A Survey on Causal Discovery: Theory and Practice
International Journal of Approximate Reasoning(2022)
摘要
Understanding the laws that govern a phenomenon is the core of scientific progress. This is especially true when the goal is to model the interplay between different aspects in a causal fashion. Indeed, causal inference itself is specifically designed to quantify the underlying relationships that connect a cause to its effect. Causal discovery is a branch of the broader field of causality in which causal graphs are recovered from data (whenever possible), enabling the identification and estimation of causal effects. In this paper, we explore recent advancements in causal discovery in a unified manner, provide a consistent overview of existing algorithms developed under different settings, report useful tools and data, present real-world applications to understand why and how these methods can be fruitfully exploited.
更多查看译文
关键词
Causality,Causal models,Causal discovery,Structural learning
AI 理解论文
溯源树
样例
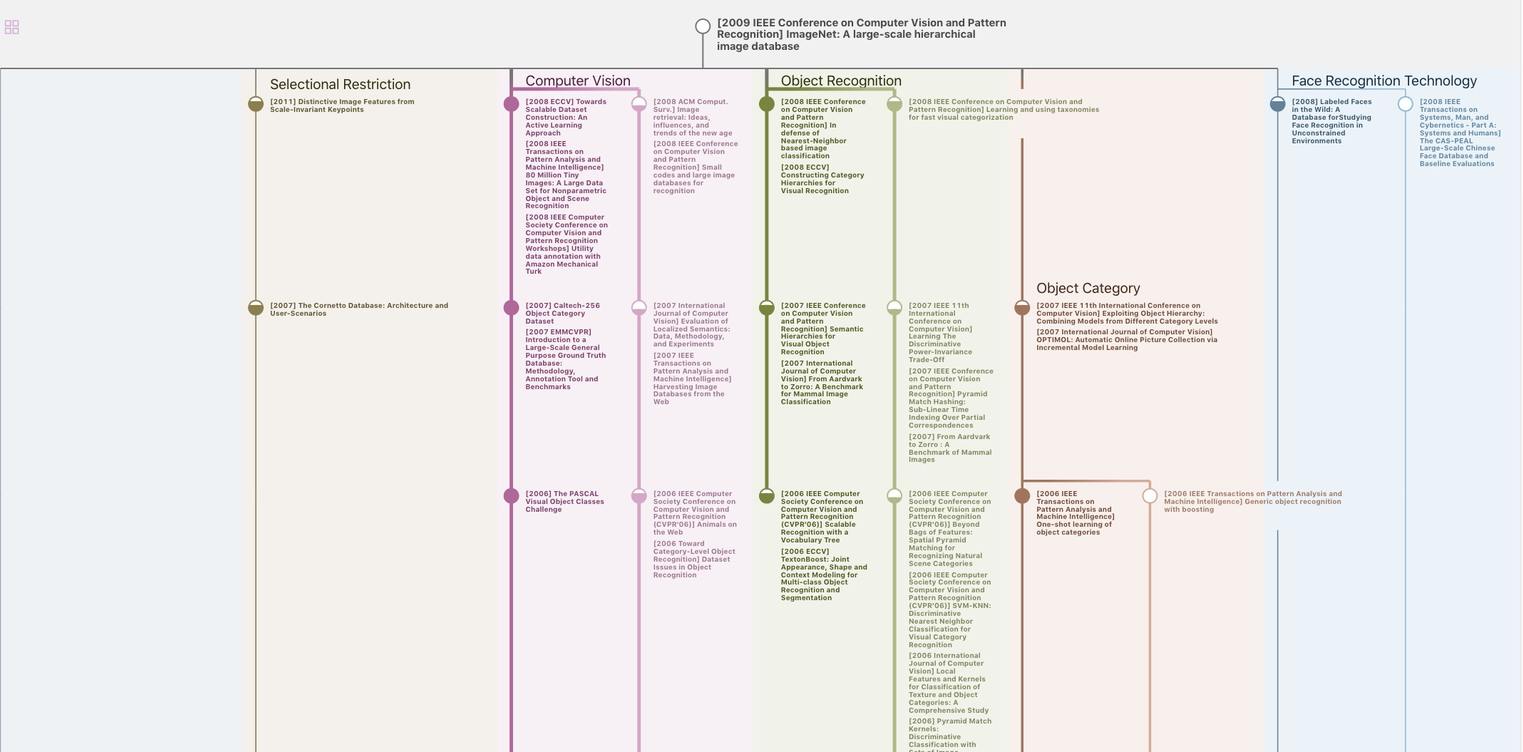
生成溯源树,研究论文发展脉络
Chat Paper
正在生成论文摘要