Prediction of atrial fibrillation in patients with embolic stroke with undetermined source using electrocardiogram deep learning algorithm and clinical risk factors
European Heart Journal(2022)
Abstract
Abstract Background Combining the artificial intelligence algorithm with the known clinical risk factors may provide enhanced accuracy for prediction of the hidden atrial fibrillation (AF) in patients with embolic stroke with undetermined source (ESUS). Purpose We aimed to develop enhanced prediction models for AF with deep learning algorithm (DLA) and clinical predictors in patients with ESUS. The DLA was created to identify the patients with paroxysmal AF based on their electrocardiograms (ECG) during sinus rhythm. Methods We analyzed the 221 patients who underwent insertable cardiac monitor (ICM) for AF detection following ESUS. The DLA was previously developed with sinus rhythm ECGs of 10,605 paroxysmal AF patients and 50,522 non-AF patients. The convolutional neural network was used for the DLA. The primary endpoint was defined as any AF episode lasting over 5 min by ICM. The atrial ectopic burden (AEB) was calculated as the percentage of the number of conducted QRS by atrial ectopy on Holter monitoring. Results AF (≥5 min) was detected by ICM in 32 patients (14.5%) during follow-up period of 15.1±8.6 months. AF patients had higher AEB (0.199% vs 0.023%, p<0.001), larger left atrial diameters (LAD, 41.2 mm vs 35.7 mm, p<0.001), and larger left atrial volume index (LAVI, 46.4 ml/m2 vs 32.3 ml/m2, p<0.001) than those without AF. The means of calculated probabilities of AF by DLA were higher in patients with AF than those without AF (63.8% vs 40.2%, p<0.001). In the receiver operating characteristic curve analysis, the areas under the curve (AUC) were the highest in DLA (0.824) followed by AEB (0.784), LAVI (0.780), and LAD (0.768). The multivariable model with AEB, LAVI, and DLA demonstrated excellent prediction accuracy for paroxysmal AF (AUC: 0.902, Figure 1) Conclusions In patients with ESUS, the DLA outperformed other clinical risk factors for prediction of AF. Combining DLA with AEB, LAD and LAVI could is a potential useful tool to predict AF in ESUS patients. Funding Acknowledgement Type of funding sources: None.
MoreTranslated text
AI Read Science
Must-Reading Tree
Example
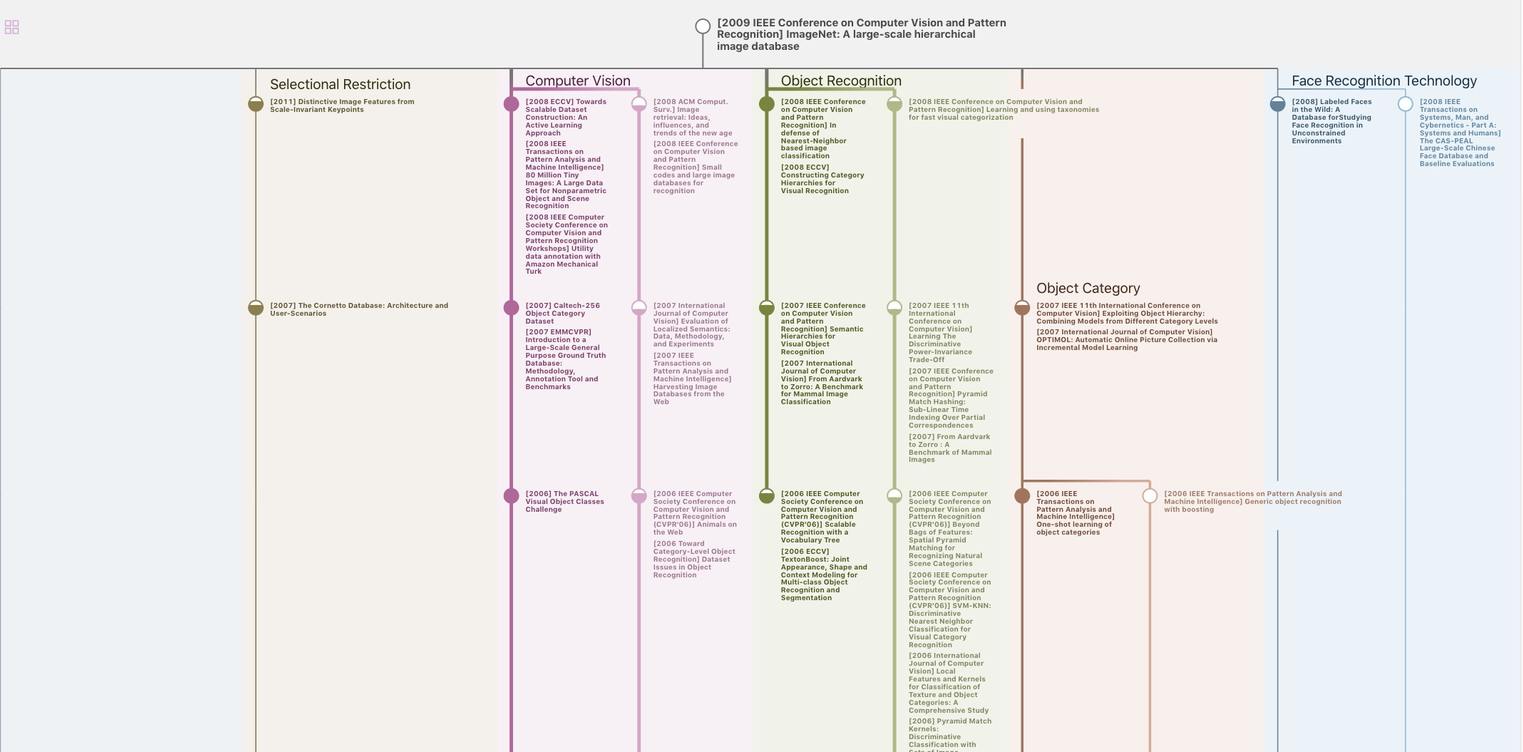
Generate MRT to find the research sequence of this paper
Chat Paper
Summary is being generated by the instructions you defined