Constraint-Induced Symmetric Nonnegative Matrix Factorization for Accurate Community Detection
Information Fusion(2023)
摘要
As a fundamental characteristic of an undirected network, community reveals its networking organization and functional mechanisms, making community detection be a highly-interesting issue in network representation learning. With great interpretability, a symmetric and nonnegative matrix factorization (SNMF)-based approach is frequently adopted to tackle this issue. However, it only adopts a unique feature matrix for describing the symmetry of an undirected network, which unfortunately results in a reduced feature space that evidently impairs its representation learning ability. Motivated by this discovery, this paper proposes a novel Constraint-induced Symmetric Nonnegative Matrix Factorization (C-SNMF) model that adopts three-fold ideas: a) Representing a target undirected network with multiple latent feature matrices, thus preserving its representation learning capacity; b) Incorporating a symmetry-regularizer into its objective function, which preserves the symmetry of the learnt low-rank approximation to the adjacency matrix, thereby making the resultant detector precisely illustrate the target network's symmetry; and c) Introducing a graph-regularizer that preserves local invariance of the network's intrinsic geometry into its learning objective, thus making the achieved detector well-aware of community structure within the target network. Note that the regularization coefficients are selected according to the modularity of the learnt community structure on the training data only, thereby greatly improving the achieved model's practical significance for real applications. Experimental results on six real-world networks demonstrate that the proposed C-SNMF model significantly outperforms the benchmarks and state-of-the-art models in achieving highly-accurate community detection results.
更多查看译文
关键词
Undirected Network,Social Network,Information Fusion,Network Representation Learning,Community Detection,Nonnegative Matrix Factorization,Symmetry-regularization,Multiple Constraints
AI 理解论文
溯源树
样例
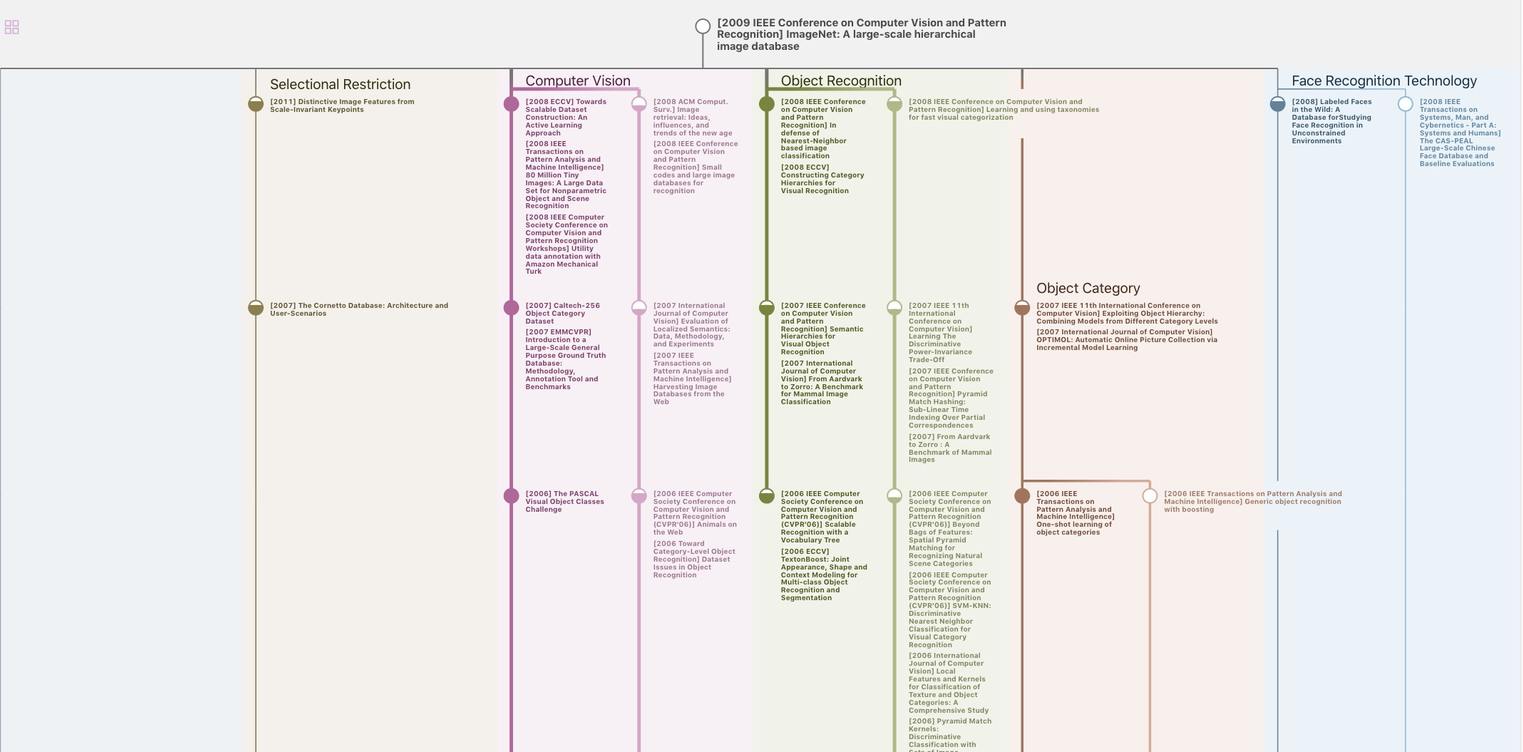
生成溯源树,研究论文发展脉络
Chat Paper
正在生成论文摘要