Organs-At-Risk Segmentation in Medical Imaging Based on the U-Net with Residual and Attention Mechanisms.
IEEE International Conference on High Performance Computing and Communications(2021)
摘要
In this paper, we proposed a new organs-at-risk segmentation method for Medical images of heads and necks based on the U-Net with residuals and attention mechanism. A Project & Excite (PE) block combined with a residual method is proposed as Residual-PE Block in the U-Net encoding stage in order to enhance the feature expression ability. Attention Gates (AGs) are introduced in the decoding stage to increase the relevance of feature weighting in accord with segmentation tasks. The proposed method has been evaluated through a set of real world medical images including 22 organs at risk in the head and neck. Experimental results showed that our proposed method improved the average segmentation accuracy by 3–4% compared with current existing methods. The average Dice Similarity Coefficient (DSC) score on the segmentation of the 22 types of head-and-neck related organs at risk is 79.5% and the average 95% Hausdorff Distance (HD) is 6.99mm. It demonstrates that this approach is promising for clinical doctors to improve their accuracy and efficiency in radiotherapy.
更多查看译文
关键词
Convolutional Neural Networks,Computed Tomography,Image Segmentation
AI 理解论文
溯源树
样例
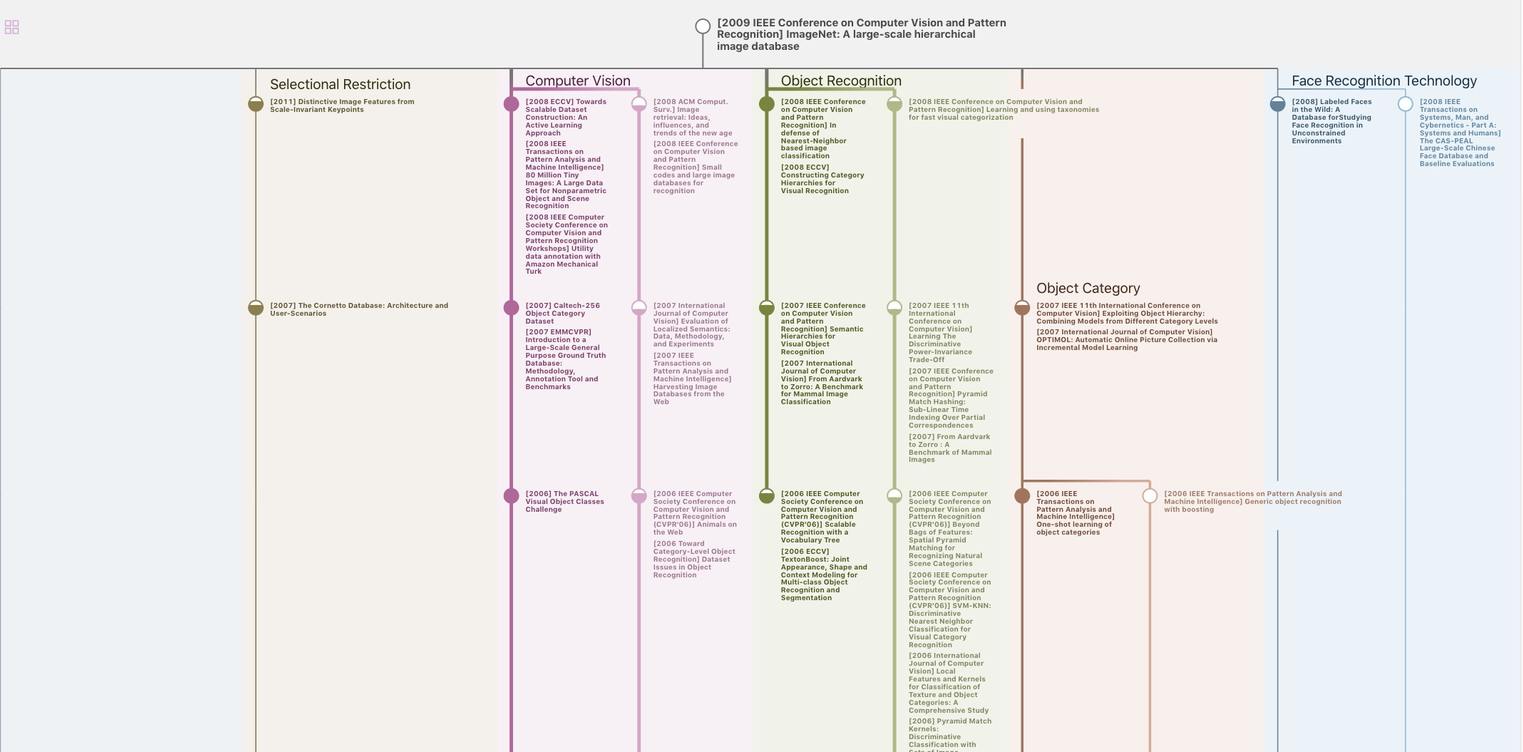
生成溯源树,研究论文发展脉络
Chat Paper
正在生成论文摘要