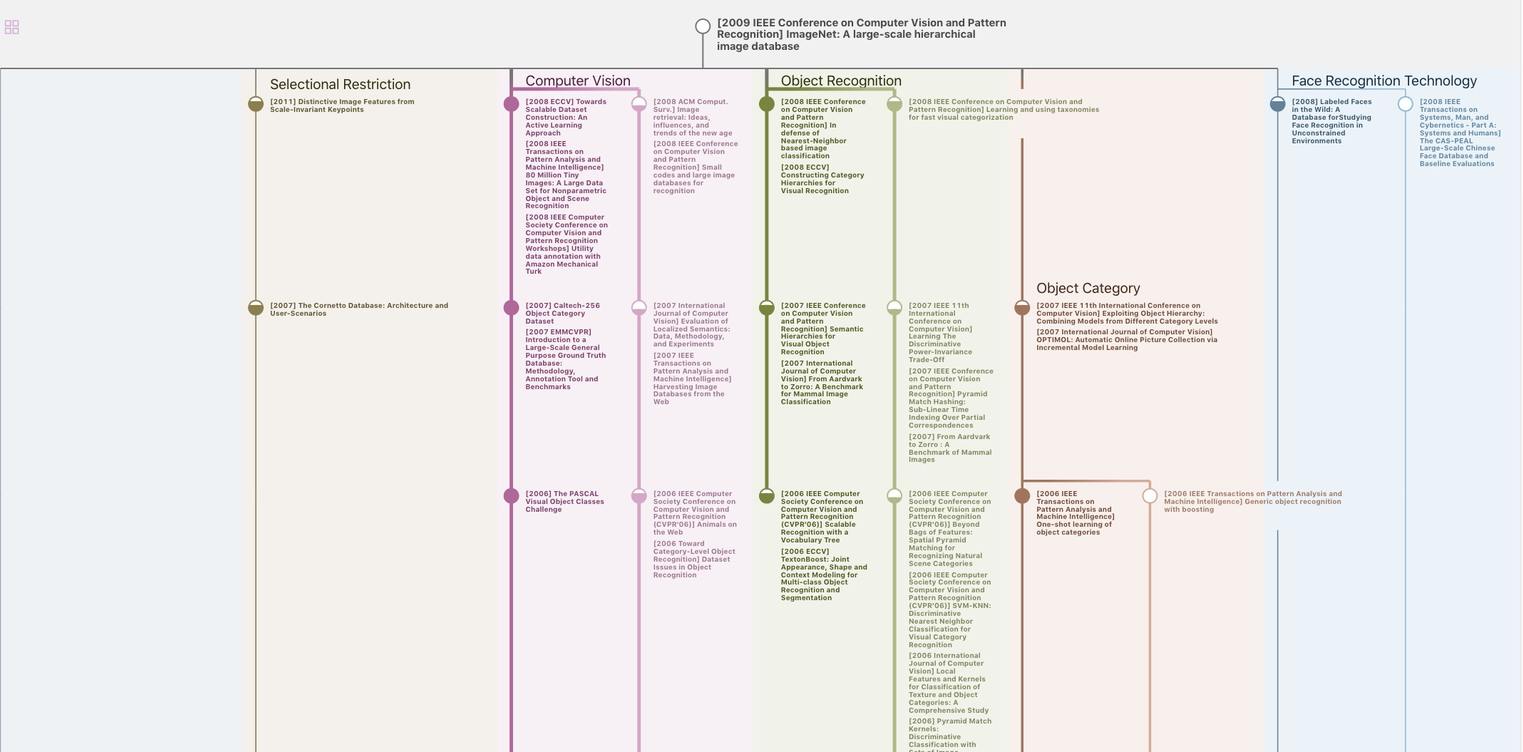
生成溯源树,研究论文发展脉络
Chat Paper
正在生成论文摘要