GNAS: Generalized Neural Attentive Similarity Model for Recommendation.
IEEE International Conference on High Performance Computing and Communications(2021)
摘要
Item-based collaborative filtering captures user preferences by using the historical interactive items of users and generates a personalized recommendation list. Recently, the state-of-the-art collaborative filtering method is neural attentive item similarity model (NAIS). However, the exploration of ensemble models has obtained less investigation. In this work, we present a generalized neural attentive similarity (GNAS) model to perform recommendation based on implicit feedback. The key of our design is to integrate the generalized matrix factorization model and neural attentive item similarity model, which allows the model to have both the memorization ability from matrix factorization model and the generalization ability from neural model. To improve the user interest expression ability of the model, we add an ensemble kernel to the attention mechanism, learning the attention weight of historical items that users have interacted with before. Experimental results show that the recommended accuracy of our model is improved compared to the latest recommended models. In addition, GNAS also has the advantage of less time consumption and interpretability of recommendation.
更多查看译文
关键词
Collaborative filtering,Item-based CF,Ensem-ble model,Attention mechanisms
AI 理解论文
溯源树
样例
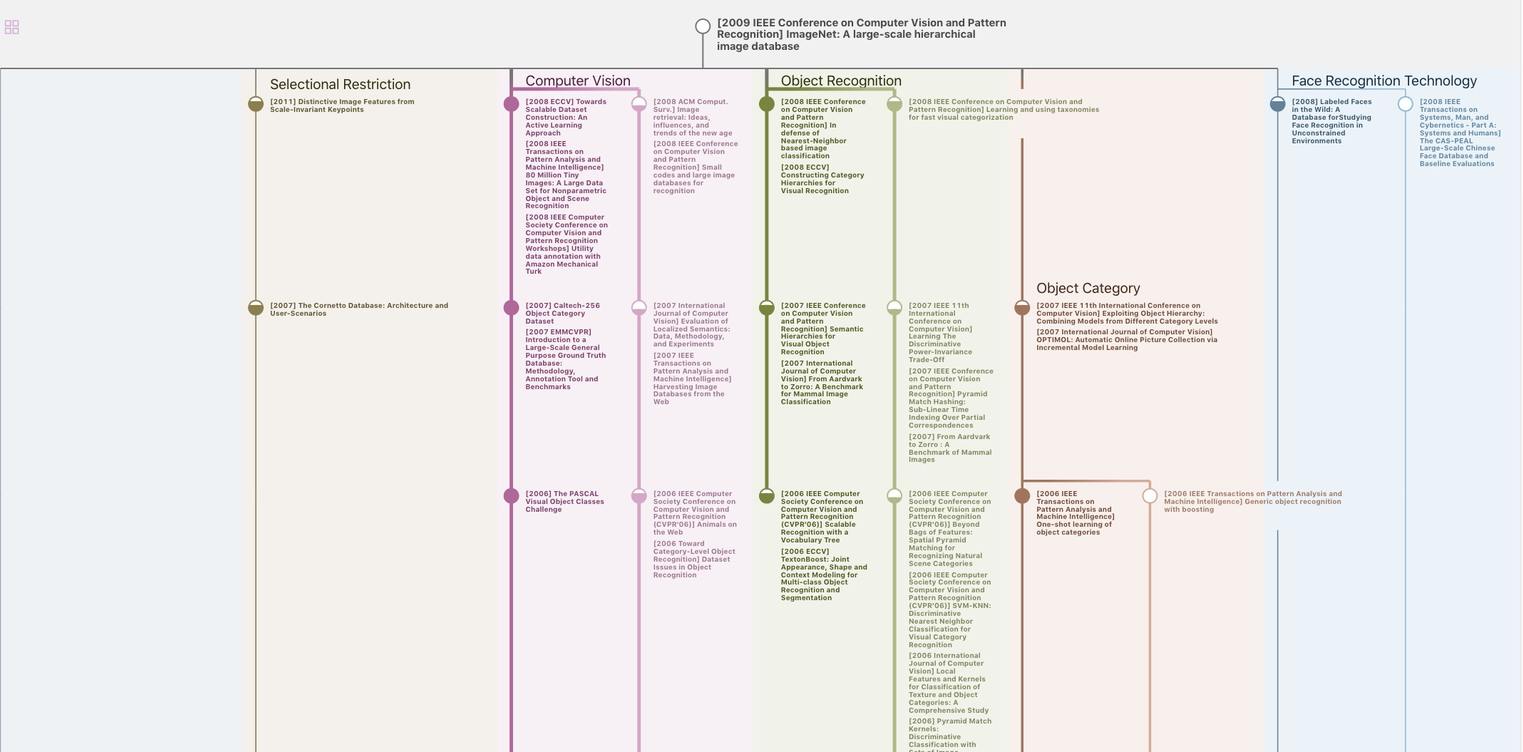
生成溯源树,研究论文发展脉络
Chat Paper
正在生成论文摘要