Addressing Scalability Issues in Semantics-Driven Recommender Systems
WI/IAT(2021)
摘要
Content-based semantics-driven recommender systems are often used in the small-scale news recommendation domain. These recommender systems improve over TF-IDF by taking into account (domain) semantics through semantic lexicons or domain ontologies. Our work explores the application of such recommender systems to other domains, using the case of large-scale movie recommendations. We propose new methods to extract semantic features from various item descriptions, and for scaling up the semantics-driven approach with pre-computation of the cosine similarities and gradient learning of the model. The results of the study on a large-scale dataset of user ratings demonstrate that semantics-driven recommenders can be extended to more complex domains and outperform TF-IDF on ROC, PR, F1, and Kappa metrics.
更多查看译文
关键词
semantics-driven recommendation, scalability, ontology
AI 理解论文
溯源树
样例
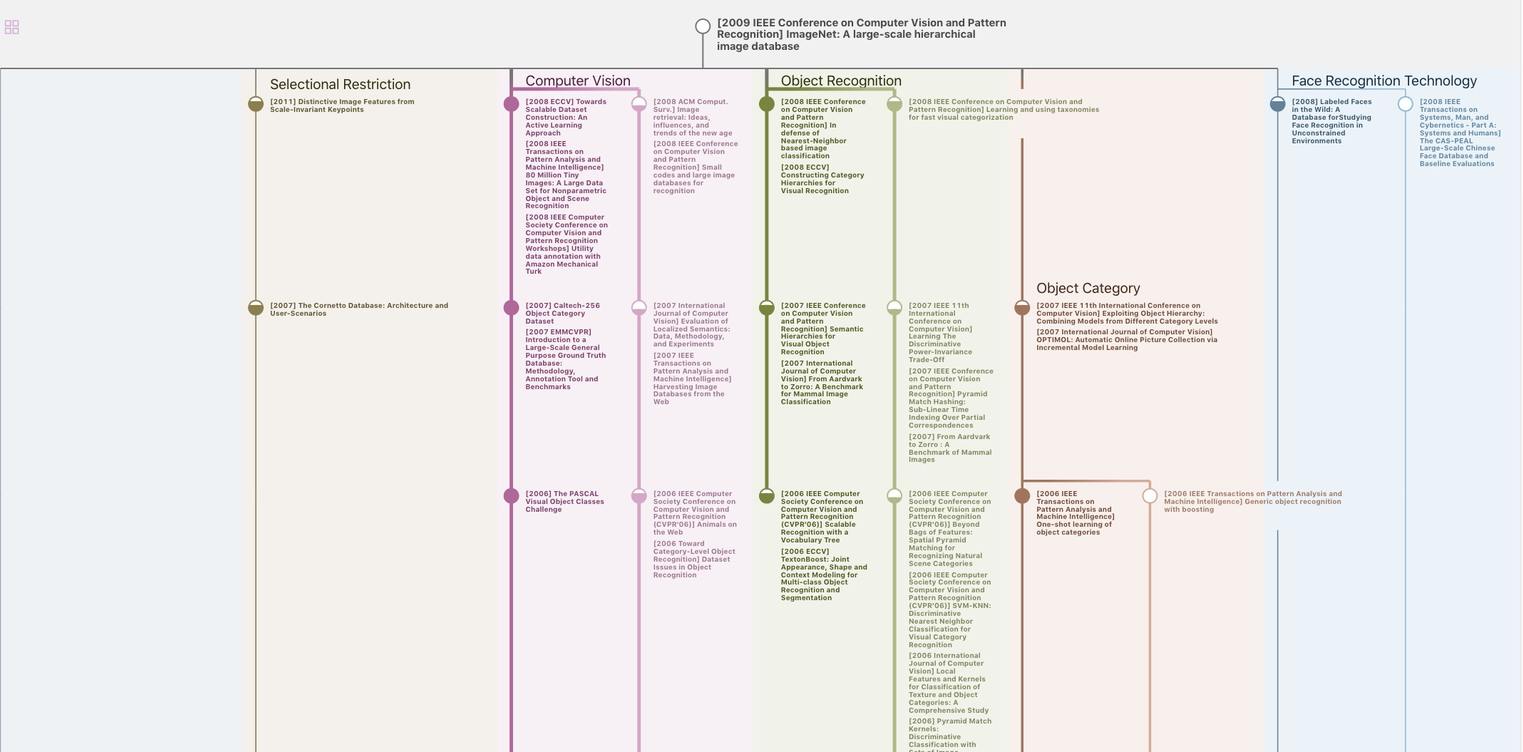
生成溯源树,研究论文发展脉络
Chat Paper
正在生成论文摘要