Few-Shot Fine-Grained Image Classification: A Survey
2022 4th International Conference on Natural Language Processing (ICNLP)(2022)
摘要
With the development of deep learning, fine-grained image classification task has made remarkable achievements, but it largely depends on a large number of annotated data samples. However, in practical applications, such as public safety, medicine, endangered species and other professional fields, such fine-grained samples with annotations are difficult to obtain. When the samples are insufficient, the traditional deep learning training method is easy to yield the over fitting phenomenon, which greatly limits the applicability of such algorithms. The general few-shot algorithm is not designed for the fine-grained characteristics of the image, so it can not effectively extract the subtle differences in the image, which results in poor performance. Therefore, fine-grained image classification task based on few-shot learning has attracted more and more attentions in recent years. This paper introduces the task of few-shot fine-grained image classification, and summarizes the literatures in this field over the recent years. According to the few-shot learning methods, the existing algorithms are divided into the following three categories: meta learning based methods, multimodal based methods, and data augmentation based methods. In addition, the common datasets and performance evaluation indexes in the field of few-shot fine-grained image classification are summarized. Finally, the technical difficulties and research trends in this field are discussed.
更多查看译文
关键词
deep learning,few-shot learning,fine-grained image classification,computer vision
AI 理解论文
溯源树
样例
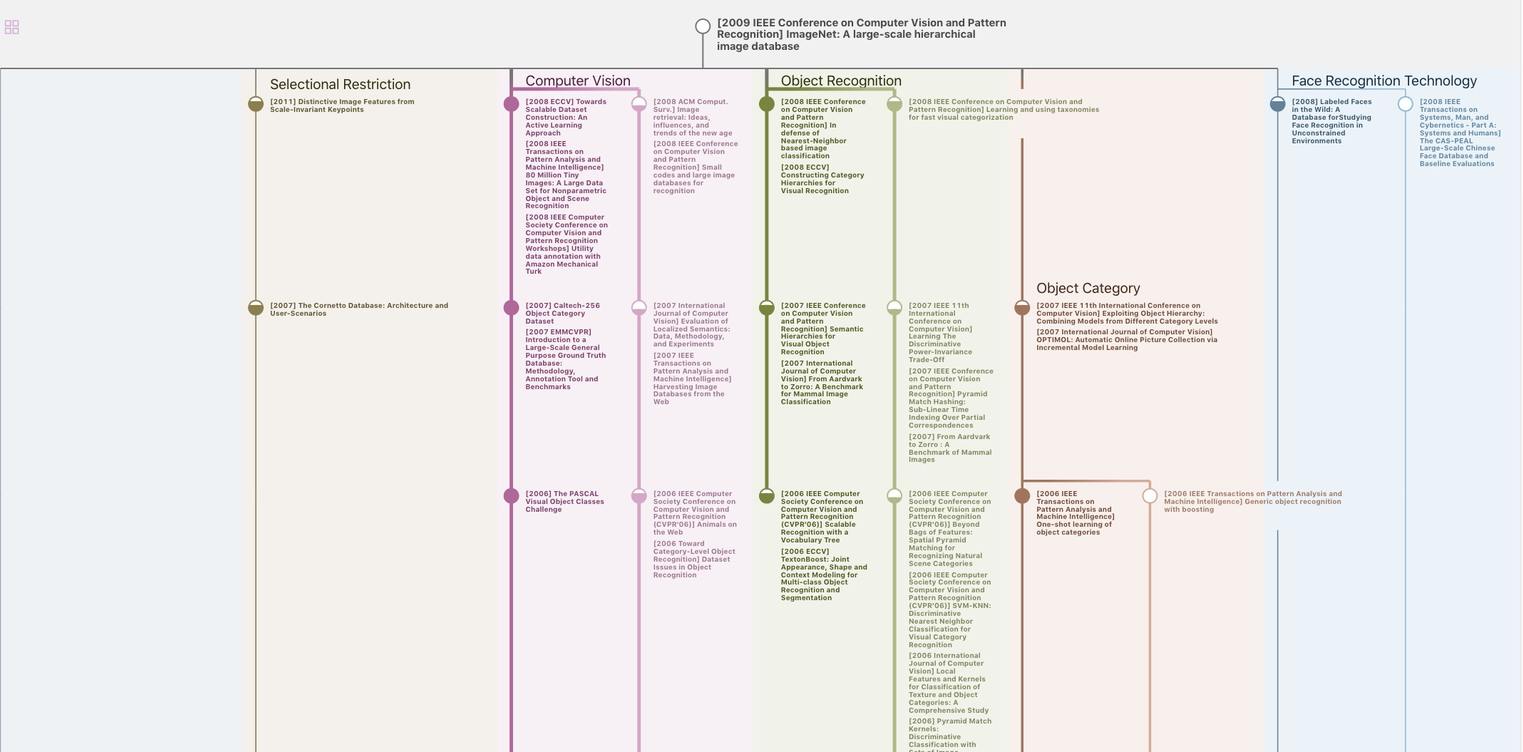
生成溯源树,研究论文发展脉络
Chat Paper
正在生成论文摘要