Synthesis of model predictive control and iterative learning control for topography regulation in additive manufacturing
IFAC-PapersOnLine(2022)
摘要
This paper presents a spatially derived control solution for improving the performance of a high-resolution additive manufacturing (AM) process towards the fabrication of repeatable, thin-film functional devices. In particular, this work addresses challenges in fabrication of high-quality films using AM technology by incorporating knowledge about the interactions between printed layers of material within a control framework for improved repeatability and reliability in the fabrication of multi-layered micro devices using AM technology. We implement an SILC-MPC method that leverages the information from previous layers using spatial iterative learning control (SILC) and projects forward the data from future layers using model predictive control (MPC) to improve the tracking performance of iteration varying AM processes. Simulation results of an AM process termed electrohydrodynamic jet (e-jet) printing demonstrate that an SILC-MPC framework is effective and robust to repetitive and nonrepetitive model uncertainties and outperforms traditional SILC by converging faster to the nominal behavior with a lower tracking error.
更多查看译文
关键词
Model predictive control,Iterative learning control,Micro-Additive Manufacturing
AI 理解论文
溯源树
样例
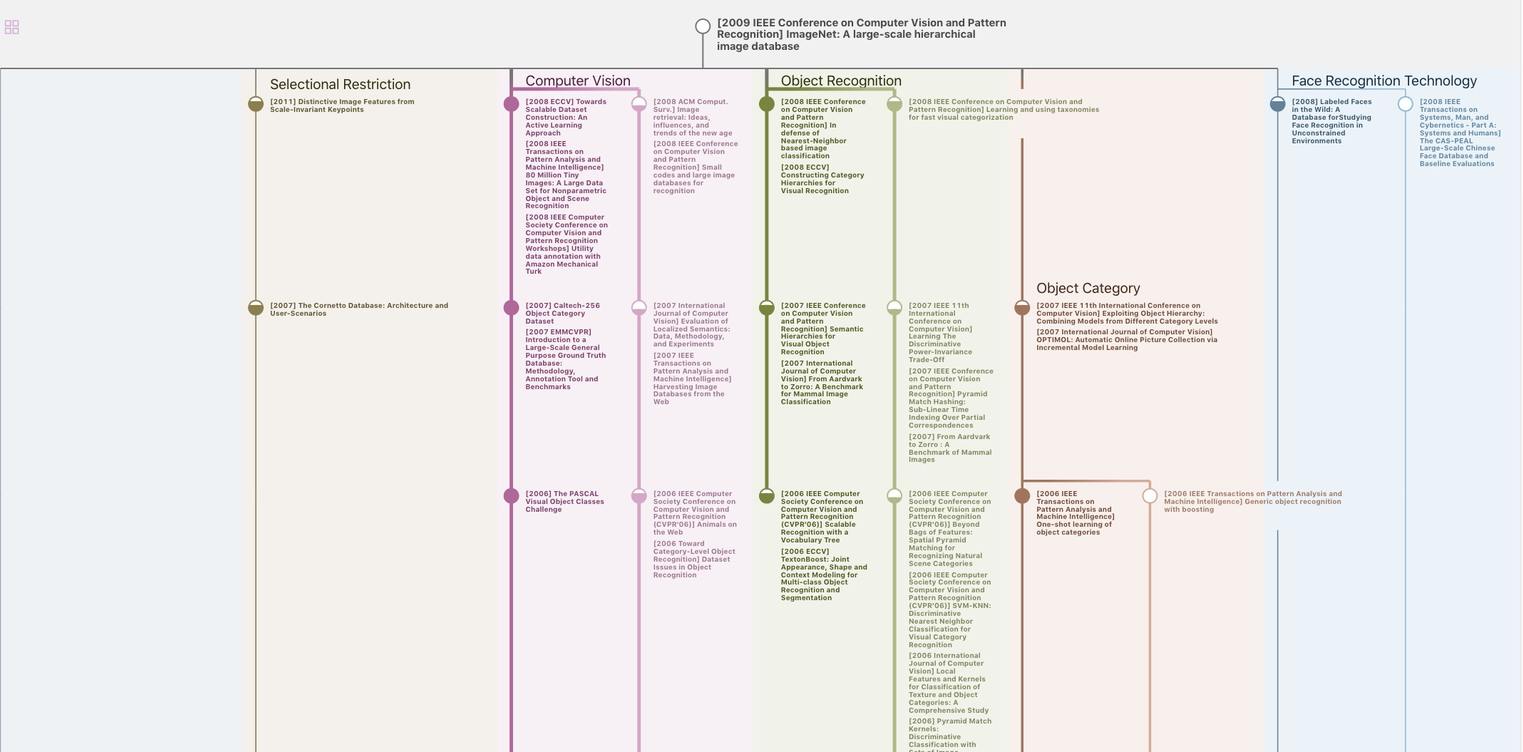
生成溯源树,研究论文发展脉络
Chat Paper
正在生成论文摘要