Network Anomaly Detection based on Traffic Clustering with Group-Entropy Similarity
2022 International Symposium on Networks, Computers and Communications (ISNCC)(2022)
Abstract
Although we may observe heterogeneous traffic appearance on the network backbone, malicious traffic tends to converge with their traffic appearance similarity due to the consistent hostile behaviors of the same anomaly category. Measuring such traffic similarity of host behaviors can help us to detect anomalous traffic from benign traffic. This paper proposes a novel framework for the detection of network intrusion based on traffic similarity measures and clustering. We apply the grouping and DBSCAN method to feature dimensionality reduction so that traffic carrying the same category anomalies is concentrated in the limited amount of clusters, which can be interpreted as the structured significant characteristics of the corresponding anomaly category. The derived anomaly cluster characteristics are useful for detecting newly coming traffic in future for its maliciousness. Based on the experiment with the IDS 2018 dataset, our proposed detection procedure can effectively separate the malicious network traffic from background with an accuracy of up to 96%. Our proposed method has apparent benefits for identifying malicious traffic in large-scale network traffic data, and it is a practical intrusion detection method.
MoreTranslated text
Key words
anomaly detection,malicious traffic similarity,conditional entropy
AI Read Science
Must-Reading Tree
Example
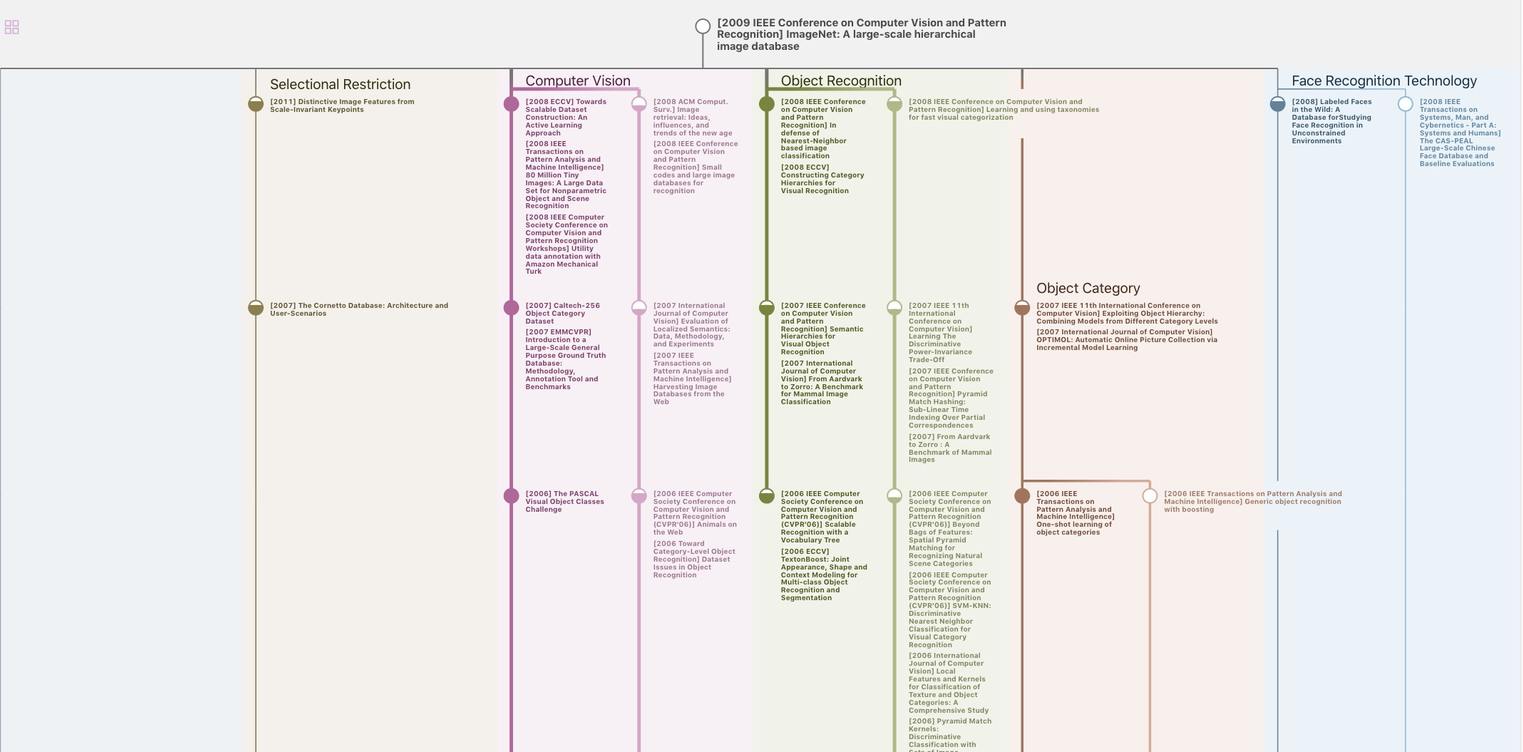
Generate MRT to find the research sequence of this paper
Chat Paper
Summary is being generated by the instructions you defined