Behavioural Monitoring and Security Profiling in the Internet of Things (IoT).
IEEE International Conference on High Performance Computing and Communications(2021)
摘要
In recent years the number of IoT devices in businesses has significantly increased, which creates an undeniable challenge for network administrators and industries to identify and monitor the heterogeneous IoT environment. Also, identifying IoT devices helps organizations resolve several security dilemmas, such as determining if connected IoT devices' behaviour is compromised or not. In this work, we propose a distributive profiling mode to distinguish between IoT and non-IoT devices, map devices, identify the network that devices are attached to, and then continuously update the profile. Using a hybrid set of features and 23 new proposed features for identifying IoT devices, our method improves accuracy and shorter training time compared to other existing methods. Our proposed approach experiments with 18 Machine Learning (ML) classifiers on three publicly available datasets, i.e., IoTSentinel, UNSW, LSIF. Our study shows that Random Forest (RF) and Decision Tree (DT) classifiers outperformed the other ML classifiers by achieving an average accuracy, precision, recall, and f1-score above 90% with a shorter training time. This shows that our proposed system is suitable for providing a more generalized solution for profiling IoT devices.
更多查看译文
关键词
Security,IoT Profiling,device identification,machine learning,network-traffic,device behavior
AI 理解论文
溯源树
样例
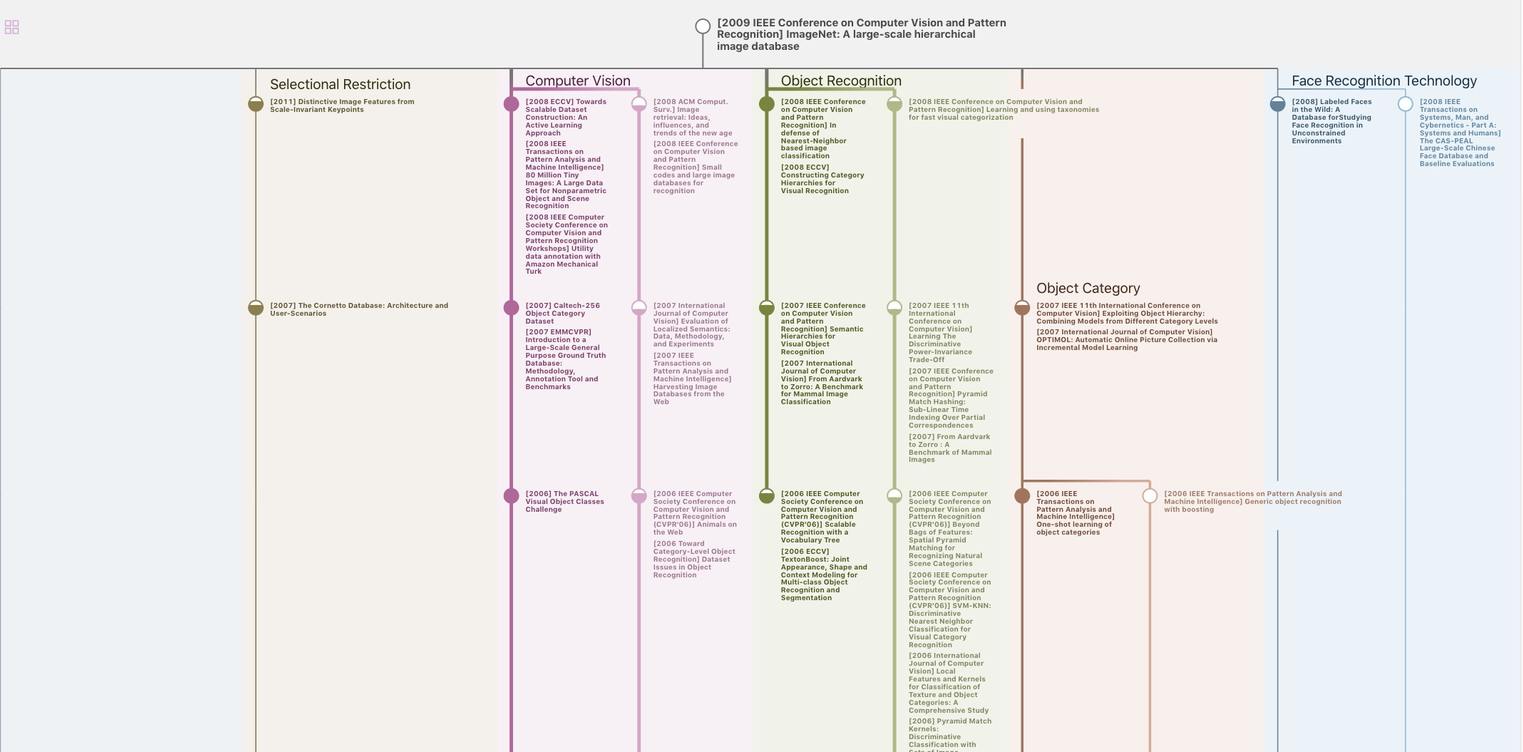
生成溯源树,研究论文发展脉络
Chat Paper
正在生成论文摘要