Detecting Data Leakage in DNS Traffic based on Time Series Anomaly Detection
2021 IEEE 23rd Int Conf on High Performance Computing & Communications; 7th Int Conf on Data Science & Systems; 19th Int Conf on Smart City; 7th Int Conf on Dependability in Sensor, Cloud & Big Data Systems & Application (HPCC/DSS/SmartCity/DependSys)(2021)
摘要
In recent years, privacy data leakage has become a hot topic of security. After malware controls the target client, it needs to bypass the existing security defense to transmit the data to the controller. DNS is a common communication protocol in the network, thus traditional defense methods will not place strict restrictions on DNS traffic. Researchers have found various domain requests for covert data transmission in DNS traffic. In the past ten years, people have only noticed the communication of DNS tunnels, but the new kind of DNS data leakage has a more covert transmission mode through sub-domain name coding and other ways. DNS data leakage exhibits low traffic volume and periodicity, which is totally different from DNS tunnels with bi-directional data exchange and high traffic volume. In this paper, a detection model named as LSTM-AE is proposed. LSTM-AE integrates LSTM-based time-series characterization and unsupervised autoencoder to detect data leakage malware through DNS traffic. Experimental results show the detection performance of LSTM-AE is better than other ML-based methods and several unknown malicious domains related data leakage have been detected with real-world DNS traffic.
更多查看译文
关键词
DNS,Data Leakage,Anomaly Detection,Time Series
AI 理解论文
溯源树
样例
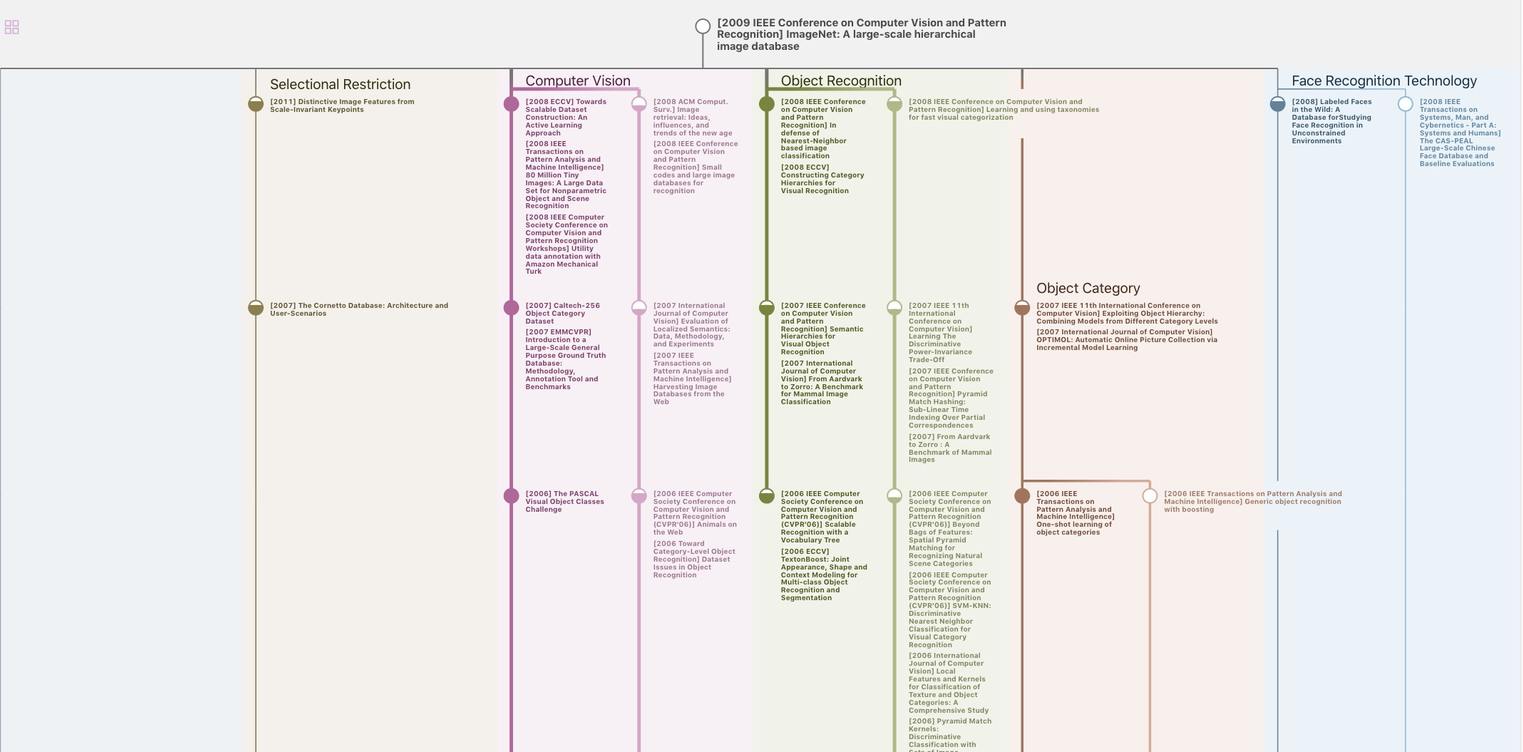
生成溯源树,研究论文发展脉络
Chat Paper
正在生成论文摘要