Feature Interaction Prototypical Network for Few-Shot Fine-Grained Image Classification
2022 4th International Conference on Natural Language Processing (ICNLP)(2022)
摘要
In recent years, although the task of fine-grained image classification has achieved remarkable results, these algorithms need to be trained on large datasets in order to obtain good results, otherwise it is easy to cause model overfitting and reduce the effect. However, fine-grained image datasets need to distinguish different subcategories under the same category. It is difficult to collect and label fine-grained images in practical application, so it is not easy to obtain a large number of good training data. Few-shot learning provides a solution for this. However, the traditional few-shot learning algorithm has limited feature extraction ability, and it is difficult to effectively classify fine-grained images with large intra class differences and small inter class differences. To this end, this paper utilizes the idea of model fine-tuning to extract features using a pre-trained model. In addition, the SimAM non-parametric attention mechanism is introduced to enhance features and filter backgrounds while reducing model parameters. Secondly, feature maps of different convolutional layers are fused using hierarchical bilinear pooling (HBP), thus effectively utilizing information from different convolutional layers to further enhance feature representation. Finally, the classification is completed by calculating the distance between the embedding vectors of the test samples and the well-distributed prototypes generated using the fused features. Experiments show that this algorithm performs well on few-shot fine-grained image classification tasks and achieves excellent performance on traditional few-shot image classification.
更多查看译文
关键词
Few-shot learning,Fine-grained image classification,Hierarchical Bilinear Pooling,Non-parametric attention mechanism
AI 理解论文
溯源树
样例
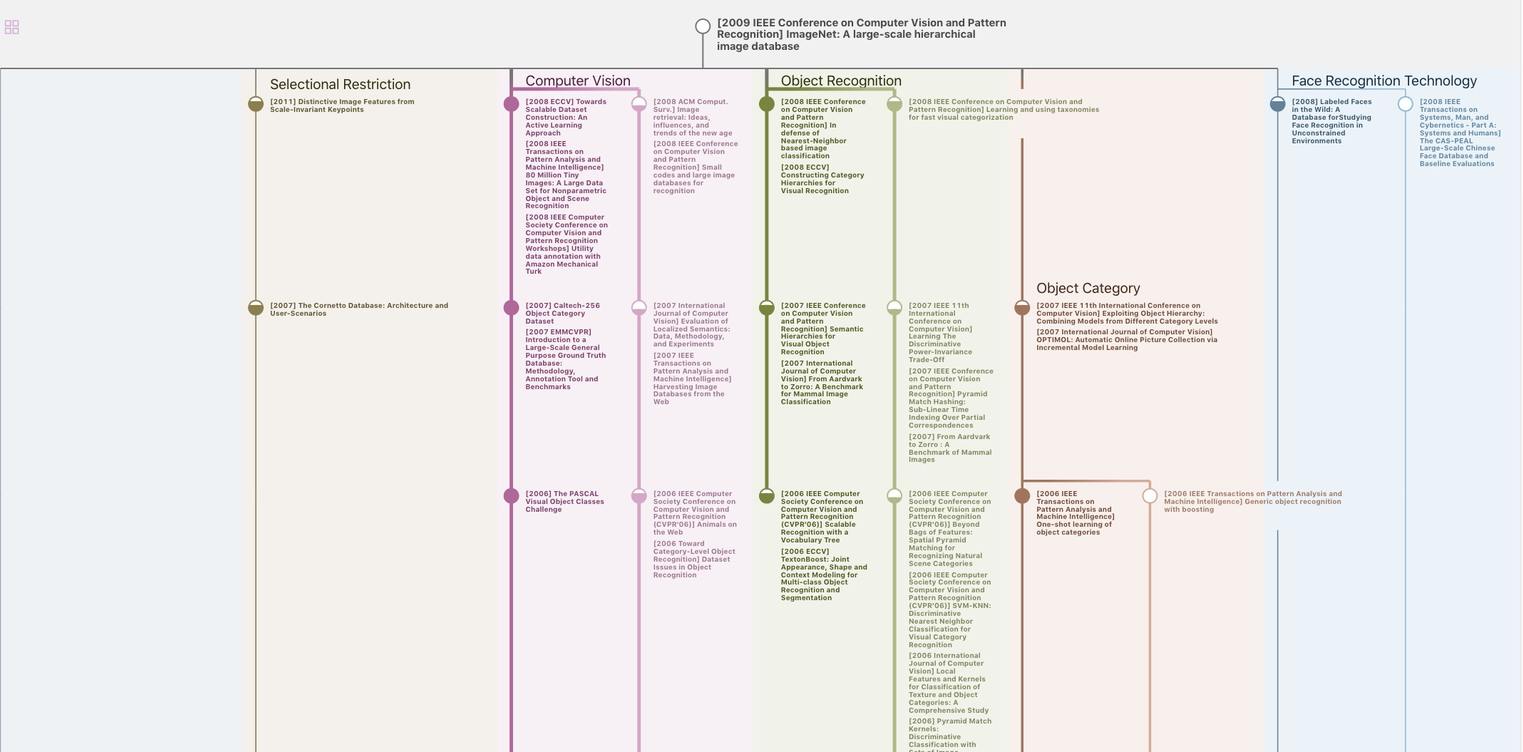
生成溯源树,研究论文发展脉络
Chat Paper
正在生成论文摘要