LRP-based Policy Pruning and Distillation of Reinforcement Learning Agents for Embedded Systems
2022 IEEE 25th International Symposium On Real-Time Distributed Computing (ISORC)(2022)
Abstract
Reinforcement Learning (RL) is an effective approach to developing control policies by maximizing the agent’s reward. Deep Reinforcement Learning (DRL) uses Deep Neural Networks (DNNs) for function approximation in RL, and has achieved tremendous success in recent years. Large DNNs often incur significant memory size and computational overheads, which greatly impedes their deployment into resource-constrained embedded systems. For deployment of a trained RL agent on embedded systems, it is necessary to compress the Policy Network of the RL agent to improve its memory and computation efficiency. In this paper, we perform model compression of the Policy Network of an RL agent by leveraging the relevance scores computed by Layer-wise Relevance Propagation (LRP), a technique for Explainable AI (XAI), to rank and prune the convolutional filters in the Policy Network, combined with fine-tuning with Policy Distillation. Performance evaluation based on several Atari games indicates that our proposed approach is effective in reducing model size and inference time of RL agents.
MoreTranslated text
Key words
Reinforcement Learning,Knowledge Distillation,Policy Distillation,embedded systems
AI Read Science
Must-Reading Tree
Example
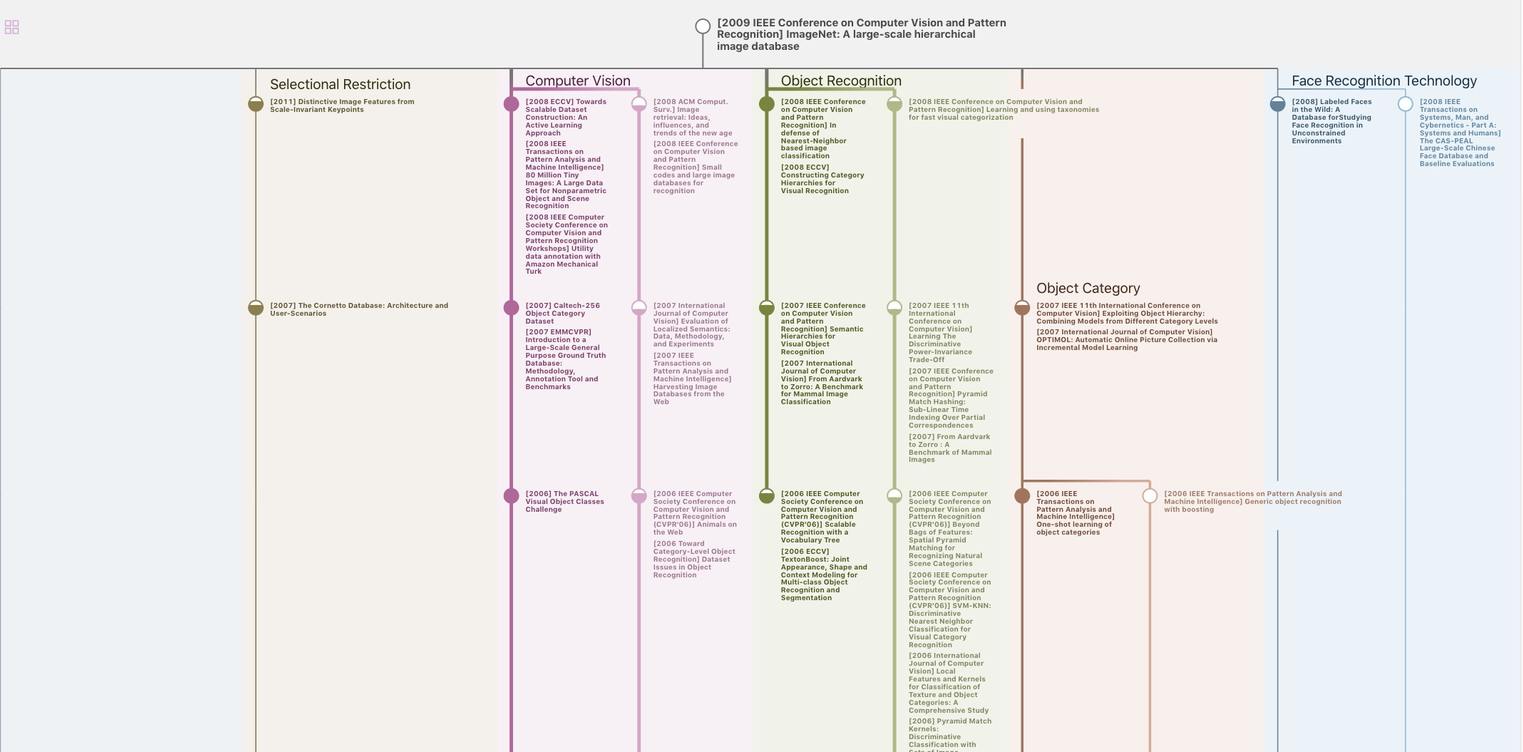
Generate MRT to find the research sequence of this paper
Chat Paper
Summary is being generated by the instructions you defined