Left atrial overload detection in ECG using frequency domain features with convolutional neural network
European Heart Journal(2022)
摘要
Abstract Background/Introduction Volume and pressure alterations in the left atrium (LA) are utilized as important biomarkers due to their association with numerous cardiac pathologies. Because of the anatomical peculiarities of the LA, echocardiographic evaluation is quite challenging. There are many conducted studies to determine LA hemodynamic biomarkers using ECG signals, however, the low specificity has been seen as a common problem. Purpose Within the scope of this study, we aimed to develop a fully automatic artificial intelligence algorithm. Therefore, the frequency domain properties of the ECG signal were examined by wavelet transform and deep learning algorithm to detect the left atrial overload (LAO) from the ECG signal. We also compared the effectiveness of lead selection (Lead II and V1 Lead) to detect LAO. Methods Physionet-PTB-XL database was used for ECG inputs. 10 s ECG waveform of 403 healthy people and 352 LAO patients used for feature extraction after carefully filtering. Each signal was segmented to a 1.5 s epoch and in total 4530 ECG was used for training (70%) and test (30%) of deep learning algorithms. Two different deep learning models were developed for the automatic detection of LAO. In the first approach (1D convolutional neural network, 1D-CNN) segmented raw ECG was used, and in the second approach (2D convolutional neural network, 2D-CNN) each segmented ECG was transformed to scalograms with continuous wavelets transform. All models were tested for both leads. Results Our results show that frequency-domain features are highly capable to detect LAO compared to time-domain features and either Lead II and V1 Lead can be used for the detection of LAO. The success rate of 1D-CNN is 72% for Lead-II and 75% for V1 Lead while the success rate of 2D-CNN is 92% for both leads. Conclusions The most important limitation in the diagnosis of LAO by ECG is the low specificity. In this study, a model that eliminates this limitation has been developed by using the frequency characteristics of the ECG and artificial intelligence algorithms and tested with a large population. Considering its high success rate, 2D-CNN model has the potential to help the clinician by detecting the pathology with a cheaper, operator-independent method, and a short-term measurement. Funding Acknowledgement Type of funding sources: None.
更多查看译文
关键词
atrial overload detection,ecg,convolutional neural network,frequency domain features
AI 理解论文
溯源树
样例
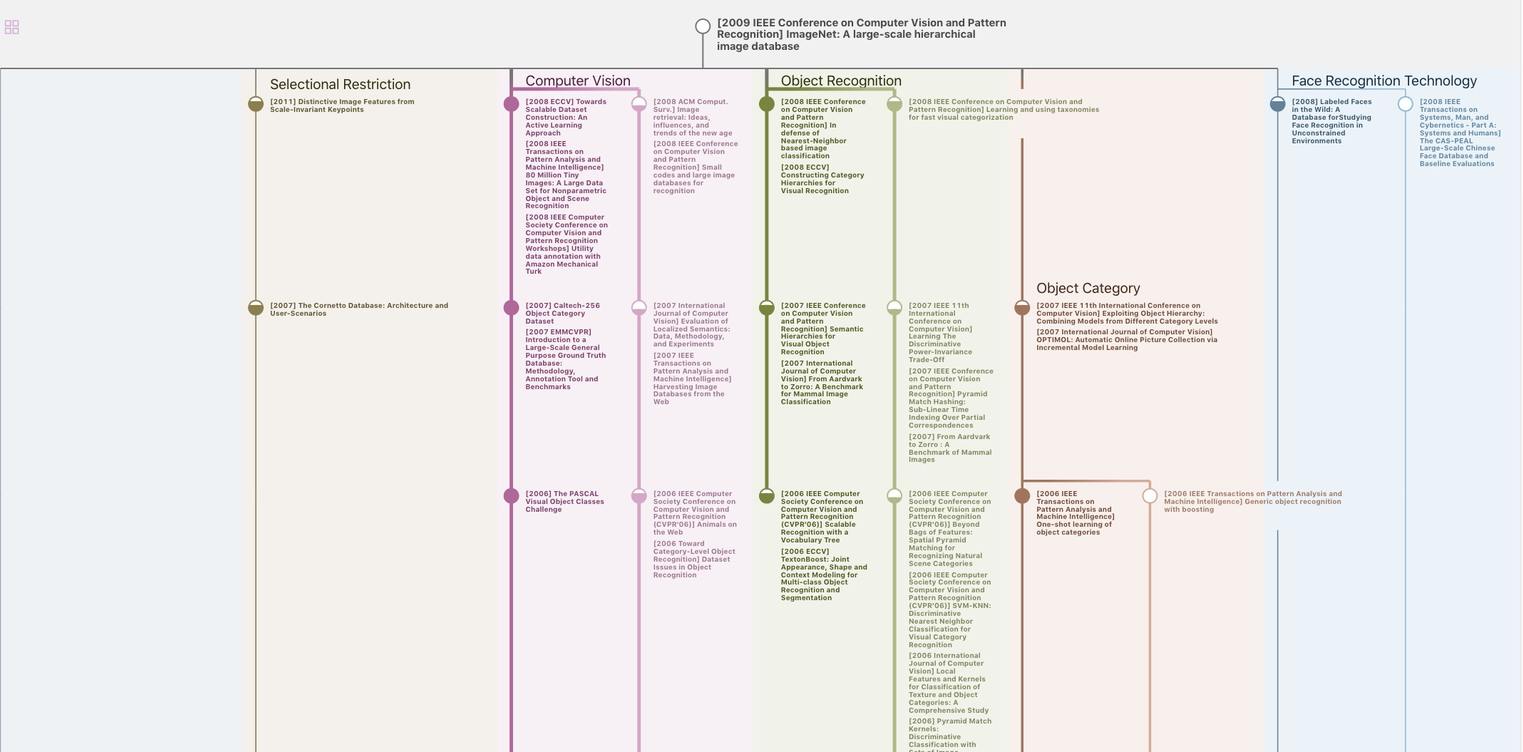
生成溯源树,研究论文发展脉络
Chat Paper
正在生成论文摘要